Customer Company Size
Large Corporate
Region
- America
Country
- United States
Product
- Trifacta Wrangler Pro
- DataRobot
Tech Stack
- Amazon S3
- Amazon Redshift
- Amazon EMR
- AWS VPC
Implementation Scale
- Enterprise-wide Deployment
Impact Metrics
- Cost Savings
- Customer Satisfaction
- Productivity Improvements
Technology Category
- Analytics & Modeling - Machine Learning
- Analytics & Modeling - Data-as-a-Service
- Infrastructure as a Service (IaaS) - Cloud Computing
Applicable Industries
- Retail
Applicable Functions
- Business Operation
- Sales & Marketing
Use Cases
- Fraud Detection
Services
- Data Science Services
- System Integration
About The Customer
Consensus Corporation is a subsidiary of Target that simplifies the complex process of selling connected devices. It connects retailers with manufacturers and network operators using a single platform to enable what it calls multiplex selling: bundling technology and service purchases together, such as a smartphone and a data plan, or a smart television and a subscription to a video streaming service. Consensus enables retail stores nationwide to sell these types of connected devices and services through one unified online platform. However, a major risk for retailers selling expensive devices and services is fraudulent customer activity. To address this risk, Consensus adopted fraud prevention as one of its core services.
The Challenge
Consensus Corporation, a subsidiary of Target, simplifies the complex process of selling connected devices. However, a major risk for retailers selling expensive devices and services is fraudulent customer activity. To address this risk, Consensus adopted fraud prevention as one of its core services. Through its automated machine learning-powered online engine, Consensus can alert its retailer clients to high-risk consumers before they purchase expensive devices. To identify potential fraud, Consensus built an advanced data model that leverages huge volumes of disparate data and undergoes routine updates. In order to be able to constantly refine its predictive models and alert their retailer clients faster to potential fraud, Consensus sought out technologies that would allow it to prepare this data faster for use in its machine learning models. The painstaking process of re-engineering SQL scripts took Consensus up to six weeks (on average) to update its fraud detection machine learning model. In addition, the data preparation process required sophisticated knowledge of data science techniques, leaving the company’s product and business intelligence teams unable to perform data preparation tasks on their own.
The Solution
Consensus identified the need for better data preparation and rapid model prototyping and evaluation that did not require advanced data science knowledge. At first, the company attempted to work with several third-party data preparation solutions, but found them either expensive, unwieldy, or complicated to deploy. Finally, Harrison Lynch, Senior Director of Product Development for Consensus, discovered the DataRobot automated machine learning platform and free Trifacta Wrangler solution. Trifacta’s user-friendly UI and DataRobot’s ability to rapidly build and deploy machine learning models helped differentiate both technologies from the competition. Consensus then selected DataRobot and Trifacta Wrangler Pro on AWS to get more power and more connectivity to wrangle even more data and quickly create machine learning models. Trifacta Wrangler Pro & DataRobot are AWS–deployed solutions that seamlessly access data stored on AWS, including Amazon S3 and Amazon Redshift. In addition, Trifacta Wrangler Pro leverages Amazon EMR (Elastic MapReduce) to process the data.
Operational Impact
Quantitative Benefit
Case Study missing?
Start adding your own!
Register with your work email and create a new case study profile for your business.
Related Case Studies.
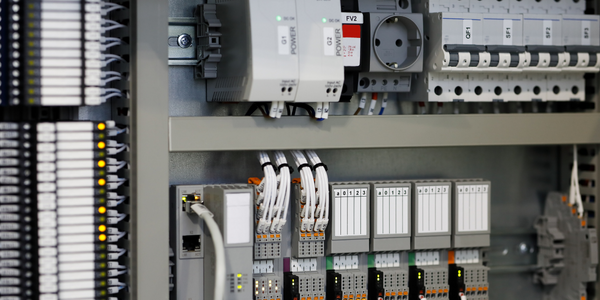
Case Study
Improving Production Line Efficiency with Ethernet Micro RTU Controller
Moxa was asked to provide a connectivity solution for one of the world's leading cosmetics companies. This multinational corporation, with retail presence in 130 countries, 23 global braches, and over 66,000 employees, sought to improve the efficiency of their production process by migrating from manual monitoring to an automatic productivity monitoring system. The production line was being monitored by ABB Real-TPI, a factory information system that offers data collection and analysis to improve plant efficiency. Due to software limitations, the customer needed an OPC server and a corresponding I/O solution to collect data from additional sensor devices for the Real-TPI system. The goal is to enable the factory information system to more thoroughly collect data from every corner of the production line. This will improve its ability to measure Overall Equipment Effectiveness (OEE) and translate into increased production efficiencies. System Requirements • Instant status updates while still consuming minimal bandwidth to relieve strain on limited factory networks • Interoperable with ABB Real-TPI • Small form factor appropriate for deployment where space is scarce • Remote software management and configuration to simplify operations
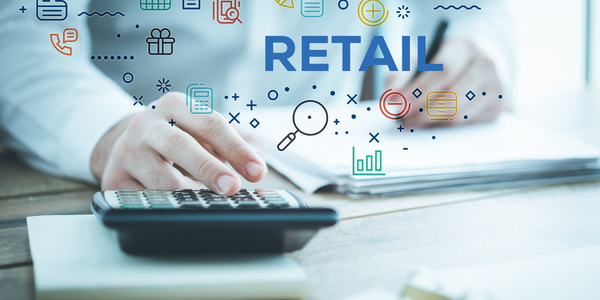
Case Study
Digital Retail Security Solutions
Sennco wanted to help its retail customers increase sales and profits by developing an innovative alarm system as opposed to conventional connected alarms that are permanently tethered to display products. These traditional security systems were cumbersome and intrusive to the customer shopping experience. Additionally, they provided no useful data or analytics.
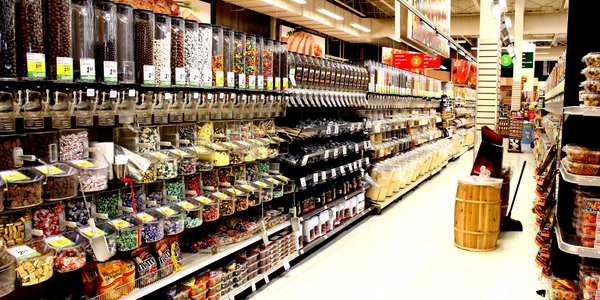
Case Study
How Sirqul’s IoT Platform is Crafting Carrefour’s New In-Store Experiences
Carrefour Taiwan’s goal is to be completely digital by end of 2018. Out-dated manual methods for analysis and assumptions limited Carrefour’s ability to change the customer experience and were void of real-time decision-making capabilities. Rather than relying solely on sales data, assumptions, and disparate systems, Carrefour Taiwan’s CEO led an initiative to find a connected IoT solution that could give the team the ability to make real-time changes and more informed decisions. Prior to implementing, Carrefour struggled to address their conversion rates and did not have the proper insights into the customer decision-making process nor how to make an immediate impact without losing customer confidence.
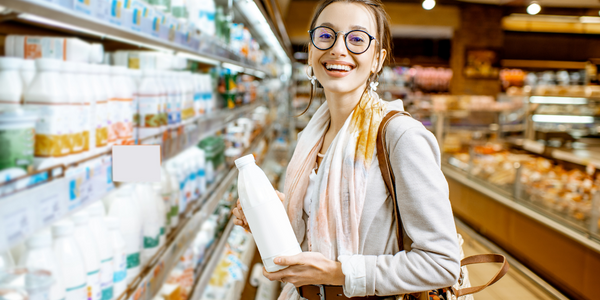
Case Study
Ensures Cold Milk in Your Supermarket
As of 2014, AK-Centralen has over 1,500 Danish supermarkets equipped, and utilizes 16 operators, and is open 24 hours a day, 365 days a year. AK-Centralen needed the ability to monitor the cooling alarms from around the country, 24 hours a day, 365 days a year. Each and every time the door to a milk cooler or a freezer does not close properly, an alarm goes off on a computer screen in a control building in southwestern Odense. This type of alarm will go off approximately 140,000 times per year, equating to roughly 400 alarms in a 24-hour period. Should an alarm go off, then there is only a limited amount of time to act before dairy products or frozen pizza must be disposed of, and this type of waste can quickly start to cost a supermarket a great deal of money.
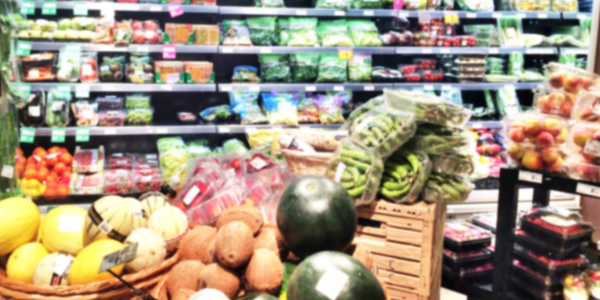
Case Study
Supermarket Energy Savings
The client had previously deployed a one-meter-per-store monitoring program. Given the manner in which energy consumption changes with external temperature, hour of the day, day of week and month of year, a single meter solution lacked the ability to detect the difference between a true problem and a changing store environment. Most importantly, a single meter solution could never identify root cause of energy consumption changes. This approach never reduced the number of truck-rolls or man-hours required to find and resolve issues.