Growing geospatial applications with quality training data
Customer Company Size
Mid-size Company
Region
- Asia
- America
- Europe
Country
- Australia
Product
- Nearmap AI
- CloudFactory Data Labeling
Tech Stack
- Proprietary Imaging Technology
- Automated Insight-Gathering Software
Implementation Scale
- Enterprise-wide Deployment
Impact Metrics
- Productivity Improvements
- Customer Satisfaction
- Innovation Output
Technology Category
- Analytics & Modeling - Computer Vision Software
- Analytics & Modeling - Machine Learning
- Functional Applications - Remote Monitoring & Control Systems
Applicable Industries
- Construction & Infrastructure
Applicable Functions
- Quality Assurance
- Product Research & Development
Use Cases
- Predictive Maintenance
- Building Automation & Control
- Remote Asset Management
Services
- Data Science Services
- System Integration
- Training
About The Customer
Nearmap, a global technology pioneer, provides easy and instant access to up-to-date and historic geospatial data to its customers. It uses proprietary technology to capture high-resolution aerial imagery of urban exteriors, providing data-rich virtual tours across rooftops, buildings, and other location features, all at a massive scale. This year, the company’s AI team added another layer of data to their traditional product offering: an automated insight-gathering software that can identify things such as the shape or material of a roof. The accuracy of Nearmap’s AI product relies on correct data labeling on a large scale. A long-term partnership with CloudFactory has removed the data annotation challenge, leaving the Nearmap team to focus on its core functions: innovating and growing operations.
The Challenge
The first among aerial imagery providers, Nearmap offers both AI analysis and high-definition aerial images with accuracy on a commercial scale. Its geospatial data can help speed up and simplify many organizations’ workflows, from insurance underwriting to property appraisals, by processing virtual location data without the need for in-person inspections. Automating AI data sets requires up-to-date information and labor-intensive data labeling. When an AI component was first introduced to Nearmap’s offering, data labeling was done in-house, distracting the team from developing software. The Nearmap team quickly realized that to expand the business, they would need outside help. In addition to the sheer volume of images that need processing, a key challenge is the variety of imagery assets, and the different ways they must be labeled. Nearmap had to develop an in-house labeling tool, as no commercial tool exists that could meet their imaging requirements, which change frequently. Business growth meant finding a reliable partner that understood the complexities of what was required, could learn and work with the proprietary tooling, and ensured fast delivery of high-quality labeling work.
The Solution
CloudFactory took over the Nearmap data labeling function, quickly onboarding additional team members as needed so the Nearmap team could begin to scale up operations. 'One of the key factors that influenced our decision to partner with CloudFactory was quality. Everything I knew about crowdsourced labeling was that you have to very carefully gamify your solutions, because the labelers are just understandably trying to maximize their reward, with as little effort as possible. With a trained team, you get something you simply can’t with crowdsourcing—accountability. In retrospect, this has had a huge impact for us, because the biggest limiting factor on the performance of the models is actually the quality of the labels, and how precise the definitions are. Discussing endless corner cases on a weekly basis with the team, and how they are to be treated, is hugely important,' said Michael Bewley, Senior Director of AI Systems at Nearmap. It’s a relationship that has gone from strength to strength in the three and a half years since the two companies began working together. The partnership started at just 200 hours of work per month and has grown 1 to 2 orders of magnitude above that in the time since. Working with CloudFactory means those additional hours have made a positive impact on the communities where the work is completed; a fact that appeals to clients like Nearmap. Ongoing training, quality reviews, and an understanding of changing client needs gives the Nearmap team confidence their decision to hand the data labeling function to CloudFactory was a sound business move.
Operational Impact
Quantitative Benefit
Case Study missing?
Start adding your own!
Register with your work email and create a new case study profile for your business.
Related Case Studies.
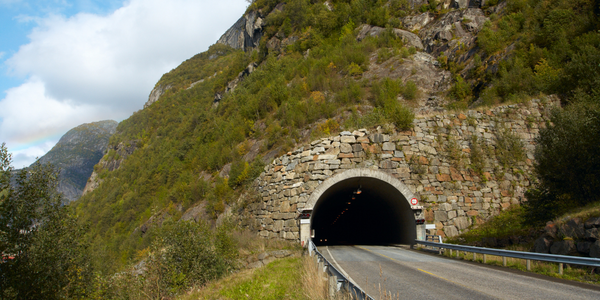
Case Study
IoT System for Tunnel Construction
The Zenitaka Corporation ('Zenitaka') has two major business areas: its architectural business focuses on structures such as government buildings, office buildings, and commercial facilities, while its civil engineering business is targeted at structures such as tunnels, bridges and dams. Within these areas, there presented two issues that have always persisted in regard to the construction of mountain tunnels. These issues are 'improving safety" and "reducing energy consumption". Mountain tunnels construction requires a massive amount of electricity. This is because there are many kinds of electrical equipment being used day and night, including construction machinery, construction lighting, and ventilating fan. Despite this, the amount of power consumption is generally not tightly managed. In many cases, the exact amount of power consumption is only ascertained when the bill from the power company becomes available. Sometimes, corporations install demand-monitoring equipment to help curb the maximum power demanded. However, even in these cases, the devices only allow the total volume of power consumption to be ascertained, or they may issue warnings to prevent the contracted volume of power from being exceeded. In order to tackle the issue of reducing power consumption, it was first necessary to obtain an accurate breakdown of how much power was being used in each particular area. In other words, we needed to be able to visualize the amount of power being consumed. Safety, was also not being managed very rigorously. Even now, tunnel construction sites often use a 'name label' system for managing entry into the work site. Specifically, red labels with white reverse sides that bear the workers' names on both sides are displayed at the tunnel work site entrance. The workers themselves then flip the name label to the appropriate side when entering or exiting from the work site to indicate whether or not they are working inside the tunnel at any given time. If a worker forgets to flip his or her name label when entering or exiting from the tunnel, management cannot be performed effectively. In order to tackle the challenges mentioned above, Zenitaka decided to build a system that could improve the safety of tunnel construction as well as reduce the amount of power consumed. In other words, this new system would facilitate a clear picture of which workers were working in each location at the mountain tunnel construction site, as well as which processes were being carried out at those respective locations at any given time. The system would maintain the safety of all workers while also carefully controlling the electrical equipment to reduce unnecessary power consumption. Having decided on the concept, our next concern was whether there existed any kind of robust hardware that would not break down at the construction work site, that could move freely in response to changes in the working environment, and that could accurately detect workers and vehicles using radio frequency identification (RFID). Given that this system would involve many components that were new to Zenitaka, we decided to enlist the cooperation of E.I.Sol Co., Ltd. ('E.I.Sol') as our joint development partner, as they had provided us with a highly practical proposal.
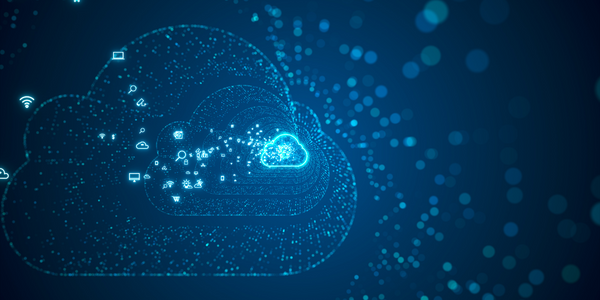
Case Study
Splunk Partnership Ties Together Big Data & IoT Services
Splunk was faced with the need to meet emerging customer demands for interfacing IoT projects to its suite of services. The company required an IoT partner that would be able to easily and quickly integrate with its Splunk Enterprise platform, rather than allocating development resources and time to building out an IoT interface and application platform.
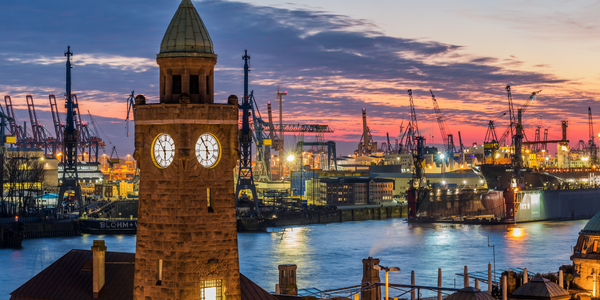
Case Study
Bridge monitoring in Hamburg Port
Kattwyk Bridge is used for both rail and road transport, and it has played an important role in the Port of Hamburg since 1973. However, the increasing pressure from traffic requires a monitoring solution. The goal of the project is to assess in real-time the bridge's status and dynamic responses to traffic and lift processes.
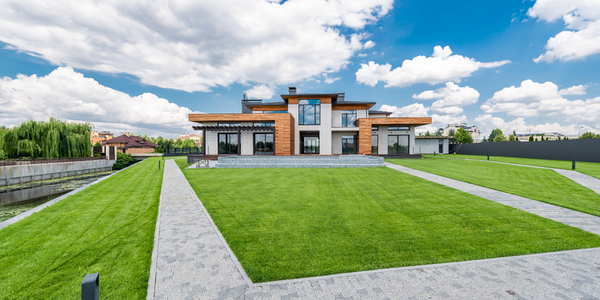
Case Study
Bellas Landscaping
Leading landscaping firm serving central Illinois streamlines operations with Samsara’s real-time fleet tracking solution: • 30+ vehicle fleet includes International Terrastar dump trucks and flatbeds, medium- and light-duty pickups from Ford and Chevrolet. Winter fleet includes of snow plows and salters.
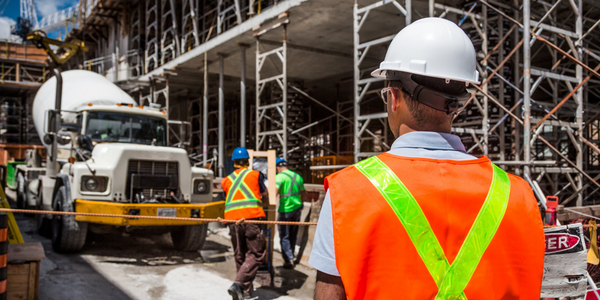
Case Study
Condition Based Monitoring for Industrial Systems
A large construction aggregate plant operates 10 high horsepower Secondary Crusher Drive Motors and associated conveyor belts, producing 600 tons of product per hour. All heavy equipment requires maintenance, but the aggregate producer’s costs were greatly magnified any time that the necessary maintenance was unplanned and unscheduled. The product must be supplied to the customers on a tight time schedule to fulfill contracts, avoid penalties, and prevent the loss of future business. Furthermore, a sudden failure in one of the drive motors would cause rock to pile up in unwanted locations, extending the downtime and increasing the costs.Clearly, preventative maintenance was preferable to unexpected failures. So, twice each year, the company brought in an outside vendor to attach sensors to the motors, do vibration studies, measure bearing temperatures and attempt to assess the health of the motors. But that wasn’t enough. Unexpected breakdowns continued to occur. The aggregate producer decided to upgrade to a Condition Based Monitoring (CBM) sensor system that could continually monitor the motors in real time, apply data analytics to detect changes in motor behavior before they developed into major problems, and alert maintenance staff via email or text, anywhere they happened to be.A wired sensor network would have been cost prohibitive. An aggregate plant has numerous heavy vehicles moving around, so any cabling would have to be protected. But the plant covers 400 acres, and the cable would have to be trenched to numerous locations. Cable wasn’t going to work. The aggregate producer needed a wireless solution.