Customer Company Size
Large Corporate
Product
- Cortical.io Semantic Search
Tech Stack
- Natural Language Understanding
Implementation Scale
- Enterprise-wide Deployment
Impact Metrics
- Productivity Improvements
- Digital Expertise
Technology Category
- Analytics & Modeling - Natural Language Processing (NLP)
Applicable Industries
- Automotive
Applicable Functions
- Product Research & Development
Use Cases
- Process Control & Optimization
Services
- Data Science Services
About The Customer
The customer is a leading car manufacturer. The company was dealing with a growing number of requirements that were described in multiple documents and databases. Thousands of specification sheets existed, each of them containing on average 500 requirements. The company was struggling with the lack of information about whether a requirement relates to a specific project or to multiple projects, and there was no easy way to compare requirements across projects. The requirement engineers had to manually copy-paste requirements and compare them before using them in new documents. The company had previously attempted to automate this task but failed due to the complexity of the requirements and the lack of standardization in the specification sheets.
The Challenge
The leading car manufacturer was dealing with a growing number of requirements (over 150,000) that were described in multiple documents and databases. Thousands of specification sheets existed, each of them containing on average 500 requirements. There was no information about whether a requirement relates to a specific project or to multiple projects, nor an easy way to compare requirements across projects. The requirement engineers had to copy-paste requirements and manually compare them, before using them in new documents. Previous attempts to automate this task had failed, because similar requirements formulated in different ways could not be identified and because requirement texts are often too short to convey a meaning to conventional search engines. The fact that many specification sheets contain complex tables from which requirements must be extracted and that specification sheets do not have a standard structure made it even more difficult for current approaches to solve the problem.
The Solution
The company decided to use Cortical.io Semantic Search to automate the requirement analysis process. The tool was trained with reference data from the car manufacturer’s language domain (specification sheets, indices of abbreviations, company-specific standards, industry standards, automotive engineering literature, etc.) and evaluated against a Gold Standard of requirements for which correct entries had been specified in the respective specifications. The solution was able to match different formulations of the same requirement, even when the text was short, providing requirement engineers with a transparent overview of specification details across projects. The solution enabled them to easily compare specifications, which helped them make sure their own specification sheet is complete and up to the company standards. The comparison table also allowed them to identify and question variations of requirements across similar projects.
Operational Impact
Quantitative Benefit
Case Study missing?
Start adding your own!
Register with your work email and create a new case study profile for your business.
Related Case Studies.
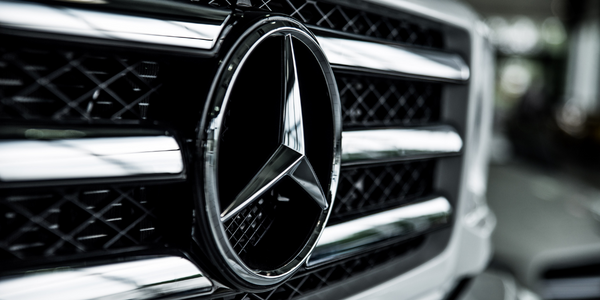
Case Study
Integral Plant Maintenance
Mercedes-Benz and his partner GAZ chose Siemens to be its maintenance partner at a new engine plant in Yaroslavl, Russia. The new plant offers a capacity to manufacture diesel engines for the Russian market, for locally produced Sprinter Classic. In addition to engines for the local market, the Yaroslavl plant will also produce spare parts. Mercedes-Benz Russia and his partner needed a service partner in order to ensure the operation of these lines in a maintenance partnership arrangement. The challenges included coordinating the entire maintenance management operation, in particular inspections, corrective and predictive maintenance activities, and the optimizing spare parts management. Siemens developed a customized maintenance solution that includes all electronic and mechanical maintenance activities (Integral Plant Maintenance).
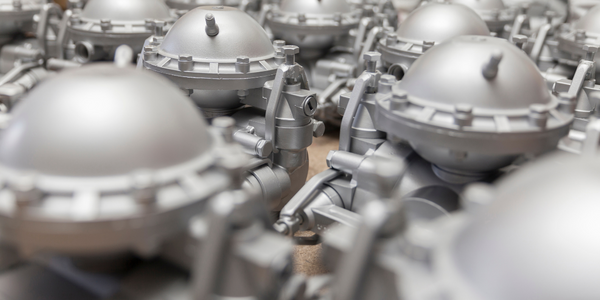
Case Study
Monitoring of Pressure Pumps in Automotive Industry
A large German/American producer of auto parts uses high-pressure pumps to deburr machined parts as a part of its production and quality check process. They decided to monitor these pumps to make sure they work properly and that they can see any indications leading to a potential failure before it affects their process.