Customer Company Size
Large Corporate
Country
- Worldwide
Product
- Google Analytics
Tech Stack
- Google Analytics
Implementation Scale
- Enterprise-wide Deployment
Impact Metrics
- Brand Awareness
- Customer Satisfaction
Technology Category
- Analytics & Modeling - Real Time Analytics
Applicable Functions
- Sales & Marketing
Use Cases
- Demand Planning & Forecasting
Services
- Data Science Services
About The Customer
Fairmont Hotels & Resorts is a luxury hotel company with more than 60 distinctive hotels and resorts worldwide. The company uses social media channels such as Twitter to increase awareness of offers and drive traffic to sites within their portfolio. They faced a challenge in accurately identifying and tracking website traffic generated by their tweets, as many Twitter users don’t use the web interface, instead employing one of the many desktop clients or mobile apps available. In addition, a link that’s been posted on Twitter might be forwarded via email or SMS, resulting in traffic that should be attributed to Twitter activities being reported as direct or other referral traffic.
The Challenge
Fairmont Hotels & Resorts, a luxury hotel company with more than 60 distinctive hotels and resorts worldwide, was looking to optimize its social media marketing efforts. The company uses Twitter to increase awareness of offers and drive traffic to sites within their portfolio. However, they faced a challenge in accurately identifying and tracking website traffic generated by their tweets. This was due to the fact that a significant proportion of Twitter traffic doesn’t originate from twitter.com; many Twitter users don’t use the web interface, instead employing one of the many desktop clients or mobile apps available. In addition, a link that’s been posted on Twitter might be forwarded via email or SMS. In all these cases, any traffic which in theory should be attributed to Twitter activities would be reported as direct or other referral traffic.
The Solution
Fairmont Hotels & Resorts solved this problem by using Google Analytics’ campaign tracking variables. These variables allowed them to tag their links so that Google Analytics could recognize and measure non-AdWords campaigns that brought visitors to their site. When campaign tracking variables were applied to any link in Fairmont’s tweets, the traffic resulting from those tweets was correctly attributed to the respective tweet regardless of where the visitor found and clicked on the link. To keep the links short and not use up valuable characters in a 140-character message, Fairmont’s team used free URL shortening services such as goo.gl. Google Analytics allowed Fairmont to compare the quality and growth rate of the Twitter traffic generated by their own activities compared to the “organic” traffic received from Twitter. Since each Twitter post was uniquely tagged, they could review how each one performed, first by selecting the chosen traffic channel and then by selecting the specific campaign.
Operational Impact
Quantitative Benefit
Case Study missing?
Start adding your own!
Register with your work email and create a new case study profile for your business.
Related Case Studies.
Case Study
Pepsico's Transformation to Smarter Sales Forecasting with Designer Cloud
PepsiCo, a global consumer packaged goods company, faced a significant challenge in calibrating sales forecasting to supply the right product quantities to its retailers. The sales forecast incorporated a variety of data, including warehouse data, store stock data, and promotional forecast data, all of which were provided by retailers in different file formats and delivered using various methods. The primary challenge was the speed of preparing a sales forecast. With the existing Microsoft Access and Excel-based processes, the time required to prepare this data was so extensive that analysts could only leverage it once a month or not at all. This inefficiency risked under or oversupplying retailers, potentially impacting PepsiCo's business operations and customer relationships.
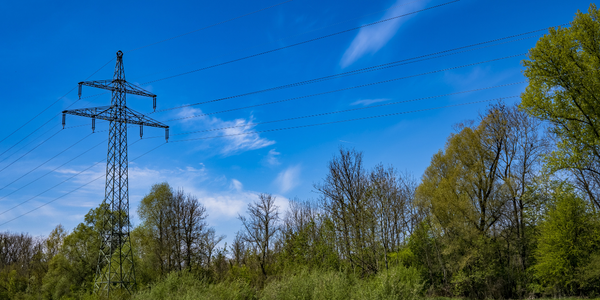
Case Study
Gexa Energy and AutoGrid's Innovative Demand Response Programs in ERCOT
Gexa Energy, a leading retail electricity provider in Texas, was seeking to introduce new demand response programs for its commercial and industrial customers in the Electric Reliability Council of Texas (ERCOT) market. The challenge was to provide a platform that would allow these customers to lower their energy bills by adjusting their energy consumption during peak energy demand or high wholesale electricity prices. The solution needed to be intelligent, scalable, and offer both manual and automated options for adjusting energy consumption. The demand response programs needed to include Emergency Response Service (ERS), Real-Time Price Response (RTPR), and 4 Coincident Peak (4CP).
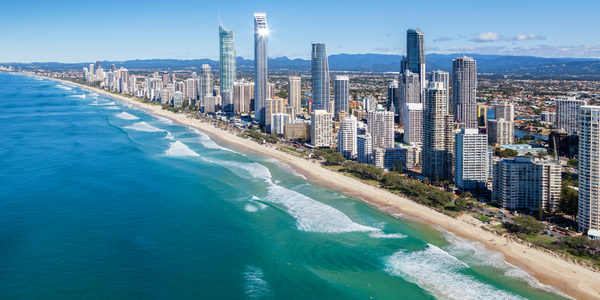
Case Study
ZettaNet's Agile Juniper Network Meets Booming Digital Demand in Australia
ZettaNet, a privately-held company based in Perth, Australia, was facing a significant challenge due to the exponential demand for enterprise network, data center, and cloud services in the region. The company's business growth necessitated an upgrade of their core network to meet the increasing bandwidth requirements of their customers. The customers, which primarily include managed service providers, were demanding 1 Gbps connectivity between locations. These service providers then deliver network, data center, cloud, and voice services to a diverse range of customers including local businesses, schools, hospitals, residential communities, and government offices in Western and Southern Australia. The challenge for ZettaNet was to meet this high-capacity network services demand while maintaining profitability.
Case Study
MARS Incorporated: Leading a Global Digital Transformation
MARS Incorporated, a multinational manufacturer, faced significant challenges in digitizing and standardizing processes across its mid-markets globally. The company's reliance on legacy solutions necessitated continuous, time-consuming upgrades and made data compilation and comparison across different regions and business units difficult. Excel spreadsheets were extensively used for forecasting in smaller markets, leading to disconnected processes, siloed working environments, and increased risk of data inconsistencies and inaccuracies in demand forecasting. MARS also struggled with a lack of visibility across its midmarket footprint, scattered critical data across various systems and spreadsheets, a low degree of automation, and insufficient statistical analytics for demand planning. The absence of a standard process for demand planning made it challenging to consolidate KPIs and gain a comprehensive view of demand trends and supply chain performance.
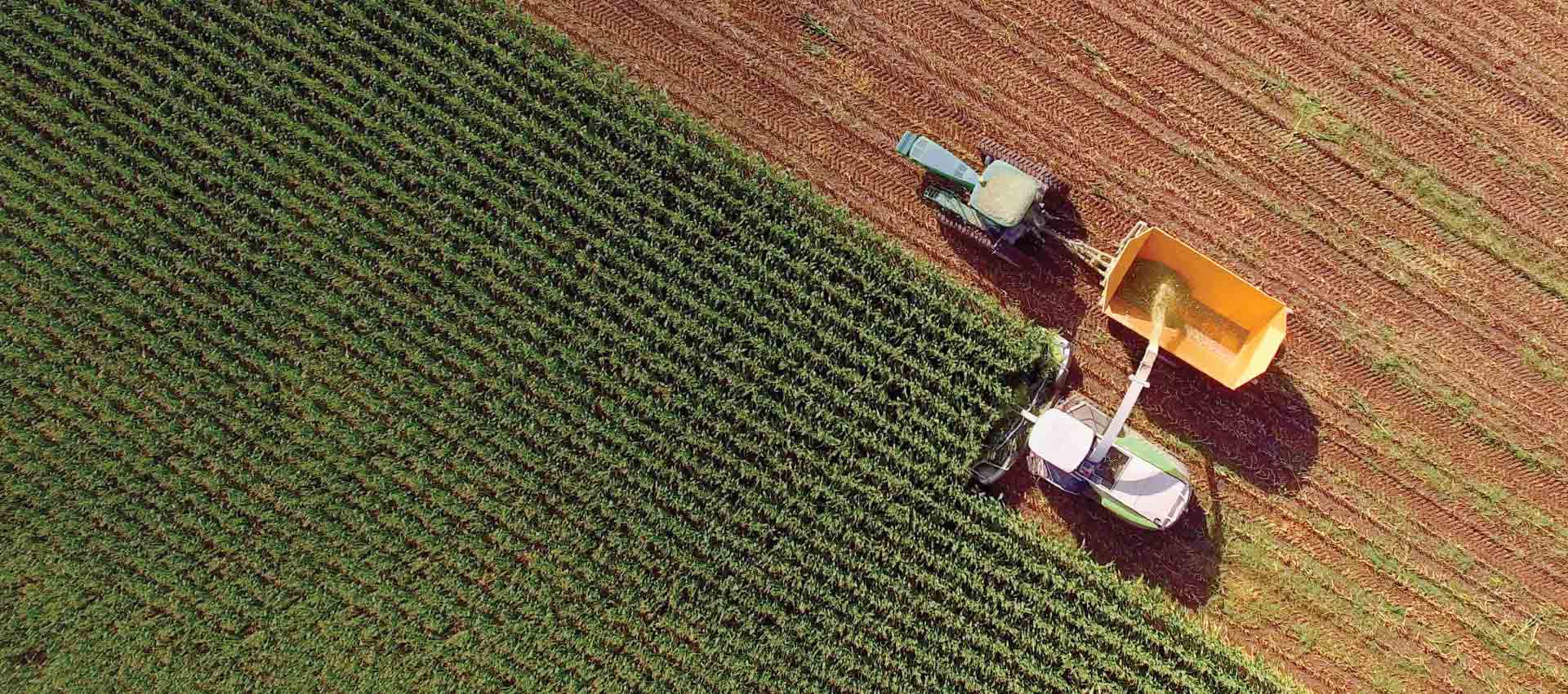
Case Study
Enterprise AI for Demand Forecasting and Production Scheduling in Global Agribusiness
A global agribusiness and food manufacturer, producing over 80 million pounds of food products per year across eight production lines, faced significant challenges in demand forecasting and production scheduling. The company's only customer, a global retailer, exhibited highly variable demand, leading to discrepancies with the manufacturer's weekly demand forecast. Traditional demand forecasting solutions, based on statistical algorithms, were unable to cope with the short lead times and daily sales orders due to the short shelf life of food products. This resulted in unfulfilled customer orders. Additional rule-based solutions procured to improve production scheduling also failed to optimize schedules and significantly improve manufacturing operations.
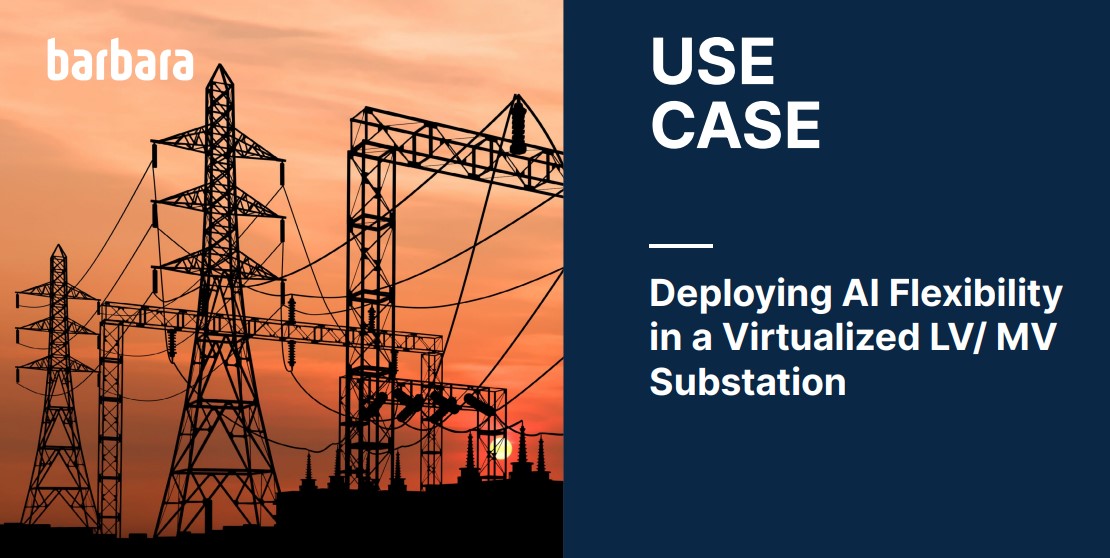
Case Study
Edge AI: Deploying AI Flexibility in a Virtualized LV/ MV Substation
Cuerva a Spanish Grip Operator, was seeking to enhance grid knowledge through the implementation of the AI Energy Forecasting Model to obtain precise forecasts of user demand and energy generation.Cuerva’s grid encompasses over 16,000 diverse supply points, making cloud-based operations intricate and susceptible to issues such as connectivity loss, delays in information transmission, and reliance on centralized infrastructure, which can result in the loss of critical data.To tackle these challenges, the Edge technology has proven to be the sole alternative capable of addressing these issues effectively. It ensures real-time data access and operates in a decentralized manner, minimizing the impact of device failures on the overall functionality of the network.In this successful case, we illustrate how with Barbara DSOs can implement AI directly in substations to accurately predict the demand and production values of consumers linked to the transformation center where an Edge node run by Barbara has been deployed.