Customer Company Size
Large Corporate
Region
- America
Country
- United States
Product
- Cortical.io Support Intelligence
Tech Stack
- Unsupervised Machine Learning
Implementation Scale
- Enterprise-wide Deployment
Impact Metrics
- Productivity Improvements
- Customer Satisfaction
Technology Category
- Analytics & Modeling - Machine Learning
Applicable Industries
- Electronics
Services
- Data Science Services
About The Customer
The customer is a Fortune 100 Technology Manufacturer. They are a large-scale company with a complex networking environment. Their support engineers handle a multitude of support cases that often refer to complicated technical issues. The company's internal documentation uses specific terminology, which often differs from the language used by their customers. This discrepancy in language use further complicates the resolution of support cases. The company had previously attempted to reduce the time to find meaningful results with other search-based solutions, but these attempts failed to improve the support team’s productivity.
The Challenge
The support cases handled by the company’s support engineers were difficult and time-consuming to resolve because they referred to complicated technical issues in a complex networking environment. The fact that customers often used different terminology than what is used in the company internal documentation made the task even more difficult. Efficient handling of support cases was predicated on finding a solution from past cases instead of troubleshooting the issue from scratch. Attempts to reduce the time to find meaningful results with other search-based solutions failed, as they were not able to quickly and consistently identify similar support cases and did not improve the support team’s productivity.
The Solution
Cortical.io Support Intelligence was rapidly trained in an unsupervised machine-learning approach using support cases. The solution’s patented technology overcame the problems of language ambiguity and vocabulary mismatch by analyzing not just keywords but also the meaning of whole support cases, including customers’ written requests, engineers’ notes, email exchanges, and the meaning of sections of text from support documents, long or short. This allowed the solution to quickly provide the support engineers with the most applicable documents to the support case. As new material became available, it was ingested, indexed and automatically became searchable. Based on support engineer feedback, the system also continuously learned to assess the quality of the applicable documents.
Operational Impact
Quantitative Benefit
Case Study missing?
Start adding your own!
Register with your work email and create a new case study profile for your business.
Related Case Studies.
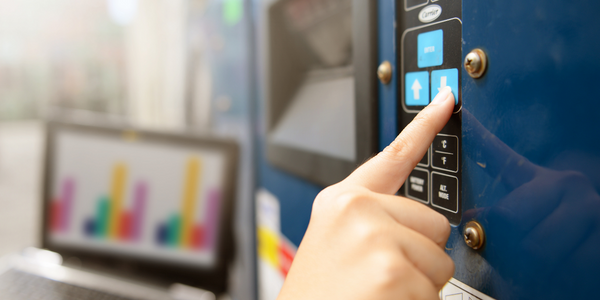
Case Study
Remote Temperature Monitoring of Perishable Goods Saves Money
RMONI was facing temperature monitoring challenges in a cold chain business. A cold chain must be established and maintained to ensure goods have been properly refrigerated during every step of the process, making temperature monitoring a critical business function. Manual registration practice can be very costly, labor intensive and prone to mistakes.
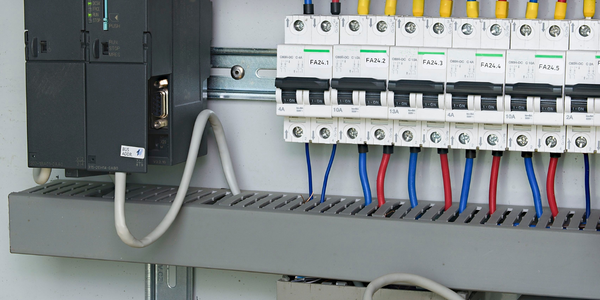
Case Study
Predictive maintenance in Schneider Electric
Schneider Electric Le Vaudreuil factory in France is recognized by the World Economic Forum as one of the world’s top nine most advanced “lighthouse” sites, applying Fourth Industrial Revolution technologies at large scale. It was experiencing machine-health and unplanned downtime issues on a critical machine within their manufacturing process. They were looking for a solution that could easily leverage existing machine data feeds, be used by machine operators without requiring complex setup or extensive training, and with a fast return on investment.
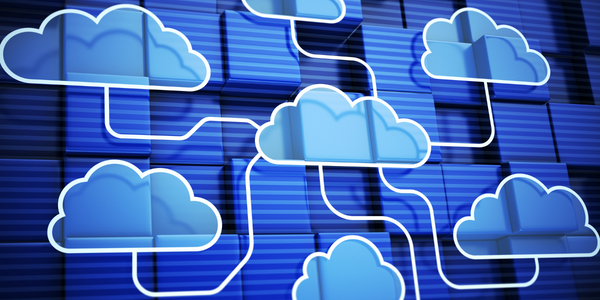
Case Study
Cloud Solution for Energy Management Platform-Schneider Electric
Schneider Electric required a cloud solution for its energy management platform to manage high computational operations, which were essential for catering to client requirements. As the business involves storage and analysis of huge amounts of data, the company also needed a convenient and scalable storage solution to facilitate operations efficiently.
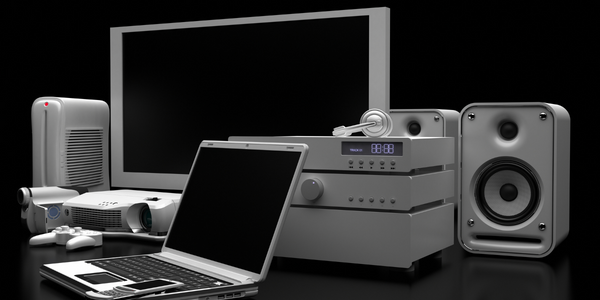
Case Study
Leveraging the IoT to Gain a Competitive Edge in International Competition
Many large manufacturers in and outside Japan are competing for larger market share in the same space, expecting a growing demand for projectors in the areas of entertainment, which requires glamor and strong visual performance as well as digital signage that can attract people’s attention. “It is becoming more and more difficult to differentiate ourselves with stand-alone hardware products,” says Kazuyuki Kitagawa, Director of Service & Support at Panasonic AVC Networks. “In order for Panasonic to grow market share and overall business, it is essential for us to develop solutions that deliver significant added value.” Panasonic believes projection failure and quality deterioration should never happen. This is what and has driven them to make their projectors IoT-enabled. More specifically, Panasonic has developed a system that collects data from projectors, visualizes detailed operational statuses, and predicts issues and address them before failure occurs. Their projectors are embedded with a variety of sensors that measure power supply, voltage, video input/ output signals, intake/exhaust air temperatures, cooling fan operations, and light bulb operating time. These sensors have been used to make the projector more intelligent, automatically suspending operation when the temperature rises excessively, and automatically switching light bulbs. Although this was a great first step, Panasonic projectors were still not equipped with any capability to send the data over a network.