Customer Company Size
Large Corporate
Region
- America
Country
- United States
Product
- Cortical.io SemanticPro
Tech Stack
- Natural Language Processing
Implementation Scale
- Enterprise-wide Deployment
Impact Metrics
- Productivity Improvements
- Cost Savings
- Digital Expertise
Technology Category
- Analytics & Modeling - Natural Language Processing (NLP)
Applicable Industries
- Finance & Insurance
Applicable Functions
- Business Operation
Use Cases
- Fraud Detection
- Regulatory Compliance Monitoring
Services
- Data Science Services
About The Customer
The customer is a Tier 1 bank in the US. The bank was exploring ways to improve efficiencies through automation. The bank’s goal was to find an appropriate extraction tool to help automate the risk assessment process and, provided the quality of results is sufficiently reliable, reduce the operational risks of wrong covenant assessments. The bank's VP Strategy & Emerging Technologies, Martha, was responsible for identifying areas where automation can improve process efficiencies in the organization. She continuously scouts new technologies to keep abreast of developments on the market and conducts internal interviews with line-of-business owners to assess optimization potentials. One of the areas identified for improvement was the wholesale credit department, headed by Rick, whose team spent a lot of time thoroughly reviewing financial information in order to assess credit risks.
The Challenge
The bank was looking for ways to improve efficiencies through automation, particularly in the area of credit risk assessment. The complexity in language and structure of wholesale credit agreements made automation difficult. These agreements are complex, 100+-page-documents that are very bespoke because banks use different lending systems. Especially covenants use highly specific, use-case related formulations. Some extractions are straightforward, and the values can be extracted “as is” (no inference necessary), for example: named dates, commitments, rates, parties. Others are more challenging and require fine-grained distinctions between options or covenants (eg redeem deal options, termination options). Here an automation system has to comprehend domain logic based on document structure and relations of extracted items. Pricing-related tables contain many different parameters (applicable margin, interest payment schedules, commitment tables) and it is extremely difficult to automate the extraction of pricing information. This is why the review of credit agreements was still done manually, which cost a lot of time and money to the bank, not mentioning the high error rate of such a mundane task.
The Solution
The bank leveraged the meaning-based capabilities of Cortical.io SemanticPro to extract key information from credit agreements and automate the classification of covenants. After having looked at the different solutions on the market, Martha decided to test Cortical.io natural language processing capabilities and apply them to the analysis of credit agreements. The main reason why she kicked-off a project with Cortical. io was the easiness and speed with which the system could be adapted to Rick’s use case: only a limited amount of example credit agreements (less than 50) was needed for training the system. This greatly limited both the initial investment and overall risk of this project. After an initial base knowledge phase where the system had learnt from relevant source material in an unsupervised manner, two loan officers from Rick’s team provided input by reviewing a small set of documents. After that, the system kept incorporating feedback and created more accurate models (continuous learning, aka human in the loop).
Operational Impact
Quantitative Benefit
Case Study missing?
Start adding your own!
Register with your work email and create a new case study profile for your business.
Related Case Studies.
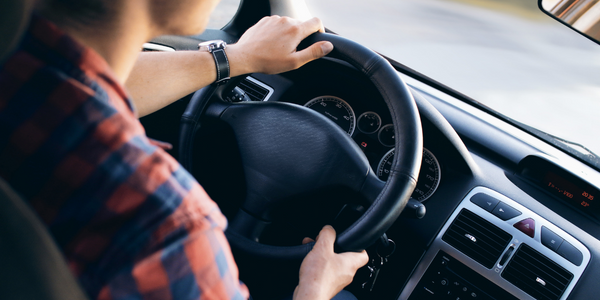
Case Study
Real-time In-vehicle Monitoring
The telematic solution provides this vital premium-adjusting information. The solution also helps detect and deter vehicle or trailer theft – as soon as a theft occurs, monitoring personnel can alert the appropriate authorities, providing an exact location.“With more and more insurance companies and major fleet operators interested in monitoring driver behaviour on the grounds of road safety, efficient logistics and costs, the market for this type of device and associated e-business services is growing rapidly within Italy and the rest of Europe,” says Franco.“The insurance companies are especially interested in the pay-per-use and pay-as-you-drive applications while other organisations employ the technology for road user charging.”“One million vehicles in Italy currently carry such devices and forecasts indicate that the European market will increase tenfold by 2014.However, for our technology to work effectively, we needed a highly reliable wireless data network to carry the information between the vehicles and monitoring stations.”
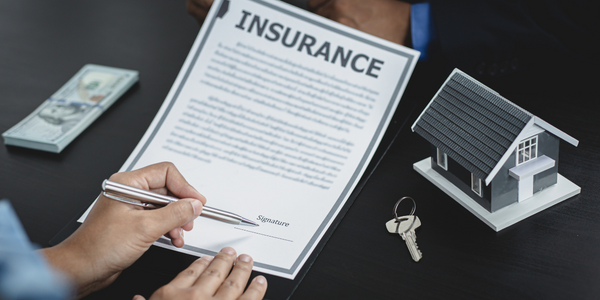
Case Study
Safety First with Folksam
The competitiveness of the car insurance market is driving UBI growth as a means for insurance companies to differentiate their customer propositions as well as improving operational efficiency. An insurance model - usage-based insurance ("UBI") - offers possibilities for insurers to do more efficient market segmentation and accurate risk assessment and pricing. Insurers require an IoT solution for the purpose of data collection and performance analysis
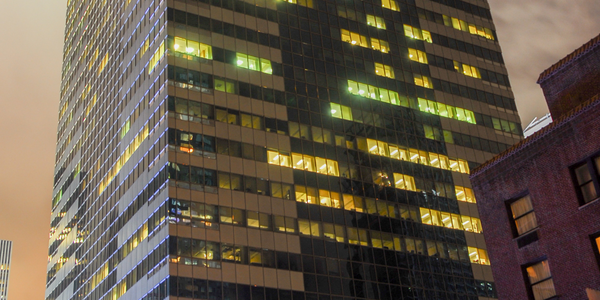
Case Study
Smooth Transition to Energy Savings
The building was equipped with four end-of-life Trane water cooled chillers, located in the basement. Johnson Controls installed four York water cooled centrifugal chillers with unit mounted variable speed drives and a total installed cooling capacity of 6,8 MW. Each chiller has a capacity of 1,6 MW (variable to 1.9MW depending upon condenser water temperatures). Johnson Controls needed to design the equipment in such way that it would fit the dimensional constraints of the existing plant area and plant access route but also the specific performance requirements of the client. Morgan Stanley required the chiller plant to match the building load profile, turn down to match the low load requirement when needed and provide an improvement in the Energy Efficiency Ratio across the entire operating range. Other requirements were a reduction in the chiller noise level to improve the working environment in the plant room and a wide operating envelope coupled with intelligent controls to allow possible variation in both flow rate and temperature. The latter was needed to leverage increased capacity from a reduced number of machines during the different installation phases and allow future enhancement to a variable primary flow system.
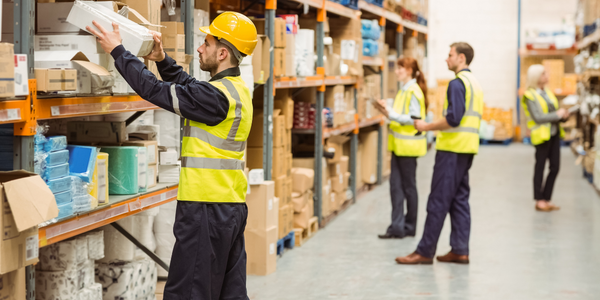
Case Study
Automated Pallet Labeling Solution for SPR Packaging
SPR Packaging, an American supplier of packaging solutions, was in search of an automated pallet labeling solution that could meet their immediate and future needs. They aimed to equip their lines with automatic printer applicators, but also required a solution that could interface with their accounting software. The challenge was to find a system that could read a 2D code on pallets at the stretch wrapper, track the pallet, and flag any pallets with unread barcodes for inspection. The pallets could be single or double stacked, and the system needed to be able to differentiate between the two. SPR Packaging sought a system integrator with extensive experience in advanced printing and tracking solutions to provide a complete traceability system.
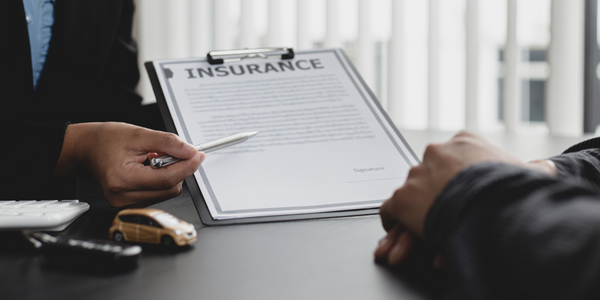
Case Study
Transforming insurance pricing while improving driver safety
The Internet of Things (IoT) is revolutionizing the car insurance industry on a scale not seen since the introduction of the car itself. For decades, premiums have been calculated using proxy-based risk assessment models and historical data. Today, a growing number of innovative companies such as Quebec-based Industrielle Alliance are moving to usage-based insurance (UBI) models, driven by the advancement of telematics technologies and smart tracking devices.
Case Study
Enhancing Security and Compliance in Remitly's Global Money Transfer Service with Fastly
Remitly, an online remittance service, was faced with the challenge of securing its proprietary global transfer network. The company needed a security solution that could meet PCI requirements and protect customers' sensitive transactions through its mobile application. The solution had to be capable of defending against new and emerging attack types without impacting performance. Remitly also had to deal with irregular traffic patterns, such as a sudden spike in account transfers from a small network segment on the Pacific coastline of South America. The company needed to determine in real time whether such traffic indicated an attack or valid requests. A traditional web application firewall (WAF) would not be able to distinguish this traffic, potentially leading to customer frustration if the IP was blacklisted.