Customer Company Size
Large Corporate
Region
- Europe
Country
- Germany
Product
- Ops-IQ
- LiveOps
Tech Stack
- Big Data Analytics
- Machine Learning
Implementation Scale
- Enterprise-wide Deployment
Impact Metrics
- Cost Savings
- Customer Satisfaction
Technology Category
- Analytics & Modeling - Big Data Analytics
- Analytics & Modeling - Machine Learning
Applicable Industries
- Telecommunications
Applicable Functions
- Business Operation
Use Cases
- Predictive Maintenance
Services
- Data Science Services
About The Customer
The customer is a European Multiple-System Operator (MSO) that provides integrated triple-play services to several million subscribers of cable TV, broadband internet, and telephony offerings. The MSO prides itself on delivering world-class customer service and wanted to accelerate network resolution times to continue delivering the strong customer experiences its subscriber base had come to value.
The Challenge
A European Multiple-System Operator (MSO) was struggling to rapidly distinguish between issues caused by customer premise devices and headend equipment. This delayed the MSO’s ability to find the root cause of problems and subsequently resolve the issues. With the cost of a truck roll in Germany running about 60 to 70 euros and the handling of incoming customer service calls running about 5 to 10 euros each, the provider hoped to reduce customer service costs and improve customer satisfaction at the same time.
The Solution
The MSO implemented Ops-IQ after a five-month proof-of-value (POV) test with actual anonymized data. The solution revealed hidden insights and root issues and removed the barriers between data silos. By correlating massive amounts of disparate data and running advanced analytics on it in real-time, the MSO’s NetOps and CareOps teams learned that they could identify and troubleshoot issues faster— sometimes even before they occurred. The result was a domino effect of reduced customer calls, trouble tickets, and truck rolls; enough to save the company “seven digits” annually.
Operational Impact
Quantitative Benefit
Case Study missing?
Start adding your own!
Register with your work email and create a new case study profile for your business.
Related Case Studies.
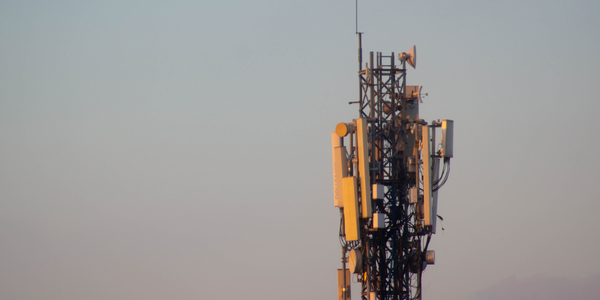
Case Study
Vodafone Hosted On AWS
Vodafone found that traffic for the applications peak during the four-month period when the international cricket season is at its height in Australia. During the 2011/2012 cricket season, 700,000 consumers downloaded the Cricket Live Australia application. Vodafone needed to be able to meet customer demand, but didn’t want to invest in additional resources that would be underutilized during cricket’s off-season.
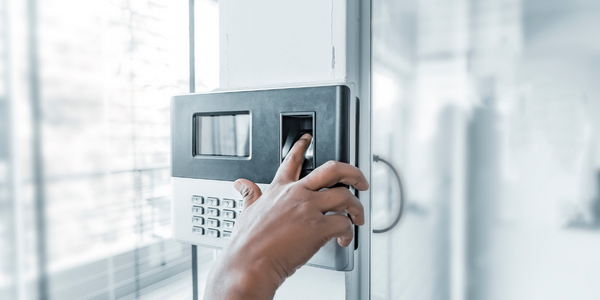
Case Study
SKT, Construction of Smart Office Environment
SK T-Tower is the headquarters of SK Telecom. Inside the building, different types of mobile devices, such as laptops, smartphones and tablets, are in use, and with the increase in WLAN traffic and the use of quality multimedia data, the volume of wireless data sees an explosive growth. Users want limitless Internet access in various places in addition to designated areas.