Customer Company Size
Large Corporate
Region
- America
Country
- United States
Product
- Med-Gemini
- Healthcare Data Engine
- Vertex AI Search for Healthcare
Tech Stack
- Generative AI
- Machine Learning
- Large Language Models (LLMs)
Implementation Scale
- Enterprise-wide Deployment
Impact Metrics
- Innovation Output
- Cost Savings
- Productivity Improvements
Technology Category
- Analytics & Modeling - Generative AI
- Analytics & Modeling - Machine Learning
- Platform as a Service (PaaS) - Data Management Platforms
Applicable Industries
- Life Sciences
- Pharmaceuticals
Applicable Functions
- Product Research & Development
- Quality Assurance
Use Cases
- Digital Twin
- Predictive Quality Analytics
- Remote Patient Monitoring
Services
- Cloud Planning, Design & Implementation Services
- Data Science Services
- System Integration
About The Customer
Google Cloud is a leading provider of cloud computing services, offering a range of products and solutions designed to support various industries, including life sciences. The company has made significant investments in AI and machine learning, focusing on applications that can transform drug discovery and development. Google Cloud partners with organizations like Superluminal Medicines, Ginkgo Bioworks, and Bayer to leverage its advanced technologies for innovative biotech applications. These partnerships aim to enhance drug discovery efforts, streamline regulatory processes, and improve the management and analysis of complex healthcare data. Google Cloud's commitment to innovation and its extensive expertise in AI make it a key player in the life sciences sector, driving advancements that have the potential to significantly impact the industry.
The Challenge
The life sciences industry faces significant challenges in drug discovery, including the need for faster identification of promising drug targets and streamlined clinical trials. Traditional methods are often time-consuming and costly, limiting the speed at which new therapies can be brought to market. Additionally, there is a scarcity of data available to train algorithms, particularly in the rare disease space, which hampers the development of effective treatments. Companies like Bayer are also dealing with complex regulatory processes that require substantial automation to improve efficiency. The industry is in need of innovative solutions that can leverage advanced technologies like AI and machine learning to overcome these hurdles and accelerate the drug development process.
The Solution
Google Cloud is leveraging its advanced AI and machine learning capabilities to transform drug discovery and development in the life sciences industry. The company has developed Med-Gemini, a family of advanced AI models specifically designed for medical applications, capable of processing and interpreting diverse data types within medical contexts. Google Cloud also offers specialized services like Healthcare Data Engine and Vertex AI Search for Healthcare, enabling life sciences organizations to manage and analyze complex healthcare data effectively. Through partnerships with companies like Superluminal Medicines, Google Cloud is accelerating drug discovery using AI-powered dynamic protein modeling. Superluminal's platform captures the dynamic behavior of proteins, creating candidate-ready compounds with unprecedented speed. Additionally, Google Cloud is collaborating with Ginkgo Bioworks to build large language models for diverse biotech applications, focusing on genomics, protein function, and cell engineering. Bayer is also working with Google Cloud to develop synthetic images for oncology and automate regulatory processes, significantly improving efficiency. These solutions demonstrate Google Cloud's commitment to leveraging AI to address the challenges faced by the life sciences industry, ultimately reducing time and cost in bringing new therapies to market.
Operational Impact
Quantitative Benefit
Case Study missing?
Start adding your own!
Register with your work email and create a new case study profile for your business.
Related Case Studies.
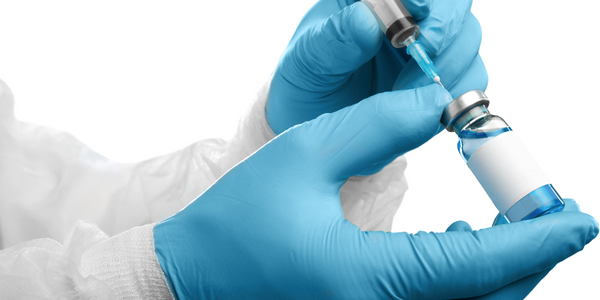
Case Study
Case Study: Pfizer
Pfizer’s high-performance computing software and systems for worldwide research and development support large-scale data analysis, research projects, clinical analytics, and modeling. Pfizer’s computing services are used across the spectrum of research and development efforts, from the deep biological understanding of disease to the design of safe, efficacious therapeutic agents.
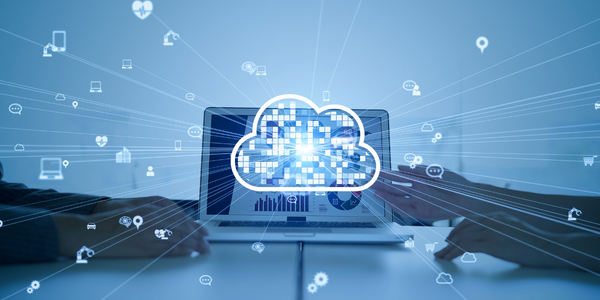
Case Study
Fusion Middleware Integration on Cloud for Pharma Major
Customer wanted a real-time, seamless, cloud based integration between the existing on premise and cloud based application using SOA technology on Oracle Fusion Middleware Platform, a Contingent Worker Solution to collect, track, manage and report information for on-boarding, maintenance and off-boarding of contingent workers using a streamlined and Integrated business process, and streamlining of integration to the back-end systems and multiple SaaS applications.
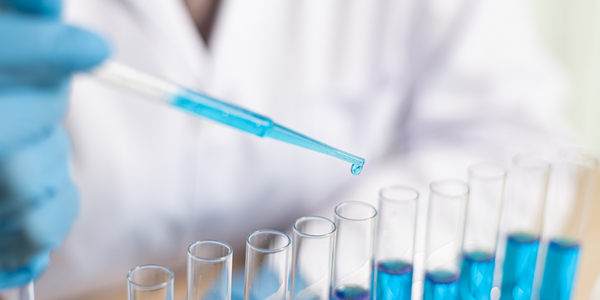
Case Study
Process Control System Support
In many automated production facilities, changes are made to SIMATIC PCS 7 projects on a daily basis, with individual processes often optimised by multiple workers due to shift changes. Documentation is key here, as this keeps workers informed about why a change was made. Furthermore, SIMATIC PCS 7 installations are generally used in locations where documentation is required for audits and certification. The ability to track changes between two software projects is not only an invaluable aid during shift changes, but also when searching for errors or optimising a PCS 7 installation. Every change made to the system is labour-intensive and time-consuming. Moreover, there is also the risk that errors may occur. If a change is saved in the project, then the old version is lost unless a backup copy was created in advance. If no backup was created, it will no longer be possible to return to the previous state if and when programming errors occur. Each backup denotes a version used by the SIMATIC PCS 7 system to operate an installation. To correctly interpret a version, information is required on WHO changed WHAT, WHERE, WHEN and WHY: - Who created the version/who is responsible for the version? - Who released the version? - What was changed in the version i.e. in which block or module of the SIMATIC PCS 7 installation were the changes made? - When was the version created? Is this the latest version or is there a more recent version? - Why were the changes made to the version? If they are part of a regular maintenance cycle, then is the aim to fix an error or to improve production processes? - Is this particular version also the version currently being used in production? The fact that SIMATIC PCS 7 projects use extremely large quantities of data complicates the situation even further, and it can take a long time to load and save information as a result. Without a sustainable strategy for operating a SIMATIC PCS 7 installation, searching for the right software version can become extremely time-consuming and the installation may run inefficiently as a result.
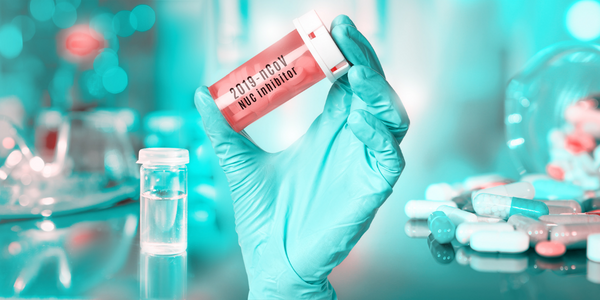
Case Study
ELI LILLY ADOPTS MICROMEDIA’S ALERT NOTIFICATION SYSTEM
Pharmaceutical production is subject to a strict set of enforced rules that must be adhered to and compliance to these standards is critically necessary. Due to the efforts of WIN 911’s strategic partner Micromedia, Lilly was able to adopt an alarm notification infrastructure that integrated smoothly with their existing workflows and emergency hardware and protocols. These raw energy sources enable the industrial process to function: electricity, WIN-911 Software | 4020 South Industrial Drive, Suite 120 | Austin, TX 78744 USA industrial steam, iced water, air mixtures of varying quality. Refrigeration towers, boilers and wastewater are monitored by ALERT. Eli Lilly identified 15000 potential variables, but limitations compelled them to chisel the variable list down to 300. This allowed all major alarms to be covered including pressure, discharge, quantity of waste water discharged,temperature, carbon dioxide content, oxygen & sulphur content, and the water’s pH.