Technology Category
- Analytics & Modeling - Machine Learning
- Sensors - Liquid Detection Sensors
Applicable Industries
- Education
- Mining
Applicable Functions
- Maintenance
- Product Research & Development
Use Cases
- Time Sensitive Networking
- Virtual Training
Services
- Data Science Services
- Training
About The Customer
Schlumberger is the world’s leading provider of technology and services for the energy industry, operating in over 120 countries. The company provides well maintenance and analytics services to the world’s biggest oil companies. Schlumberger believes that large-scale data analysis and artificial intelligence/machine learning will help them remain a leader in the market. The Software Technology Innovation Center (STIC), within the 85,000-person industry leader, is dedicated to using new AI/ML applications to support the company’s mission to improve the performance and sustainability for the global energy industry. They aim to streamline information extraction from critical field data that underpins Schlumberger’s efforts to do a large-scale analysis of business operations and deliver data-driven insights into their performance.
The Challenge
Schlumberger, a leading provider of technology and services for the energy industry, faced a significant challenge in extracting crucial information from a vast array of daily reports. These reports, ranging from daily drilling reports to well maintenance logs, each had their unique structure and format, making it difficult for Schlumberger’s team to quickly extract the necessary information. The team attempted to automate the information extraction using Named Entity Recognition (NER), but off-the-shelf ML models failed to identify the scientific terms related to the Exploration and Production (E&P) industry. Creating a domain-specific training dataset was time-consuming and not scalable, taking anywhere from 1-3 hours per document. The team needed to identify 18 different industry-specific entities and automatically associate data with these entities. However, the rich information was buried within tabular and raw text in PDFs with varied formatting across reports from different companies. There was also poor collaboration between domain experts and data scientists, with cumbersome file sharing and ad-hoc meetings.
The Solution
Schlumberger turned to Snorkel Flow to build an AI application that could automatically extract key scientific data from geological and field data reports. This solution was developed in just three days and was used to guide recommendations for better well management across multiple clients. The AI application was built using a data-centric artificial intelligence (AI) development lifecycle accelerated by programmatic labeling. After a few rounds of rapid iteration using Snorkel Flow’s model-guided error analysis and programmatic labeling, the team improved their F1 score to 91.4%. The AI application reduced the processing time of reports from 1 to 3 hours per report to just a few seconds. It was able to extract several different entities from unstructured data, including well maintenance activity description (textual), time of activity (numerical), and more. The solution also overcame the challenge of non-standard reporting formats, successfully identifying entities across 15 different document structures.
Operational Impact
Quantitative Benefit
Case Study missing?
Start adding your own!
Register with your work email and create a new case study profile for your business.
Related Case Studies.
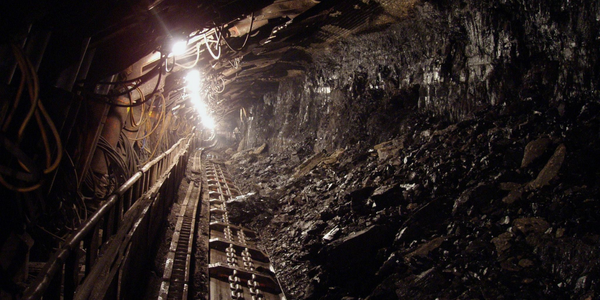
Case Study
Underground Mining Safety
The goal was to produce a safety system to monitor and support underground mining operations; existing systems were either too simple (i.e. phone line) or overly complex and expensive, inhibiting deployment, and providing little-to-no support in event of an accident. Given the dangerous nature of the mining work environment and the strict regulations placed on the industry, the solution would have to comply with Mine Safety and Health Administration (MSHA) regulations. Yet the product needed to allow for simple deployment to truly be a groundbreaking solution - increasing miner safety and changing daily operations for the better.
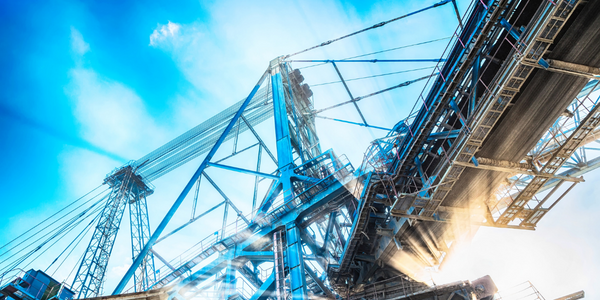
Case Study
Mining Firm Quadruples Production, with Internet of Everything
Dundee Precious Metal’s flagship mine, in Chelopech, Bulgaria, produces a gold, copper, and silver concentrate set a goal to increase production by 30%. Dundee wanted to increase production quality and output without increasing headcount and resources, improve miner safety, and minimize cost.
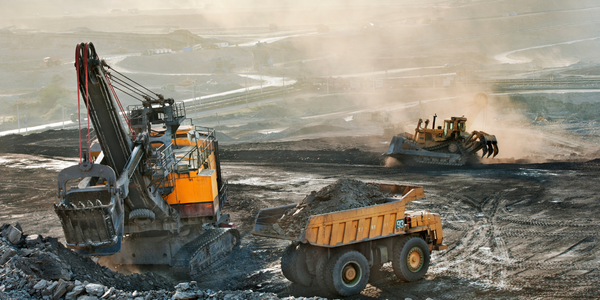
Case Study
Fastenal Builds the Future of Manufacturing with MachineMetrics
Fastenal's objective was to better understand their machine downtime, utilization, quality issues, and to embrace cutting-edge manufacturing technology/process improvement capabilities to bring their team to the next level. However, there was a lack of real-time data, visualization, and actionable insights made this transition impossible.
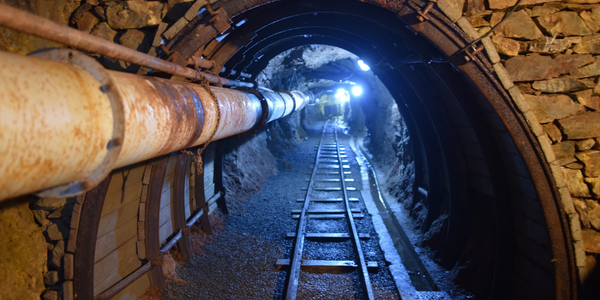
Case Study
Joy Mining Systems
Joy equipment faces many challenges. The first is machine integration and control. The business end of the machine has a rapidly-spinning cylinder with 6-inch diamond-studded cutting teeth. It chews through rock at rates measured in tens of tons per minute. The system grinds through the rock in front, creating a rectangular mine tunnel. Hydraulic lifters support the ceiling as the machine moves forward. Automated drills and screws drive 3-ft long screws into the ceiling to stabilize it. The rock and coal fall into a set of gathering "fingers" below the cutting cylinder. These fingers scoop up the rock and coal and deposit it onto a conveyor belt. The conveyor passes under the machine and out the back. A train of conveyor belt cars, up to a mile long, follows the cutter into the mine. The rock shoots along this train at over 400 feet per minute until it empties into rail cars at the end. Current systems place an operator cage next to the cutter. Choking dust (potentially explosive), the risk of collapse and the proximity of metal and rock mayhem make the operator cage a hazardous location.
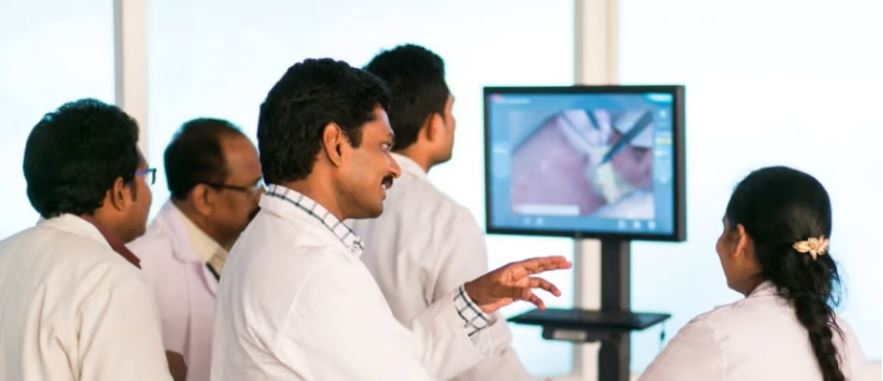
Case Study
Revolutionizing Medical Training in India: GSL Smart Lab and the LAP Mentor
The GSL SMART Lab, a collective effort of the GSL College of Medicine and the GSL College of Nursing and Health Science, was facing a challenge in providing superior training to healthcare professionals. As clinical medicine was becoming more focused on patient safety and quality of care, the need for medical simulation to bridge the educational gap between the classroom and the clinical environment was becoming increasingly apparent. Dr. Sandeep Ganni, the director of the GSL SMART Lab, envisioned a world-class surgical and medical training center where physicians and healthcare professionals could learn skills through simulation training. He was looking for different simulators for different specialties to provide both basic and advanced simulation training. For laparoscopic surgery, he was interested in a high fidelity simulator that could provide basic surgical and suturing skills training for international accreditation as well as specific hands-on training in complex laparoscopic procedures for practicing physicians in India.
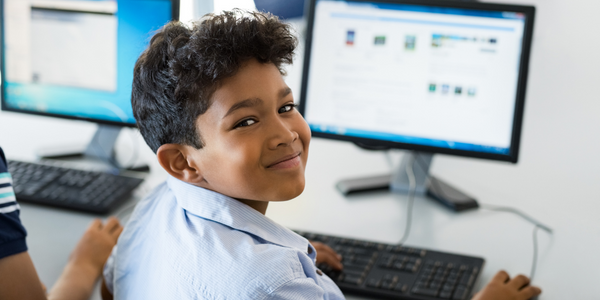
Case Study
IoT platform Enables Safety Solutions for U.S. School Districts
Designed to alert drivers when schoolchildren are present, especially in low-visibility conditions, school-zone flasher signals are typically updated manually at each school. The switching is based on the school calendar and manually changed when an unexpected early dismissal occurs, as in the case of a weather-event altering the normal schedule. The process to reprogram the flashers requires a significant effort by school district personnel to implement due to the large number of warning flashers installed across an entire school district.