Customer Company Size
Large Corporate
Region
- America
- Europe
Country
- United States
- Worldwide
Product
- Sift Payment Protection
- Sift Workflows
Tech Stack
- Machine Learning
- Predictive Analytics
Implementation Scale
- Enterprise-wide Deployment
Impact Metrics
- Cost Savings
- Customer Satisfaction
- Brand Awareness
Technology Category
- Analytics & Modeling - Predictive Analytics
Applicable Industries
- Consumer Goods
- Retail
Applicable Functions
- Sales & Marketing
- Business Operation
Use Cases
- Fraud Detection
- Predictive Quality Analytics
Services
- Data Science Services
- System Integration
About The Customer
Paula’s Choice is a pioneer in the skincare industry, offering cruelty-free, effective, and safe products for more than 25 years. The multinational skincare company is dedicated to science-backed research, using ingredients that are gentle for skin and non-irritating. Paula’s Choice strives to empower and enable people around the world with in-depth knowledge about skincare ingredients to help them understand how products impact their skin and how to make educated decisions. The company has a strong commitment to its customers and aims to maintain a secure, safe e-commerce platform.
The Challenge
Paula’s Choice, a multinational skincare company, was facing persistent fraud patterns on their platform, resulting in an influx of chargebacks. Fraudsters were ordering products in bulk at a discount and then shipping them to other countries to resell through eBay or Amazon for profit. To combat this, Paula’s Choice initially kept a spreadsheet and manually blocked suspicious orders, but soon discovered how challenging it was to manage and stay accurate. They turned to Sift as a solution. However, when they adopted a new payment processor, they switched from Sift Payment Protection to the payment processor’s revenue protection product, which was offered for free. This switch resulted in an immediate inundation with fraud, receiving hundreds of chargebacks—6x their normal volume.
The Solution
Paula’s Choice realized that a rules-based system was significantly less effective than Sift’s machine-learning models, and that turning off Sift had left their business vulnerable to fraudsters. With the competitor's product, the Client Services department would have needed a dedicated team to monitor orders and constantly make adjustments to rules in order to stay ahead of fraud—resources they didn’t have. The team decided to reverse course and re-implement Sift Payment Protection. Among the many things that Paula’s Choice missed while using the competitor product, was the predictive power of the Sift Score. The wealth of data and ability for automation would have been too difficult to replicate on their own. Paula’s Choice also came to rely on Sift Workflows, specifically their ease of use and the ability to quickly adapt to new fraud trends.
Operational Impact
Quantitative Benefit
Case Study missing?
Start adding your own!
Register with your work email and create a new case study profile for your business.
Related Case Studies.
.png)
Case Study
Improving Vending Machine Profitability with the Internet of Things (IoT)
The vending industry is undergoing a sea change, taking advantage of new technologies to go beyond just delivering snacks to creating a new retail location. Intelligent vending machines can be found in many public locations as well as company facilities, selling different types of goods and services, including even computer accessories, gold bars, tickets, and office supplies. With increasing sophistication, they may also provide time- and location-based data pertaining to sales, inventory, and customer preferences. But at the end of the day, vending machine operators know greater profitability is driven by higher sales and lower operating costs.
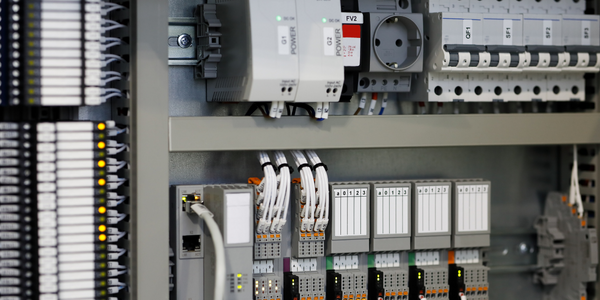
Case Study
Improving Production Line Efficiency with Ethernet Micro RTU Controller
Moxa was asked to provide a connectivity solution for one of the world's leading cosmetics companies. This multinational corporation, with retail presence in 130 countries, 23 global braches, and over 66,000 employees, sought to improve the efficiency of their production process by migrating from manual monitoring to an automatic productivity monitoring system. The production line was being monitored by ABB Real-TPI, a factory information system that offers data collection and analysis to improve plant efficiency. Due to software limitations, the customer needed an OPC server and a corresponding I/O solution to collect data from additional sensor devices for the Real-TPI system. The goal is to enable the factory information system to more thoroughly collect data from every corner of the production line. This will improve its ability to measure Overall Equipment Effectiveness (OEE) and translate into increased production efficiencies. System Requirements • Instant status updates while still consuming minimal bandwidth to relieve strain on limited factory networks • Interoperable with ABB Real-TPI • Small form factor appropriate for deployment where space is scarce • Remote software management and configuration to simplify operations
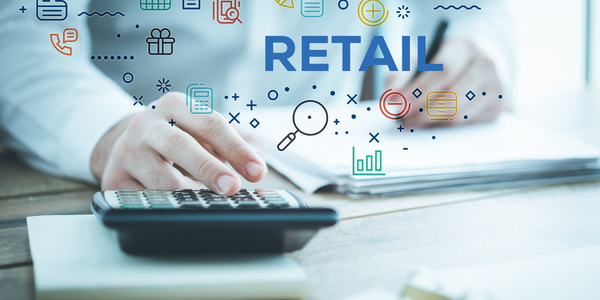
Case Study
Digital Retail Security Solutions
Sennco wanted to help its retail customers increase sales and profits by developing an innovative alarm system as opposed to conventional connected alarms that are permanently tethered to display products. These traditional security systems were cumbersome and intrusive to the customer shopping experience. Additionally, they provided no useful data or analytics.
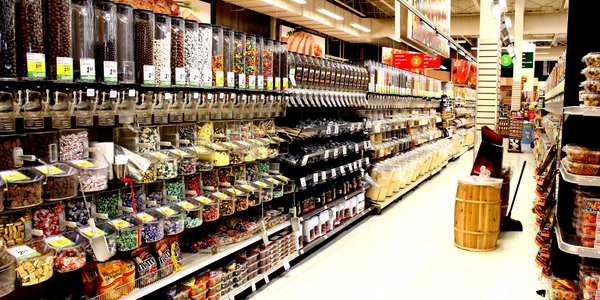
Case Study
How Sirqul’s IoT Platform is Crafting Carrefour’s New In-Store Experiences
Carrefour Taiwan’s goal is to be completely digital by end of 2018. Out-dated manual methods for analysis and assumptions limited Carrefour’s ability to change the customer experience and were void of real-time decision-making capabilities. Rather than relying solely on sales data, assumptions, and disparate systems, Carrefour Taiwan’s CEO led an initiative to find a connected IoT solution that could give the team the ability to make real-time changes and more informed decisions. Prior to implementing, Carrefour struggled to address their conversion rates and did not have the proper insights into the customer decision-making process nor how to make an immediate impact without losing customer confidence.