Customer Company Size
Large Corporate
Country
- Worldwide
Product
- SendinBlue
- Dataiku Data Science Studio (DSS)
Tech Stack
- MongoDB
- MySQL
- Redshift
- Python
Implementation Scale
- Enterprise-wide Deployment
Impact Metrics
- Productivity Improvements
- Customer Satisfaction
Technology Category
- Analytics & Modeling - Predictive Analytics
Applicable Functions
- Sales & Marketing
Use Cases
- Fraud Detection
- Predictive Quality Analytics
Services
- Data Science Services
About The Customer
SendinBlue is a relationship marketing SaaS solution that was launched in 2012. The company's mission is to become the simplest, most reliable, and cost-effective marketing platform. SendinBlue now powers marketing campaigns for more than 50,000 companies around the world. With offices in North America, Europe, and Asia, the SendinBlue team supports the product in six languages. Their platform integrates with the top e-commerce and CMS tools, and their system delivers over 30 million emails and text messages per day.
The Challenge
SendinBlue, a relationship marketing SaaS solution, faced a significant challenge in validating new customers and ensuring the quality of their databases. The company had to ensure that all contacts on the list were opted in, which required manual validation. This process was not only time-consuming and required a large workforce, but it also severely delayed account validation for customers, damaging SendinBlue’s reputation. As the customer base grew, manual validation became increasingly unfeasible. The company needed a solution that could automate the validation process and scale with the growing demand.
The Solution
SendinBlue turned to Dataiku Data Science Studio (DSS) to develop an automated fraud detection system. Using historical data from over 1 billion emails and associated events, thousands of blocked accounts, and hundreds of fraud criteria, SendinBlue built a scalable solution. The new system analyzes new customers and automatically classifies them as 'good,' 'bad,' or 'uncertain.' An algorithm then determines the customer’s credibility by taking into account sending volume, the scoring of the contacts, etc. Depending on the customer’s risk score, they may be blocked, validated, or sent to customer care for manual analysis. Dataiku was instrumental in deploying the data product into production, handling large amounts of different datasets, and designing, testing, and developing the solution in less than three months.
Operational Impact
Quantitative Benefit
Case Study missing?
Start adding your own!
Register with your work email and create a new case study profile for your business.
Related Case Studies.
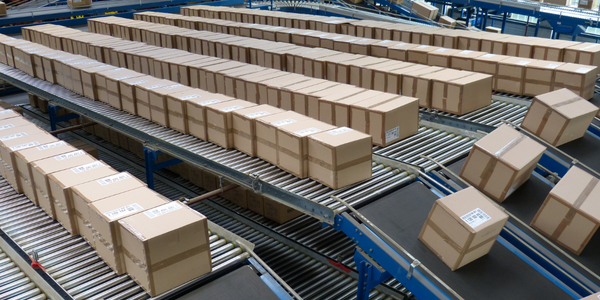
Case Study
IoT Data Analytics Case Study - Packaging Films Manufacturer
The company manufactures packaging films on made to order or configure to order basis. Every order has a different set of requirements from the product characteristics perspective and hence requires machine’s settings to be adjusted accordingly. If the film quality does not meet the required standards, the degraded quality impacts customer delivery causes customer dissatisfaction and results in lower margins. The biggest challenge was to identify the real root cause and devise a remedy for that.
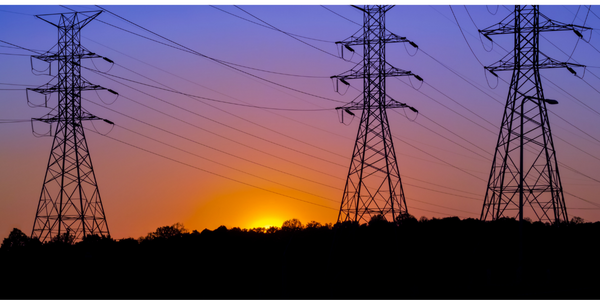
Case Study
Largest Production Deployment of AI and IoT Applications
To increase efficiency, develop new services, and spread a digital culture across the organization, Enel is executing an enterprise-wide digitalization strategy. Central to achieving the Fortune 100 company’s goals is the large-scale deployment of the C3 AI Suite and applications. Enel operates the world’s largest enterprise IoT system with 20 million smart meters across Italy and Spain.
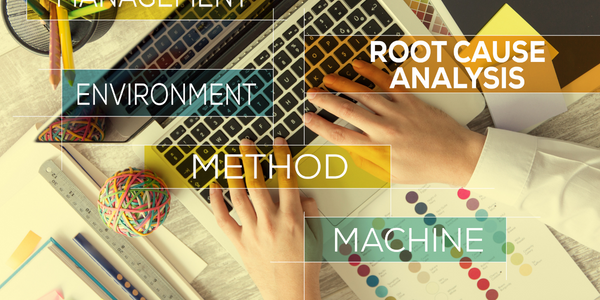
Case Study
Prevent Process Inefficiencies with Automated Root Cause Analysis
Manufacturers mostly rely on on-site expert knowledge for root cause analysis. When the defective product is sent to lab for analysis, it is laborious and always a post-mortem one. Manufacturers that collect data from IT and OT also need a comprehensive understanding of a variety of professionals to make sense of it. This is not only time consuming, but also inefficiencient.
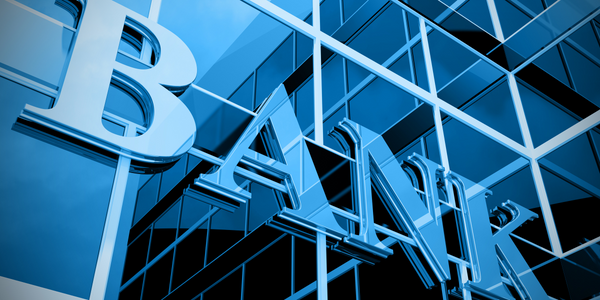
Case Study
KeyBank's Digital Transformation with Confluent's Data in Motion
KeyBank, one of the nation's largest bank-based financial services companies, embarked on a national digital bank initiative following the acquisition of Laurel Road, a digital consumer lending business. The initiative aimed to build a digital bank focused on healthcare professionals looking to refinance student loans and buy homes. A significant challenge was reducing the time to market for new products by democratizing data and decoupling systems across the IT landscape. Like many large enterprises, KeyBank had a variety of vendor applications, custom applications, and other systems that were tightly coupled to one another. New projects often required developing specific point-to-point integrations for exchanging data, which did not address the needs of other downstream systems that could benefit from the same data.
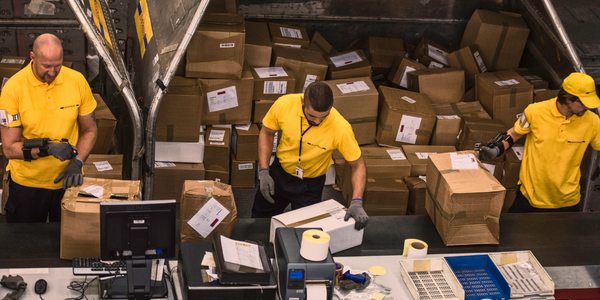
Case Study
Digitization of Pharmaceutical Packaging Machines: A Case Study of CVC Technologies
CVC Technologies, a leading manufacturer of pharmaceutical packaging machines, was seeking an end-to-end IoT solution to fully digitize their pharmaceutical liquid filling and capping machines. The company aimed to enhance the safety of their equipment, introduce digital maintenance capabilities, and gain visibility into machine status from anywhere at any time. The challenge was to find a solution that could provide real-time visibility into the machine's status, deliver direct cloud connectivity and digital services, and simplify all aspects of the machine's lifecycle, from engineering to maintenance.
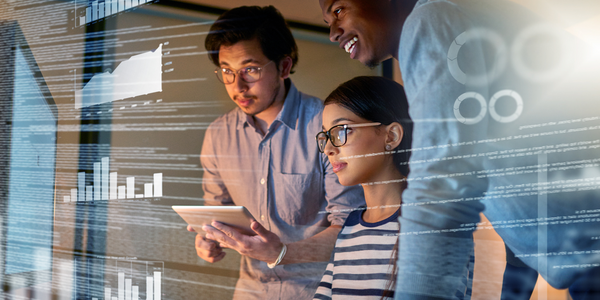
Case Study
CleanTelligent Enhances Janitorial Software Solutions with Infor Birst
CleanTelligent Software, a company that aids in-house and contracted janitorial teams in streamlining communication and improving quality control, faced a significant challenge. Their clients were demanding a more dynamic way to present reporting data. The company's software was primarily used to analyze and summarize a custodial team's performance, replacing a highly manual, paper-driven process. However, the initial differences between service providers in the janitorial industry are often unclear, and the cost of switching is comparatively low. This situation led to high client turnover, with a janitorial company's customer lifetime averaging four years or less. CleanTelligent needed to improve the customer experience with dynamic dashboards and reporting, retain customers through predictive analysis, capitalize on advanced analytics capabilities to build market differentiation, and improve client retention rates.