Technology Category
- Analytics & Modeling - Big Data Analytics
- Functional Applications - Enterprise Asset Management Systems (EAM)
Applicable Industries
- Buildings
- Retail
Applicable Functions
- Procurement
- Product Research & Development
Use Cases
- Asset Health Management (AHM)
- Time Sensitive Networking
Services
- Data Science Services
- Testing & Certification
About The Customer
Hodges Ward Elliott is a commercial real estate investment company that is at the forefront of leveraging data science in the real estate industry. Recognizing the potential of data science in understanding and predicting trends in real estate, the company has been proactive in adopting new technologies and methodologies to gain a competitive edge. The company believes that understanding the preferences of groups of people and how these preferences change over time is key to profiting in real estate. To this end, they have been using open data and the open source programming language R to analyze data and identify trends and patterns. They have also been using predictive modeling to predict the probability of a building selling in any given year in New York City.
The Challenge
Hodges Ward Elliott, a commercial real estate investment company, was looking to leverage data science to gain a competitive edge in the real estate market. The company recognized the potential of data science in real estate, an industry that has traditionally been slow to adopt such technologies. The challenge was to find a way to effectively use data science to understand the preferences of groups of people and how these preferences change over time. This understanding is crucial to predicting trends in real estate, as it can provide insights into where people want to live, shop, and work. However, obtaining and analyzing relevant data was a significant challenge due to the lack of readily available data in the real estate industry.
The Solution
The company turned to open data, a movement by governments around the world to make all of their government agency data available online. This provided a wealth of data that was previously unavailable for analysis, especially within urban markets. The company used the open source programming language R to analyze this data and identify trends and patterns. They used various data sources, including taxi traffic, city bikes, bank deposit data, new build permits, and more to understand people's preferences and how they change over time. They also used predictive modeling to predict the probability of a building selling in any given year in New York City. This model was based on NYC tax lot data and NYC sales data, and used random forest modeling for its speed and accuracy. The company also used spatial lag, a technique that summarizes data points around a point, to improve the predictive accuracy of their model.
Operational Impact
Quantitative Benefit
Case Study missing?
Start adding your own!
Register with your work email and create a new case study profile for your business.
Related Case Studies.
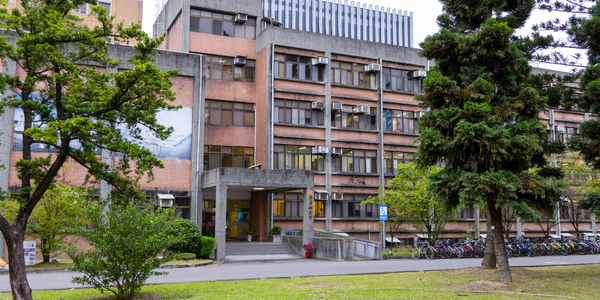
Case Study
Energy Saving & Power Monitoring System
Recently a university in Taiwan was experiencing dramatic power usage increases due to its growing number of campus buildings and students. Aiming to analyze their power consumption and increase their power efficiency across 52 buildings, the university wanted to build a power management system utilizing web-based hardware and software. With these goals in mind, they contacted Advantech to help them develop their system and provide them with the means to save energy in the years to come.
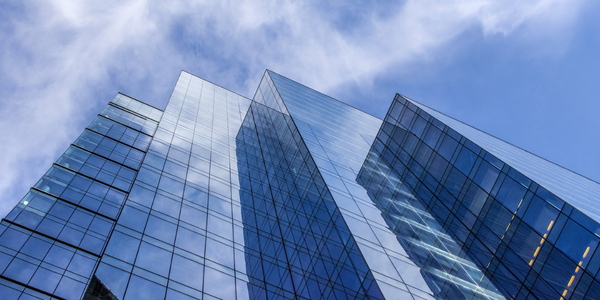
Case Study
Intelligent Building Automation System and Energy Saving Solution
One of the most difficult problems facing the world is conserving energy in buildings. However, it is not easy to have a cost-effective solution to reduce energy usage in a building. One solution for saving energy is to implement an intelligent building automation system (BAS) which can be controlled according to its schedule. In Indonesia a large university with a five floor building and 22 classrooms wanted to save the amount of energy being used.
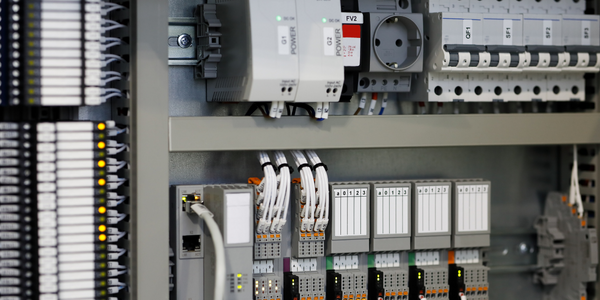
Case Study
Improving Production Line Efficiency with Ethernet Micro RTU Controller
Moxa was asked to provide a connectivity solution for one of the world's leading cosmetics companies. This multinational corporation, with retail presence in 130 countries, 23 global braches, and over 66,000 employees, sought to improve the efficiency of their production process by migrating from manual monitoring to an automatic productivity monitoring system. The production line was being monitored by ABB Real-TPI, a factory information system that offers data collection and analysis to improve plant efficiency. Due to software limitations, the customer needed an OPC server and a corresponding I/O solution to collect data from additional sensor devices for the Real-TPI system. The goal is to enable the factory information system to more thoroughly collect data from every corner of the production line. This will improve its ability to measure Overall Equipment Effectiveness (OEE) and translate into increased production efficiencies. System Requirements • Instant status updates while still consuming minimal bandwidth to relieve strain on limited factory networks • Interoperable with ABB Real-TPI • Small form factor appropriate for deployment where space is scarce • Remote software management and configuration to simplify operations
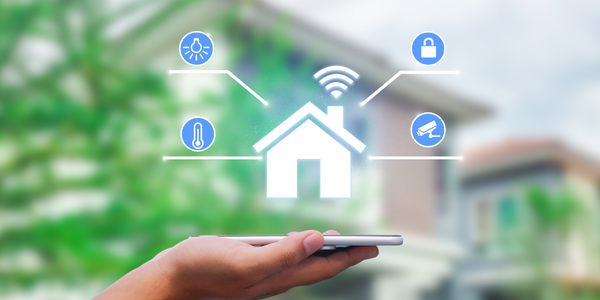
Case Study
Powering Smart Home Automation solutions with IoT for Energy conservation
Many industry leaders that offer Smart Energy Management products & solutions face challenges including:How to build a scalable platform that can automatically scale-up to on-board ‘n’ number of Smart home devicesData security, solution availability, and reliability are the other critical factors to deal withHow to create a robust common IoT platform that handles any kind of smart devicesHow to enable data management capabilities that would help in intelligent decision-making