Technology Category
- Networks & Connectivity - 5G
- Platform as a Service (PaaS) - Application Development Platforms
Applicable Industries
- E-Commerce
- Retail
Use Cases
- Supply Chain Visibility
- Traffic Monitoring
About The Customer
Future Pay is an app-based wallet offering for online shopping across Future Group stores. The app aims to provide an effortless online shopping experience to more than 20 million customers. Using the Future Pay app, customers can shop at over 1500 Future Group stores including Big Bazaar, fbb, Ezone, HyperCity, Central, Easyday Club, Heritage Fresh, Foodhall, Nilgiris, Brand Factory and more. The app also provides cashless and card-less transactions by linking the loyalty cards (payback and profit club cards) to their app, eliminating the need for customers to carry these cards.
The Challenge
Future Pay, a wallet app by Future Group, faced a challenge in tracking user interactions with promotional banners on their home screen. These banners, which redirected users to selected brand pages, were a key part of their marketing strategy. However, the Future Pay product team was unable to track clicks on these banners or measure conversions from users who landed on these brand pages. This lack of visibility made it difficult to assess the effectiveness of their marketing efforts and optimize their engagement strategy. The team wanted to understand user behavior and drop-off on these banners, hoping that this would help them offer more personalized engagement and boost traffic and conversions. The criteria for a solution were to help the team understand user behavior and drop-off on these banners.
The Solution
The Future Pay product team decided to use MoEngage, a customer engagement platform, to gain insights into user app activity and execute a comprehensive engagement strategy. The team devised a three-step strategy: understanding user app activity and engagement with discount banners, segmenting users based on these insights, and creating engagement campaign workflows to optimize user communication on various discounts and offers. They analyzed user actions such as in-app searches, clicks on push notifications, and clicks on specific banners to map user journeys. This data helped them understand user preferences and the performance of the banners. The team then segmented users based on their app activity, preference, and demographics, and ran various engagement campaigns tailored to these segments. They used MoEngage’s AI-based recommendation engine Sherpa to optimize the messaging and send time.
Operational Impact
Quantitative Benefit
Case Study missing?
Start adding your own!
Register with your work email and create a new case study profile for your business.
Related Case Studies.
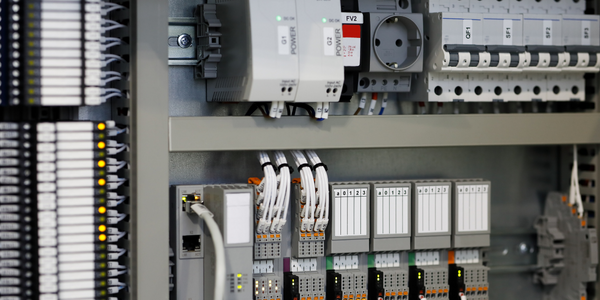
Case Study
Improving Production Line Efficiency with Ethernet Micro RTU Controller
Moxa was asked to provide a connectivity solution for one of the world's leading cosmetics companies. This multinational corporation, with retail presence in 130 countries, 23 global braches, and over 66,000 employees, sought to improve the efficiency of their production process by migrating from manual monitoring to an automatic productivity monitoring system. The production line was being monitored by ABB Real-TPI, a factory information system that offers data collection and analysis to improve plant efficiency. Due to software limitations, the customer needed an OPC server and a corresponding I/O solution to collect data from additional sensor devices for the Real-TPI system. The goal is to enable the factory information system to more thoroughly collect data from every corner of the production line. This will improve its ability to measure Overall Equipment Effectiveness (OEE) and translate into increased production efficiencies. System Requirements • Instant status updates while still consuming minimal bandwidth to relieve strain on limited factory networks • Interoperable with ABB Real-TPI • Small form factor appropriate for deployment where space is scarce • Remote software management and configuration to simplify operations
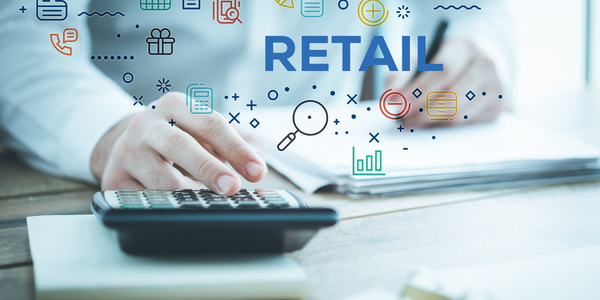
Case Study
Digital Retail Security Solutions
Sennco wanted to help its retail customers increase sales and profits by developing an innovative alarm system as opposed to conventional connected alarms that are permanently tethered to display products. These traditional security systems were cumbersome and intrusive to the customer shopping experience. Additionally, they provided no useful data or analytics.
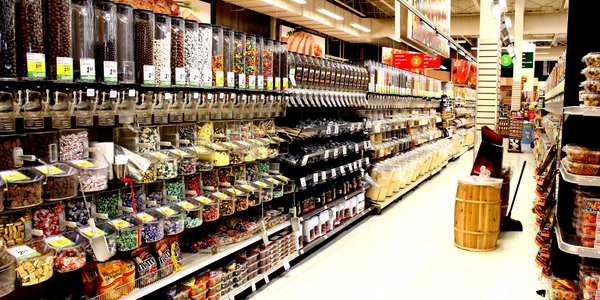
Case Study
How Sirqul’s IoT Platform is Crafting Carrefour’s New In-Store Experiences
Carrefour Taiwan’s goal is to be completely digital by end of 2018. Out-dated manual methods for analysis and assumptions limited Carrefour’s ability to change the customer experience and were void of real-time decision-making capabilities. Rather than relying solely on sales data, assumptions, and disparate systems, Carrefour Taiwan’s CEO led an initiative to find a connected IoT solution that could give the team the ability to make real-time changes and more informed decisions. Prior to implementing, Carrefour struggled to address their conversion rates and did not have the proper insights into the customer decision-making process nor how to make an immediate impact without losing customer confidence.
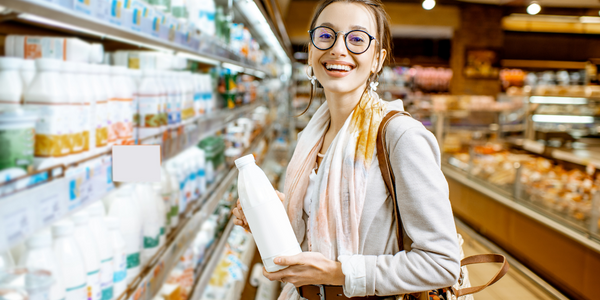
Case Study
Ensures Cold Milk in Your Supermarket
As of 2014, AK-Centralen has over 1,500 Danish supermarkets equipped, and utilizes 16 operators, and is open 24 hours a day, 365 days a year. AK-Centralen needed the ability to monitor the cooling alarms from around the country, 24 hours a day, 365 days a year. Each and every time the door to a milk cooler or a freezer does not close properly, an alarm goes off on a computer screen in a control building in southwestern Odense. This type of alarm will go off approximately 140,000 times per year, equating to roughly 400 alarms in a 24-hour period. Should an alarm go off, then there is only a limited amount of time to act before dairy products or frozen pizza must be disposed of, and this type of waste can quickly start to cost a supermarket a great deal of money.
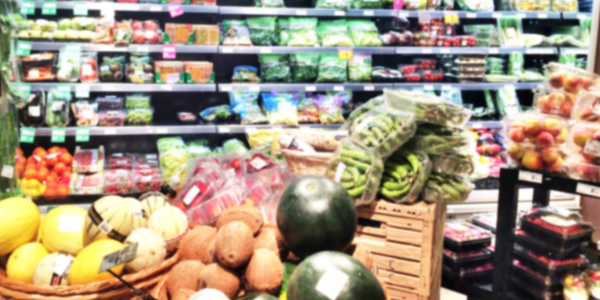
Case Study
Supermarket Energy Savings
The client had previously deployed a one-meter-per-store monitoring program. Given the manner in which energy consumption changes with external temperature, hour of the day, day of week and month of year, a single meter solution lacked the ability to detect the difference between a true problem and a changing store environment. Most importantly, a single meter solution could never identify root cause of energy consumption changes. This approach never reduced the number of truck-rolls or man-hours required to find and resolve issues.