Customer Company Size
Large Corporate
Region
- Asia
Country
- India
Product
- Blue Yonder Luminate Platform
Tech Stack
- Machine Learning
- Artificial Intelligence
Implementation Scale
- Enterprise-wide Deployment
Impact Metrics
- Cost Savings
- Productivity Improvements
Technology Category
- Platform as a Service (PaaS) - Data Management Platforms
- Analytics & Modeling - Machine Learning
Applicable Industries
- Automotive
Applicable Functions
- Logistics & Transportation
Use Cases
- Inventory Management
- Supply Chain Visibility
Services
- Data Science Services
About The Customer
Mahindra & Mahindra Farm Equipment is part of the $20 billion Mahindra Group. It is the world’s number-one tractor company by volume, and its automotive business competes in almost every segment of the industry. The Spares Business Unit (SBU) provides genuine vehicle and tractor spare parts via advanced capabilities in sourcing, assembling, warehousing and distribution. The company constantly evaluates scientific methods to tweak demand forecasting, inventory management and replenishment planning strategies to ensure that the right parts are available at the right place and time.
The Challenge
Mahindra & Mahindra Farm Equipment, part of the $20 billion Mahindra Group, is the world’s number-one tractor company by volume. Its automotive business competes in almost every segment of the industry. The Spares Business Unit (SBU) provides genuine vehicle and tractor spare parts via advanced capabilities in sourcing, assembling, warehousing and distribution. To maximize supply chain efficiencies and service, Mahindra & Mahindra constantly evaluates scientific methods to tweak demand forecasting, inventory management and replenishment planning strategies to ensure that the right parts are available at the right place and time. However, their traditional, manually driven segmentation processes and tools often resulted in inefficient allocation, high safety inventory levels and less-than-optimal service levels.
The Solution
Mahindra & Mahindra SBU leverages Blue Yonder Luminate Platform, which applies machine learning (ML) to both systematically classify spare parts into unique demand clusters and establish the significance of demand attributes. The result is built-in visibility, intelligence, context and collaboration tools that enable increased allocation efficiency and profitability. Via optimization techniques, powered by ML, service levels are assigned to demand clusters, with the goal of minimizing safety inventory costs. In addition, ML and artificial intelligence algorithms accurately estimate lead times and further optimize the cost-to-serve from both a customer service and inventory cost perspective. Instead of making manual decisions, the SBU can analyze data at scale, identify critical demand variables and autonomously optimize the supply chain for specified profitability goals.
Operational Impact
Quantitative Benefit
Case Study missing?
Start adding your own!
Register with your work email and create a new case study profile for your business.
Related Case Studies.
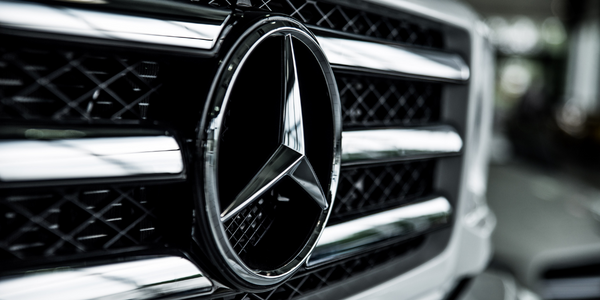
Case Study
Integral Plant Maintenance
Mercedes-Benz and his partner GAZ chose Siemens to be its maintenance partner at a new engine plant in Yaroslavl, Russia. The new plant offers a capacity to manufacture diesel engines for the Russian market, for locally produced Sprinter Classic. In addition to engines for the local market, the Yaroslavl plant will also produce spare parts. Mercedes-Benz Russia and his partner needed a service partner in order to ensure the operation of these lines in a maintenance partnership arrangement. The challenges included coordinating the entire maintenance management operation, in particular inspections, corrective and predictive maintenance activities, and the optimizing spare parts management. Siemens developed a customized maintenance solution that includes all electronic and mechanical maintenance activities (Integral Plant Maintenance).
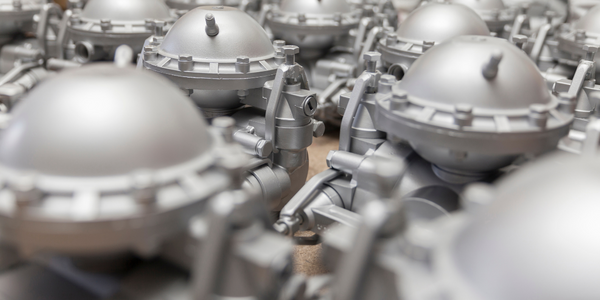
Case Study
Monitoring of Pressure Pumps in Automotive Industry
A large German/American producer of auto parts uses high-pressure pumps to deburr machined parts as a part of its production and quality check process. They decided to monitor these pumps to make sure they work properly and that they can see any indications leading to a potential failure before it affects their process.