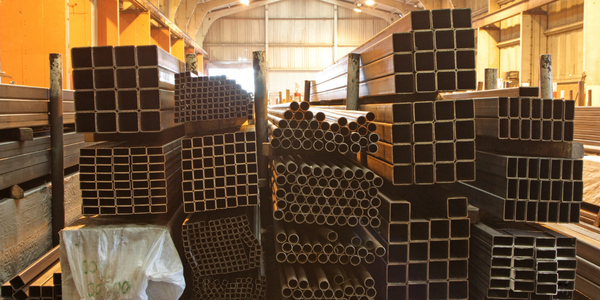
Technology Category
- Analytics & Modeling - Machine Learning
- Functional Applications - Remote Monitoring & Control Systems
- Infrastructure as a Service (IaaS)
Applicable Industries
- Metals
Applicable Functions
- Maintenance
Use Cases
- Machine Condition Monitoring
The Customer
China Steel Corporation
About The Customer
China Steel Corporation (CSC) is the largest integrated steel maker in Taiwan.
The Challenge
China Steel Corporation (CSC) is the largest integrated steel maker in Taiwan. Our goal is to enhance facility maintenance and management efficiency. First and foremost, to achieve this goal we know we need to understand the tradeoffs between the set-up costs of machine condition monitoring (MCM) devices and the losses we experience due to unexpected faults. We also need to consider the critical pieces of equipment we own and the degree of difficulty involved in maintaining them. Effective MCM can help maintenance managers understand the condition of critical equipment in real time, which is a competitive advantage for steel manufacturers.
Our first self-developed facility online monitoring and diagnosis system (FOMOS) was installed on the CSC production line in 1998. The system performed well for monitoring certain issues such as mill vibrations, but after a few years the system became less reliable. We found that the hardware and software had limited functionality. We knew we could build a smarter, more connected system that could use the latest advances in processing power, wireless networks, and software connectivity. We realized that it was fairly easy to develop an MCM system, but it was difficult to address specific challenges we faced.
Our biggest challenge to address was how to detect equipment abnormalities at an early stage without creating a large number of false alarms. Since vibration is a relative condition indicator and strongly affected by system or structural dynamic rigidity and transmissibility, in real situations, it is common to find that two pieces of the same equipment at similar locations and operating conditions exhibit different vibration levels after several years of operation. Vibration signals can be a key indicator for information such as wear, imbalance, misalignment, impact load, and bearing fault by using different signal processing and algorithms.
A conventional monitoring and diagnosis system uses the same or very few indicators and criteria to monitor and diagnose various machine conditions regardless of the nature of the operation of the machine. Therefore, these systems are prone to false alarms or missing alarms. To make it worse, with diverse monitored equipment, a complicated operation regime, and widespread applications in numerous process lines in an integrated steel factory, it is impractical to achieve our goals with only a few diagnosis experts. Thus, we discovered the best way to improve the effectiveness and efficiency of our MCM system was to introduce some artificial intelligence methods.
The Solution
Developing a smart, problem-oriented analysis system that uses the Internet of Things (IoT) to remotely monitor the facility in real time, quickly convert large amounts of data to facility management, and immediately detect facility abnormities. The system must also improve overall facility efficiency, save energy, reduce carbon emissions, and enhance work place safety.
Our new online MCM system, FOMOS-AI, is a patented self-learning system that is based on equipment behavior instead of conventional methodology. To extract useful condition indicators from vibration signals, we categorized the operation context of the monitored equipment into four different patterns, including constant speed and stable load, constant speed and variable load, variable speed and load, and reciprocating.
To obtain better self-diagnosis accuracy, each module integrates data by acquiring several interactive condition signals simultaneously. Moreover, if a facility experiences a specific failure, the analysis module can increase system intelligence and failure identification capabilities. Therefore, it can take large amounts of data and automatically convert it to actionable information for the condition monitoring and prognostic technology.
We designed both the hardware and the software architecture of FOMOS-AI with the highest flexibility in mind so various fault diagnosis and detection algorithms can be implemented. The system software programmed with LabVIEW consists of a diagnostic center, mail setting, and baseline setting
Our new online MCM system, FOMOS-AI, is a patented self-learning system that is based on equipment behavior instead of conventional methodology. To extract useful condition indicators from vibration signals, we categorized the operation context of the monitored equipment into four different patterns, including constant speed and stable load, constant speed and variable load, variable speed and load, and reciprocating.
To obtain better self-diagnosis accuracy, each module integrates data by acquiring several interactive condition signals simultaneously. Moreover, if a facility experiences a specific failure, the analysis module can increase system intelligence and failure identification capabilities. Therefore, it can take large amounts of data and automatically convert it to actionable information for the condition monitoring and prognostic technology.
We designed both the hardware and the software architecture of FOMOS-AI with the highest flexibility in mind so various fault diagnosis and detection algorithms can be implemented. The system software programmed with LabVIEW consists of a diagnostic center, mail setting, and baseline setting
Case Study missing?
Start adding your own!
Register with your work email and create a new case study profile for your business.
Related Case Studies.
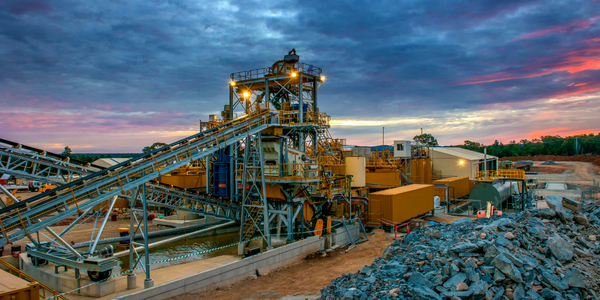
Case Study
Goldcorp: Internet of Things Enables the Mine of the Future
Goldcorp is committed to responsible mining practices and maintaining maximum safety for its workers. At the same time, the firm is constantly exploring ways to improve the efficiency of its operations, extend the life of its assets, and control costs. Goldcorp needed technology that can maximize production efficiency by tracking all mining operations, keep employees safe with remote operations and monitoring of hazardous work areas and control production costs through better asset and site management.
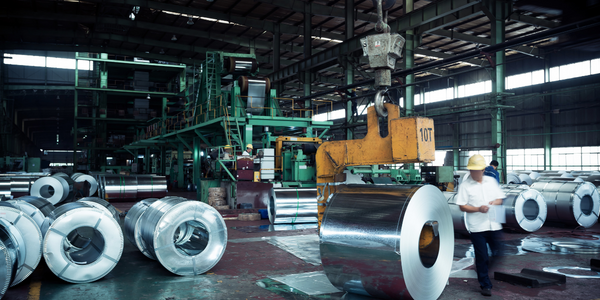
Case Study
KSP Steel Decentralized Control Room
While on-site in Pavlodar, Kazakhstan, the DAQRI team of Business Development and Solutions Architecture personnel worked closely with KSP Steel’s production leadership to understand the steel production process, operational challenges, and worker pain points.
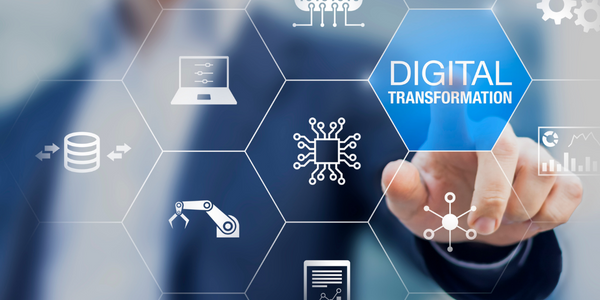
Case Study
Bluescope Steel on Path to Digitally Transform Operations and IT
Increasing competition and fluctuations in the construction market prompted BlueScope Steel to look toward digital transformation of its four businesses, including modern core applications and IT infrastructure. BlueScope needed to modernize its infrastructure and adopt new technologies to improve operations and supply chain efficiency while maintaining and updating an aging application portfolio.
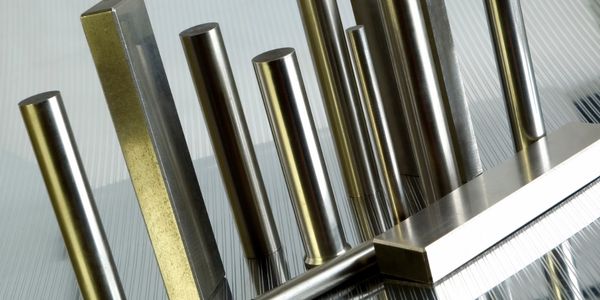
Case Study
RobotStudio Case Study: Benteler Automobiltechnik
Benteler has a small pipe business area for which they produce fuel lines and coolant lines made of aluminum for Porsche and other car manufacturers. One of the problems in production was that when Benteler added new products, production had too much downtime.
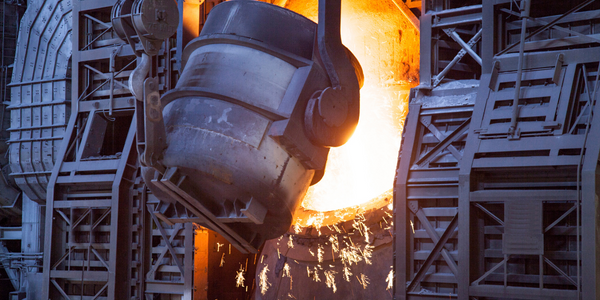
Case Study
Continuous Casting Machines in a Steel Factory
With a very broad range of applications, steel is an important material and has been developed into the most extensive alloy in the engineering world. Since delivering high quality is absolutely crucial for steel plants, ensuring maximum productivity and the best quality production are the keys to competitiveness in the steel industry. Additionally, working conditions in steel factories are not suitable for workers to stay in for long periods of time, so manufactures usually adopt various machines to complete the steel production processes. However, the precision of these machines is often overestimated and the lack of flexibility also makes supervisors unable to adjust operating procedures. A renowned steel factory in Asia planned to improve its Distributed Control System (DCS) of furnaces as well as addressing the problem of insufficient accuracy. However, most well-known international equipment suppliers can not provide a satisfactory solution and local maintenance because the project needed new technologies to more accurately control equipment operations. By implementing Advantech’s automated monitoring and control solution, steel factories can not only improve the manufacturing processes but can also allow users to add additional functions to the existing system so as to make sure the operation runs at high efficiency.
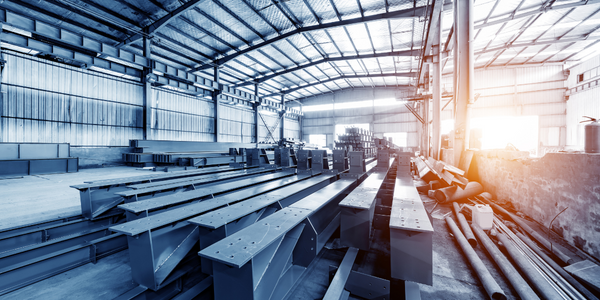
Case Study
Automated Predicitive Analytics For Steel/Metals Industry
Asset to be monitored: Wire Compactor that produces Steel RebarCustomer Faced The Following Challenges:Dependent upon machine uptime.Pressure cylinders within the compactor fail to control compression and speed causing problems in binding the coil.Equipment failure occurs in the final stage of production causing the entire line to stop, can you say bottleneck?Critical asset unequipped with sensors to produce data.