Technology Category
- Analytics & Modeling - Natural Language Processing (NLP)
- Analytics & Modeling - Robotic Process Automation (RPA)
Applicable Industries
- Telecommunications
Use Cases
- Chatbots
- Speech Recognition
Services
- System Integration
- Training
About The Customer
Goodcall is a company that provides businesses with intelligent phone agents. These agents are essentially chatbots that customers can call to request information about the business’s hours, location, and products or services. The chatbots use automatic speech recognition (ASR) to convert speech-to-text and then employ AI analysis to interpret the person’s request and provide the appropriate response. The intent classification models that power Goodcall’s chatbots need to be regularly fine-tuned with real-world production data. Goodcall's goal is to improve the customer experience for their clients by enhancing the performance of their chatbots.
The Challenge
Goodcall, a company providing businesses with intelligent phone agents, faced a significant challenge in managing and annotating the high volume of data generated by their chatbots. The chatbots, which use automatic speech recognition (ASR) to convert speech-to-text and AI analysis to interpret customer requests, required regular fine-tuning with real-world production data. However, the process of labeling this massive amount of data with high-quality annotations was time-consuming and resource-intensive. Furthermore, Goodcall was unable to match the scale of available data due to their in-house data annotation process. This meant that every piece of unlabeled data was a missed opportunity to improve their models. To enhance model performance and customer experience, Goodcall needed a scalable, sustainable approach for labeling large quantities of data.
The Solution
Goodcall partnered with Scale Rapid to acquire high-quality text annotations. Scale Rapid's user-friendly tools allowed Goodcall to send large collections of samples for review and receive text classification labels in return. These labels, which describe the meaning of certain phrases, provided the data that software engineers needed to evaluate and improve their models. Goodcall also used these human-reviewed labels to check against the unlabeled items in their system, thereby expanding the variety of data they could use to improve their models. This process helped Goodcall improve the accuracy of a model’s text-to-speech transcripts and enhance a bot’s ability to correctly understand intent.
Operational Impact
Quantitative Benefit
Case Study missing?
Start adding your own!
Register with your work email and create a new case study profile for your business.
Related Case Studies.
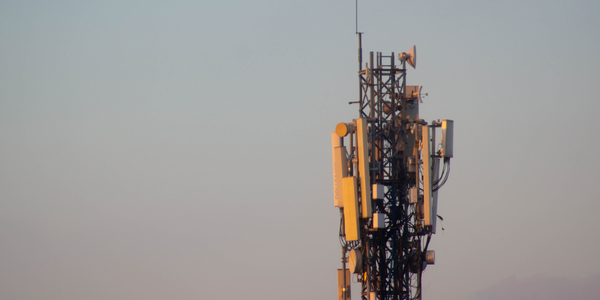
Case Study
Vodafone Hosted On AWS
Vodafone found that traffic for the applications peak during the four-month period when the international cricket season is at its height in Australia. During the 2011/2012 cricket season, 700,000 consumers downloaded the Cricket Live Australia application. Vodafone needed to be able to meet customer demand, but didn’t want to invest in additional resources that would be underutilized during cricket’s off-season.
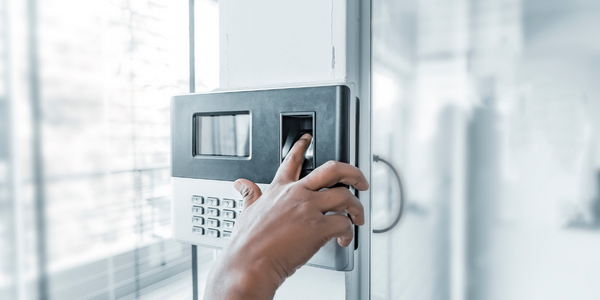
Case Study
SKT, Construction of Smart Office Environment
SK T-Tower is the headquarters of SK Telecom. Inside the building, different types of mobile devices, such as laptops, smartphones and tablets, are in use, and with the increase in WLAN traffic and the use of quality multimedia data, the volume of wireless data sees an explosive growth. Users want limitless Internet access in various places in addition to designated areas.