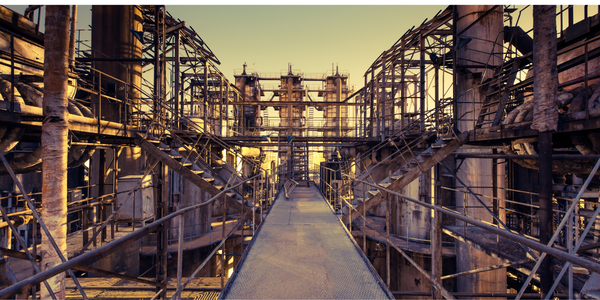
- Analytics & Modeling - Machine Learning
- Analytics & Modeling - Predictive Analytics
- Application Infrastructure & Middleware - Data Exchange & Integration
- Application Infrastructure & Middleware - Data Visualization
- Functional Applications - Remote Monitoring & Control Systems
- Sensors - Accelerometers
- Sensors - Pressure Sensors
- Sensors - Temperature Sensors
- Chemicals
- Maintenance
- Predictive Maintenance
Historically, Texmark's operations and maintenance teams monitored equipment manually and through wired sensors at a significant cost of dollars and man-hours each year in inspecting equipment on site.
Wanting to act quickly and execute on this multi-year plan, Texmark turned to Deloitte to deliver an end-to-end IIoT implementation in late 2017. Over the course of the next two years, Deloitte coordinated the following partners for the project:
1. National Instruments to outfit pumps with sensors that collect operational data
2. Hewlett Packard Enterprises to enable deployment at the edge
3. OSIsoft PI to aggregate the sensor data from the NI sources
4. SparkCognition™ to predict impending pump failures
5.Flowserve to help develop the model and data flow architecture 6. PTC ThingWorx to create a mobile-supported interface
Installation of the data acquisition hardware and edge infrastructure was completed in spring of 2018. For the first time, the Texmark operations team was able to monitor live pump behaviour from wireless sensors and react to impending failures. SparkCognitionTM could not model the asset behaviour in its SparkPredict® solution immediately, as machine learning models depend on rich, historical data, but within two months of implementation, SparkPredict was able to create accurate asset models.
Once enough data was collected, SparkCognitionTM data scientists built a model of the pump operating behaviour based on features derived from accelerometer, pressure, and temperature data. They initially began with 125 features, and with input from Texmark’s operations and maintenance team, reduced them down to the 70 features most relevant to the model’s performance. With a still-limited data set and no labelled failure data, the data scientists used an unsupervised approach to train the model. Unsupervised learning finds patterns in unlabeled data so that a subject matter expert can interpret and label them appropriately. In total, the data cleansing, feature engineering, model building, and deployment took just over one month to complete. Another SparkCognition partner, leading pump manufacturer Flowserve, was consulted for validation of the approach.
Case Study missing?
Start adding your own!
Register with your work email and create a new case study profile for your business.
Related Case Studies.
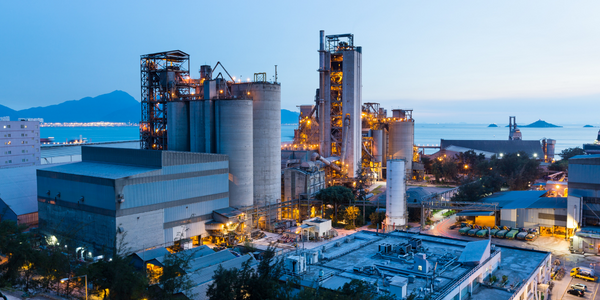
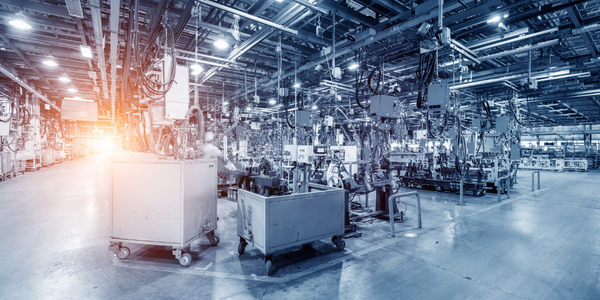
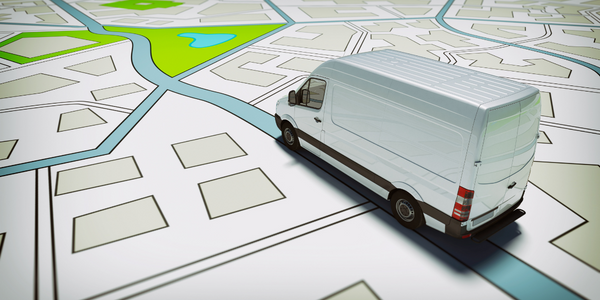
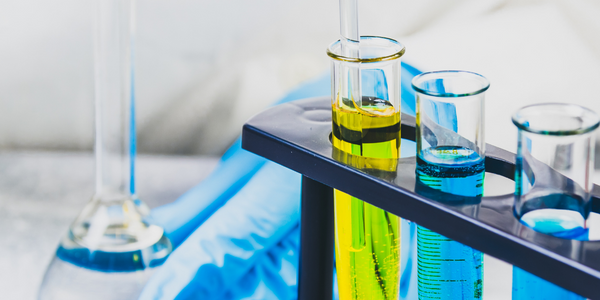
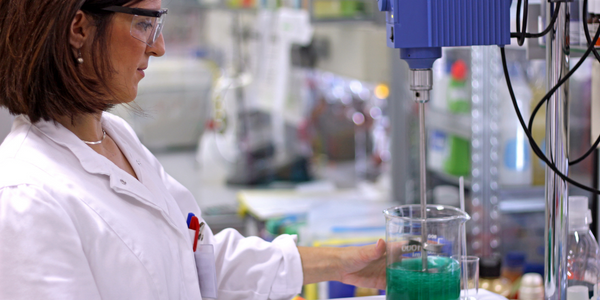