Customer Company Size
Large Corporate
Region
- America
Country
- United States
Product
- Sift Content Integrity
Tech Stack
- Machine Learning
- Data Integration
Implementation Scale
- Enterprise-wide Deployment
Impact Metrics
- Productivity Improvements
- Cost Savings
Technology Category
- Analytics & Modeling - Machine Learning
Applicable Functions
- Sales & Marketing
Use Cases
- Fraud Detection
- Automated Disease Diagnosis
Services
- Data Science Services
About The Customer
The customer is a growing email marketing platform that aims to help businesses connect with their valued customers. The platform enables messaging, which is generally trusted by consumers but can be leveraged to send fraudulent messages. As the company began to scale, they found their manual vetting processes insufficient to keep up with the pace of growth. They needed a solution that could prevent fraud attacks from reaching end users and consumers, who could fall victim to fraud unknowingly enabled by the platform.
The Challenge
The email marketing platform was facing a significant challenge due to the susceptibility of the marketing technology industry to fraud attacks. The scale and severity of spam and scams were increasing, putting the onus on sending providers to protect the health of their network. As the company began to scale their business faster than their manual vetting processes would allow, they needed a solution that could keep up. They were looking for a solution that offered uptime, affordability at scale, model customization, data sharing and app integrations, and the ability to automate common support tasks such as account disablement.
The Solution
The company chose Sift for its positive experience using Sift during a prior working relationship and the ease of integration. The customization of the Sift model was a major factor, as the model learns based on trends specific to the company’s user base and unique fraud signals to more accurately detect abuse. Sift also gives them the ability to observe user-level risk as well as content-level risk to get a clearer picture of fraud on their platform. They began using Sift Content Integrity to identify the full scope of their fraud problem, and to help automatically squash abuse before it starts. The content model implementation makes it simple to integrate data and understand which actions trigger workflows. They’re now able to identify groups of fraudsters and associated accounts to keep fraud rings from compromising their ecosystem.
Operational Impact
Quantitative Benefit
Case Study missing?
Start adding your own!
Register with your work email and create a new case study profile for your business.
Related Case Studies.
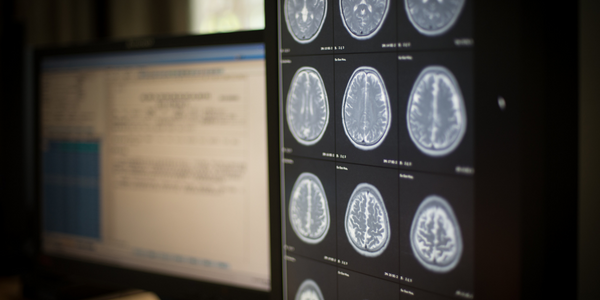
Case Study
Artificial Intelligence and the implications on Medical Imaging
There are several factors simultaneously driving integration of AI in radiology. Firstly, in many countries around the world there is a discrepancy between the number of doctors trained in radiology and the rising demand for diagnostic imaging. This leads to greater demands for work efficiency and productivity. For example, the number of radiology specialists (consultant work- force) in England went up 5% between 2012 and 2015, while in the same period the number of CT and MR scans increased by 29 and 26 percentage points respectively. In Scotland, the gap widened even further (The Royal College of Radiologists 2016). Today, the average radiologist is interpreting an image every three to four seconds, eight hours a day (Choi et al. 2016).Secondly, the image resolution of today’s scanners is continuously improving – resulting in an ever greater volume of data. Indeed, the estimated overall medical data volume doubles every three years, making it harder and harder for radiologists to make good use of the available information without extra help from computerized digital processing. It is desirable, both in radiological research and in clinical diagnostics, to be able to quantitatively analyze this largely unexploited wealth of data and, for example, utilize new measurable imaging biomarkers to assess disease progression and prognosis (O’Connor et al. 2017). Experts see considerable future potential in the transformation of radiology from a discipline of qualitative interpretation to one of quantita- tive analysis, which derives clinically relevant information from extensive data sets (“radiomics”). “Images are more than pictures, they are data,” American radiologist Robert Gillies and his colleagues write (Gillies et al. 2016). Of course, this direction for radiology will require powerful, automated procedures, some of which at least will come under the field of artificial intelligence.
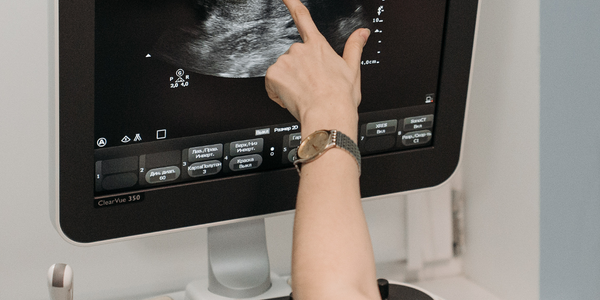
Case Study
Improving Diagnosis Accuracy and Saving Lives
Dr. Partho Sengupta needed a way to accurately identify disease patterns resulting from echocardiograms in order to improve diagnostics and save more lives. Specifically, he wanted to distinguish between two disparate diseases: cardiomyopathy, which directly impacts the heart muscle and often leads to heart failure, and pericarditis, which acts as if the heart is involved but doesn’t actually affect the heart. While both diseases present with similar heart conditions, the treatments are vastly different. For pericarditis, the treatment may include medication and, rarely, surgery. However, if the diagnosis is cardiomyopathy the patient undergoes medical management (i.e. a pacemaker) or in extreme cases, a heart transplant. Misdiagnosis of these disease conditions can put the patient’s life at risk and be very expensive for the hospital. Dr. Sengupta, therefore, looked to Saffron’s Natural Intelligence Platform to help his team increase the diagnosis accuracy of these medical conditions.
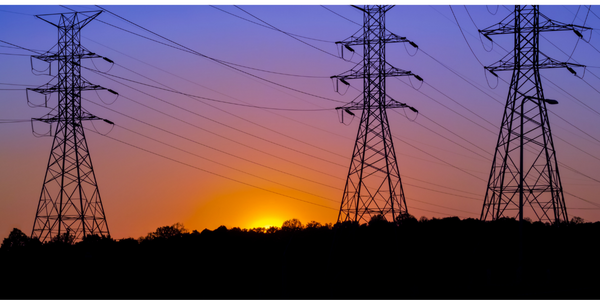
Case Study
Largest Production Deployment of AI and IoT Applications
To increase efficiency, develop new services, and spread a digital culture across the organization, Enel is executing an enterprise-wide digitalization strategy. Central to achieving the Fortune 100 company’s goals is the large-scale deployment of the C3 AI Suite and applications. Enel operates the world’s largest enterprise IoT system with 20 million smart meters across Italy and Spain.
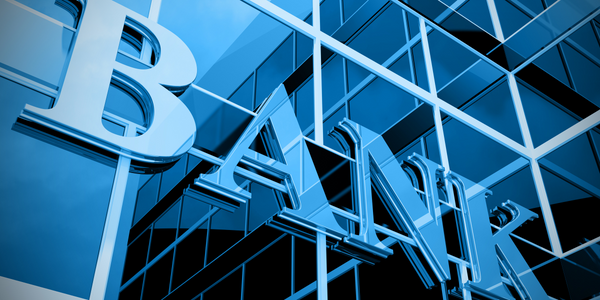
Case Study
KeyBank's Digital Transformation with Confluent's Data in Motion
KeyBank, one of the nation's largest bank-based financial services companies, embarked on a national digital bank initiative following the acquisition of Laurel Road, a digital consumer lending business. The initiative aimed to build a digital bank focused on healthcare professionals looking to refinance student loans and buy homes. A significant challenge was reducing the time to market for new products by democratizing data and decoupling systems across the IT landscape. Like many large enterprises, KeyBank had a variety of vendor applications, custom applications, and other systems that were tightly coupled to one another. New projects often required developing specific point-to-point integrations for exchanging data, which did not address the needs of other downstream systems that could benefit from the same data.
Case Study
BharatPe: Leveraging Google Cloud for Enhanced Data Analytics and AI to Promote Digital Payments
BharatPe, a fintech company founded in 2018, aimed to make digital payments more accessible for over 10 million small offline merchants and kirana store owners in India. However, the company faced challenges in managing the massive amounts of data generated daily from payment processing to business analysis. Prior to using Google Cloud, BharatPe managed its legacy data warehouse with limited capacity to run a large number of queries. The company ran key performance indicator (KPI) reports, without the ability to understand real-time data patterns. Loading three months of data for quarterly reports took more than 30 minutes on the legacy system, and in some cases, queries failed because the system could not scale to support analytical needs. Additionally, BharatPe operates in a multi-cloud environment for disaster recovery and needed a data platform that could run queries against data, regardless of where it resides.
Case Study
Barclays Enhances Customer Experience with IBM BPM Solution
Barclays, a global bank operating in over 50 countries and serving nearly 60 million customers, faced a significant challenge in improving and streamlining the customer experience. The bank's relationships with customers were multifaceted, with customers engaging with the bank through various channels such as mobile, online, and branch locations. The bank had identified 400 different customer journeys, and the challenge was to align these across the enterprise and improve them in a way that positively impacted customers quickly. The director of operations at Barclays, Mike Gamble, recognized the need to transform the bank's processes around customer journeys. He aimed to enhance the understanding of how various functions contribute to delivering customer experiences and then expedite the rollout time of these new processes to achieve the end goal for the customer.