Technology Category
- Analytics & Modeling - Computer Vision Software
- Functional Applications - Inventory Management Systems
Applicable Industries
- Food & Beverage
Applicable Functions
- Warehouse & Inventory Management
Use Cases
- Computer Vision
- Object Detection
About The Customer
CellarEye, Inc. is a company that is dedicated to revolutionizing the experience of managing private and professional wine collections. They aim to make wine more accessible to more people by providing a better and more widespread understanding of wine. To achieve this, they leverage state-of-the-art Computer Vision (CV) and Artificial Intelligence (AI) technologies to offer a seamless management system. This system automatically tracks each wine bottle in a cellar, storing both the brand and location into inventory tools without the need for manual entries. The company is committed to overcoming the challenges presented by the complex cellar environment and the numerous edge cases that arise from managing thousands of wine bottles.
The Challenge
CellarEye, Inc. is a company that aims to revolutionize the management of private and professional wine collections by leveraging state-of-the-art Computer Vision (CV) and Artificial Intelligence (AI) technologies. Their goal is to provide a seamless management system that automatically tracks each wine bottle in a cellar, storing both the brand and location into inventory tools without manual entries. However, the team at CellarEye faced a significant challenge in realizing their vision. They needed to develop a reliable object detection model to recognize and track wine bottles as they were registered to and removed from the inventory. The cellar environment, with its thousands of wine bottles, presented a complex scenario with numerous edge cases. The company initially struggled with bad or inconsistent annotations, which made achieving an accuracy rate of over 80% a challenge. They needed a better way to detect problems with their data, understand their model failures, and enable their Machine Learning (ML) team to collaborate with their annotation team to catch labeling mistakes faster.
The Solution
To overcome their challenges, the CellarEye team turned to Scale Nucleus. This tool allowed them to identify where their models were underperforming and where labels were likely to be incorrect. With Nucleus, CellarEye’s team could query their labeled and unlabeled data on custom metadata, visualize model predictions alongside ground truth annotations, and sort results by error metrics like Intersection Over Union (IoU). They could also look at high-confidence false positive predictions, and fix and export corrected labels using Nucleus’s built-in annotation editor. This solution provided a more efficient and accurate way to manage the complex task of tracking and managing the wine bottles in a cellar. By integrating Scale Nucleus into their system, CellarEye was able to significantly improve the accuracy and reliability of their object detection model, thereby enhancing their cellar management solution.
Case Study missing?
Start adding your own!
Register with your work email and create a new case study profile for your business.
Related Case Studies.
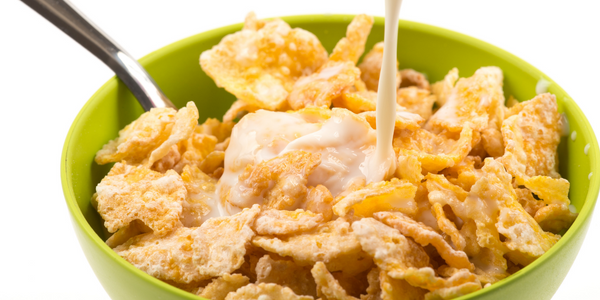
Case Study
The Kellogg Company
Kellogg keeps a close eye on its trade spend, analyzing large volumes of data and running complex simulations to predict which promotional activities will be the most effective. Kellogg needed to decrease the trade spend but its traditional relational database on premises could not keep up with the pace of demand.
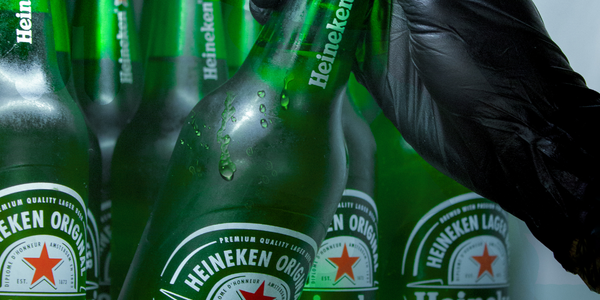
Case Study
HEINEKEN Uses the Cloud to Reach 10.5 Million Consumers
For 2012 campaign, the Bond promotion, it planned to launch the campaign at the same time everywhere on the planet. That created unprecedented challenges for HEINEKEN—nowhere more so than in its technology operation. The primary digital content for the campaign was a 100-megabyte movie that had to play flawlessly for millions of viewers worldwide. After all, Bond never fails. No one was going to tolerate a technology failure that might bruise his brand.Previously, HEINEKEN had supported digital media at its outsourced datacenter. But that datacenter lacked the computing resources HEINEKEN needed, and building them—especially to support peak traffic that would total millions of simultaneous hits—would have been both time-consuming and expensive. Nor would it have provided the geographic reach that HEINEKEN needed to minimize latency worldwide.
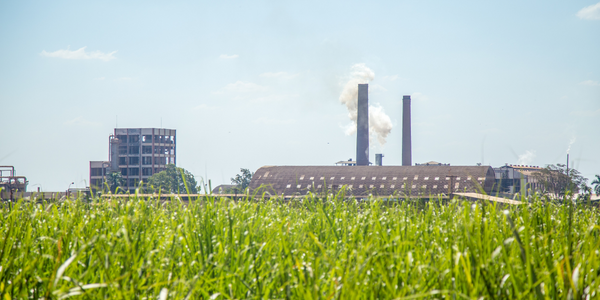
Case Study
Energy Management System at Sugar Industry
The company wanted to use the information from the system to claim under the renewable energy certificate scheme. The benefit to the company under the renewable energy certificates is Rs 75 million a year. To enable the above, an end-to-end solution for load monitoring, consumption monitoring, online data monitoring, automatic meter data acquisition which can be exported to SAP and other applications is required.
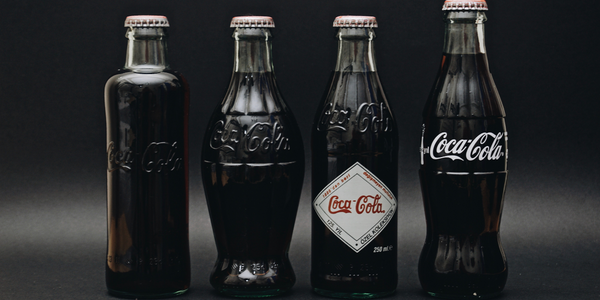
Case Study
Coca Cola Swaziland Conco Case Study
Coco Cola Swaziland, South Africa would like to find a solution that would enable the following results: - Reduce energy consumption by 20% in one year. - Formulate a series of strategic initiatives that would enlist the commitment of corporate management and create employee awareness while helping meet departmental targets and investing in tools that assist with energy management. - Formulate a series of tactical initiatives that would optimize energy usage on the shop floor. These would include charging forklifts and running cold rooms only during off-peak periods, running the dust extractors only during working hours and basing lights and air-conditioning on someone’s presence. - Increase visibility into the factory and other processes. - Enable limited, non-intrusive control functions for certain processes.
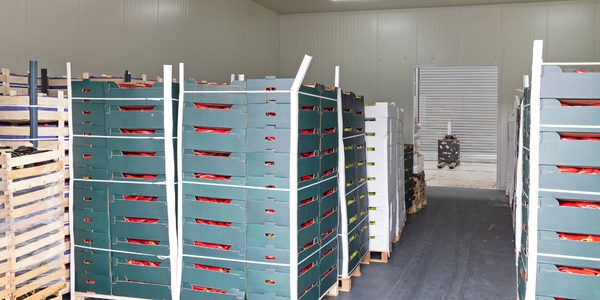
Case Study
Temperature Monitoring for Restaurant Food Storage
When it came to implementing a solution, Mr. Nesbitt had an idea of what functionality that he wanted. Although not mandated by Health Canada, Mr. Nesbitt wanted to ensure quality control issues met the highest possible standards as part of his commitment to top-of-class food services. This wish list included an easy-to use temperature-monitoring system that could provide a visible display of the temperatures of all of his refrigerators and freezers, including historical information so that he could review the performance of his equipment. It also had to provide alert notification (but email alerts and SMS text message alerts) to alert key staff in the event that a cooling system was exceeding pre-set warning limits.
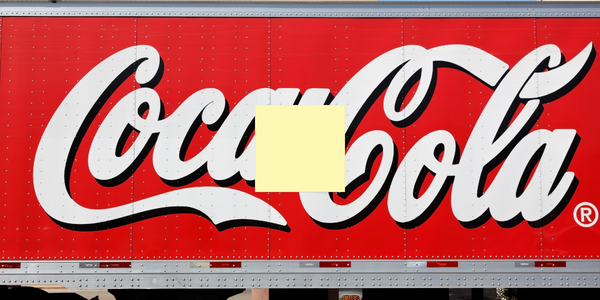
Case Study
Coca-Cola Refreshments, U.S.
Coca-Cola Refreshments owns and manages Coca-Cola branded refrigerators in retail establishments. Legacy systems were used to locate equipment information by logging onto multiple servers which took up to 8 hours to update information on 30-40 units. The company had no overall visibility into equipment status or maintenance history.