Customer Company Size
Large Corporate
Region
- Europe
Country
- France
Product
- Camunda
- Camunda Tasklist
- Camunda Operate
Tech Stack
- BPMN workflows
- Process Automation
Implementation Scale
- Enterprise-wide Deployment
Impact Metrics
- Cost Savings
- Productivity Improvements
Technology Category
- Application Infrastructure & Middleware - API Integration & Management
Applicable Functions
- Procurement
Use Cases
- Automated Disease Diagnosis
Services
- System Integration
About The Customer
Sogema Technologies is automating the time-intensive process of land title and deed registrations, providing a flexible solution powered by Camunda to its federal, regional and municipal government clients. With Camunda, Sogema streamlines the orchestration of land registrations from initial title search through registration, fee assessment, and taxation. Registrations are linked to both cadastral and historic title data and shared with national databases, with BPMN workflows modeled, run, and analyzed with Camunda process automation solutions.
The Challenge
Automating land titling and taxation requires developing workflows that often incorporate more than a century’s worth of historical land and title data, mortgage information, cadastral maps, and more. In some cases, new titles may bring previously unregistered land onto tax rolls for the first time, creating new records adding tax revenue for a municipality. Land titling offices also have to keep up with an ever-changing regulatory environment and tax structure. Automated workflows for land titling and deed registration aren’t static, and land administrators need flexibility to customize titling and deed processes regularly and visibility to see that land transfers are processed and recorded efficiently.
The Solution
Sogema’s eLAND solution orchestrates land and property registrations, starting with the notary who initiates a transaction, through historical title and lien searches to registration, fee assessment, and taxation. Land management workflows in eLAND are modeled, executed and monitored in Camunda. The process is automated to populate existing data within eLAND automatically, cutting manual tasks related to registration by as much as two-thirds. Tasks then requiring manual support are automatically assigned through Camunda Tasklist, while Camunda Operate provides visibility to users about outstanding tasks and a transactional dashboard. Heatmaps and dashboards within Operate allow land management officials to monitor workflows and task distribution, identifying bottlenecks in the process and ensuring registrations are processed in a timely manner while also ensuring registry data is standards compliant. Workflows support document management, payments, electronic signature recording, and more. Camunda’s process automation easily integrates with existing database and document management solutions as well as revenue and payment processing applications. Registrations are linked with historical title and property data as well as cadastral records.
Operational Impact
Quantitative Benefit
Case Study missing?
Start adding your own!
Register with your work email and create a new case study profile for your business.
Related Case Studies.
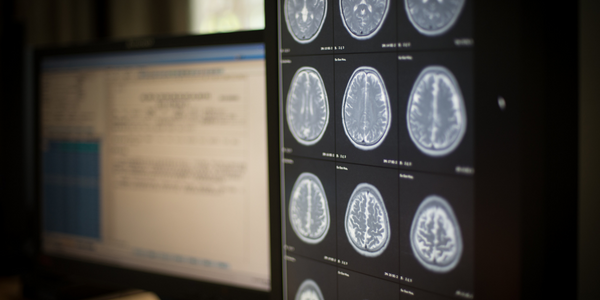
Case Study
Artificial Intelligence and the implications on Medical Imaging
There are several factors simultaneously driving integration of AI in radiology. Firstly, in many countries around the world there is a discrepancy between the number of doctors trained in radiology and the rising demand for diagnostic imaging. This leads to greater demands for work efficiency and productivity. For example, the number of radiology specialists (consultant work- force) in England went up 5% between 2012 and 2015, while in the same period the number of CT and MR scans increased by 29 and 26 percentage points respectively. In Scotland, the gap widened even further (The Royal College of Radiologists 2016). Today, the average radiologist is interpreting an image every three to four seconds, eight hours a day (Choi et al. 2016).Secondly, the image resolution of today’s scanners is continuously improving – resulting in an ever greater volume of data. Indeed, the estimated overall medical data volume doubles every three years, making it harder and harder for radiologists to make good use of the available information without extra help from computerized digital processing. It is desirable, both in radiological research and in clinical diagnostics, to be able to quantitatively analyze this largely unexploited wealth of data and, for example, utilize new measurable imaging biomarkers to assess disease progression and prognosis (O’Connor et al. 2017). Experts see considerable future potential in the transformation of radiology from a discipline of qualitative interpretation to one of quantita- tive analysis, which derives clinically relevant information from extensive data sets (“radiomics”). “Images are more than pictures, they are data,” American radiologist Robert Gillies and his colleagues write (Gillies et al. 2016). Of course, this direction for radiology will require powerful, automated procedures, some of which at least will come under the field of artificial intelligence.
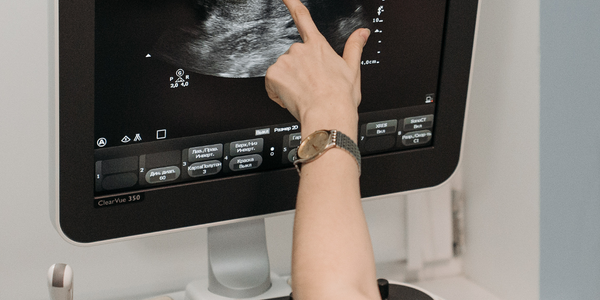
Case Study
Improving Diagnosis Accuracy and Saving Lives
Dr. Partho Sengupta needed a way to accurately identify disease patterns resulting from echocardiograms in order to improve diagnostics and save more lives. Specifically, he wanted to distinguish between two disparate diseases: cardiomyopathy, which directly impacts the heart muscle and often leads to heart failure, and pericarditis, which acts as if the heart is involved but doesn’t actually affect the heart. While both diseases present with similar heart conditions, the treatments are vastly different. For pericarditis, the treatment may include medication and, rarely, surgery. However, if the diagnosis is cardiomyopathy the patient undergoes medical management (i.e. a pacemaker) or in extreme cases, a heart transplant. Misdiagnosis of these disease conditions can put the patient’s life at risk and be very expensive for the hospital. Dr. Sengupta, therefore, looked to Saffron’s Natural Intelligence Platform to help his team increase the diagnosis accuracy of these medical conditions.
Case Study
Population Health Management Platform Anchors Arch Health’s Approach to Lowering Hypertension
Arch Health Partners, a multi-specialty group with over 90 providers at 13 locations in San Diego County, was facing a challenge with its hypertensive patient population. The group's hypertension statistics were below their expectations, and they were struggling to improve the health of these patients. The first step in driving better outcomes was identifying at-risk patients from within a large and growing patient population, which proved to be a more difficult challenge than originally expected. As the organization continued to grow, managing clinical data and improving quality scores became increasingly difficult due to manual processes. The organization realized that to increase the quality and frequency of patient engagement, they would need to automate some of their processes.
Case Study
HeartFlow's Transformation of Heart Disease Diagnosis and Treatment with AWS
HeartFlow, a medical technology company, is revolutionizing the diagnosis and treatment of heart disease with its non-invasive HeartFlow FFRct Analysis. This technology uses deep learning to create a personalized 3D model of the heart, allowing clinicians to better evaluate the impact of a blockage on blood flow and determine the best treatment. However, the company faced a significant challenge. Cardiovascular disease, the world’s leading cause of death, claims more than 17 million lives each year. The most common type, coronary artery disease (CAD), reduces blood flow to the heart, causing chest pain, heart attack, and death. Clinicians need to know if and where there is a blockage, and how it is affecting blood flow. This information is crucial in choosing the best treatment pathway for the patient, such as medical management, stenting or bypass surgery. However, the diagnostic coronary angiogram often used to detect CAD is invasive, expensive, and potentially risky. More than half of patients who undergo the test have no significant blockages, and the procedure can be associated with serious complications.
Case Study
Adaptive Biotechnologies Leverages Azure for Immune Medicine Platform
Adaptive Biotechnologies, a commercial-stage biotechnology company, has been working on harnessing the biology of the adaptive immune system to transform disease diagnosis and treatment. The company has built a proprietary immune medicine platform that decodes the genetic language of the adaptive immune system. However, the challenge was to synthesize this vast system of biology and tap into the full value of the massive clinical immunomics database generated. The company needed high-scale compute resources and machine learning capabilities to unlock the full potential of the research data. The immune system's complexity and the sheer volume of data generated by Adaptive’s dynamic clinical immunomics database, which includes more than 47 billion immune receptors, posed significant challenges.
Case Study
Stanford Medicine Uses Snorkel to Revolutionize Medical Imaging Data Labeling
Labeling training data for triaging models in medical imaging is a time-consuming process, often requiring person-months to person-years of radiologist time. This manual labeling is not only labor-intensive but also prone to human error, which can affect the accuracy and reliability of the models. The challenge was to find a more efficient and accurate method to label large datasets of medical images, which are crucial for developing and training machine learning models for disease diagnosis and patient monitoring.