H2O.ai
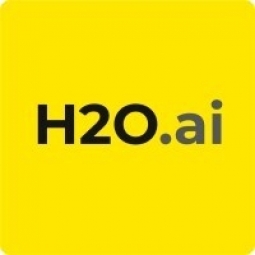
Overview
HQ Location
United States
|
Year Founded
2012
|
Company Type
Private
|
Revenue
$10-100m
|
Employees
201 - 1,000
|
Website
|
Twitter Handle
|
Company Description
H2O.ai is the leading open source Generative AI and Machine Learning platform provider on a mission to democratize AI. It distills the technical prowess of 30 Kaggle Masters into straightforward AI cloud products for Generative AI and Machine Learning that solve powerful problems. Customers, community, and partners are strategic investors in H2O.ai building a long-term vision for using AI for Good.
Supplier missing?
Start adding your own!
Register with your work email and create a new supplier profile for your business.
Case Studies.
Case Study
Nationwide Insurance Delivers Exceptional Protection for Members with H2O.ai
Data is everywhere at Nationwide and the company wanted more from the data they had to find new ways to provide more personalized service and response to their members. To achieve this goal, Nationwide needed an AI platform that allowed them the flexibility to easily work in a complex data environment to rapidly explore data and quickly prototype new models.
Case Study
H2O for Real Time Fraud Detection
Organizations responsible for fraud prevention are facing a host of challenges at the transaction, account, and network-level to detect fraudulent behavior and suspicious activities. Fraudulent transactions are rare, but costly if they aren’t detected. In the credit card business, for example, third-party fraud accounts for roughly 4 out of every 10,000 transactions. Modeling rare events is difficult, like finding a needle in a haystack. For best results, gather as much data as possible, and use the most advanced techniques available.
Case Study
AES's Transformation in Energy Business with AI and H2O.ai
AES, a leading renewable-energy company, was faced with the challenge of accelerating their transition to renewable energy at scale. This business transformation necessitated a digital and AI transformation to better predict and optimize the energy output from renewable sources, predict failures, and optimize load distribution. The company had to deal with the complexities of wind-turbine predictive maintenance, energy bidding strategy for hydroelectric power plants, and smart meters. The maintenance of wind turbines, which have numerous moving parts subjected to harsh environments, was particularly costly and time-consuming. The company also needed to optimize its energy bidding strategy to maximize revenue from its hydroelectric power plants. Additionally, the company had to manage over one million smart meters, which sometimes had maintenance issues or were subject to misuse.
Case Study
CBA boosts the bank’s AI capabilities to generate better customer and community outcomes, at greater pace and scale
Commonwealth Bank was looking to enhance its AI capabilities to provide more personalized and relevant experiences for its customers. The bank wanted to decrease the time to prototype new models from weeks to just 2 days. The bank was also looking to scale machine learning across the entire organization, including data scientists and business users. The bank was seeking a solution that would allow it to better help customers find personalized and relevant offers to save money while they shop across platforms like Little Birdie, Karta, CommBank Rewards, and Klarna, while at the same time driving sales for merchants.
Case Study
Predictive Model Factory
Cisco, a multinational technology company, faced challenges in building models to deliver accurate predictions about customer propensity to buy across its extensive product portfolio. The company was struggling with speed and scalability challenges associated with analyzing an exploding amount of information about buying patterns. The company had to recreate all its predictive models from scratch every quarter, a process that took more than four weeks. To avoid even longer processing times, models were trained on relatively small samples that were rarely larger than 100,000 cases. The company was limited to standard techniques and was unable to test competing algorithms such as ensembles, grid search, and deep learning.
Case Study
H2O.ai empowers New South Wales Government To Deliver Exceptional Services for its Citizens with AI
The New South Wales (NSW) Government wanted to build out its data practice and initiatives. They needed to enable its analysts to draw upon data science and automatic machine learning platforms to help find answers, pinpoint solutions and use data to create better services for all. The government was looking for a solution that could improve the accuracy of its predictive models and empower its team of data scientists to build models faster.
Case Study
AI Improves Profitability at Paraguay Bank
Visión Banco, based in Asunción, Paraguay, provides financial services to small and micro-sized companies in its home country. The bank was looking to expand its services and offers to customers, easily determine credit risks, and do so with accuracy and speed. It also wanted to enhance its practices by implementing predictive analytics, such as to predict customer payment default or churn. However, the bank was facing challenges in scaling these operations without a new tool or plan. The data science team first hired an external consultant who developed a model using IBM SPSS Software, a process that took a year. Then the team started using open source tools R, H2O, and Openscoring.io, which allowed the data scientists to deploy models in Predictive Model Markup Language (PMML) format—an industry standard for data models. Yet predictive analytics were still taking considerable time and effort.
Case Study
Solving Customer Churn with Machine Learning
Paypal, a global payments platform, was facing a significant challenge with customer churn. The company's previous approach to identifying churn was based on specific time increments, marking a customer as churned if they hadn't used the platform within that period. However, this method was not fully accurate and impacted the effectiveness of Paypal's marketing efforts to win back customers. The company needed a more precise way to predict if and when a customer would churn and the reasons behind it. This information was crucial for the operational teams to develop new programs aimed at customer retention.
Case Study
H20.ai for Payment Fraud
Fraud is a significant problem in financial services, with fraudsters constantly changing their tactics. Fraud detection is a balance between enabling spending, stopping fraud, and closing the loop with customers. However, new data sources from mobile apps and third-party providers can help improve fraud detection, but the increase in data size and variety creates new issues for fraud and risk management teams. These teams are already struggling with rules and statistical modeling systems and limited resources. Fraud cost US financial institutions almost $10 billion in 2018 and is a key issue for regulators. Customer acquisition and retention, however, is top of mind for many bank executives with new competitors from fintech startups to technology companies jumping into payments. Fraud and resulting customer churn can significantly impact profitability, customer trust, and regulatory compliance.
Case Study
Capital One Uses H2O for Mobile Transaction Forecasting and Anomaly Detection
Capital One's mobile app is a popular platform for customers to perform transactions, with up to 5,000 customers logging in every minute. This high volume of usage means that even small outages need to be identified and resolved quickly to prevent service disruptions. The bank's technology operations group monitors all critical systems and platforms and sets up alerts based on company policies. However, setting up alerts for volume anomalies, such as a drop or spike in transaction volume, proved to be a challenge. Traditional methods of calculating volume anomalies were not scalable and required a lot of coding, development, and oversight to manage.
Case Study
Operationalizing Machine Learning at Comcast
Comcast, a multinational mass media company, was facing challenges in building models on complete production datasets to improve the accuracy of their predictive analytics. They needed a solution to run models on complete production datasets as opposed to relying on sampling. The company was also looking for ways to operationalize and scale data science to match the company's volume of operations. They were dealing with issues such as extracting and integrating real-time production data that comes in different formats, using heavy computation to transform raw data into usable data sources, providing timely responses to a great number of prediction requests and continuously updating models with the latest data to keep predictions accurate.
Case Study
AI Helps Property Management Company Maximize Their Business
Property Guru, a leading property management company based in Singapore, handles a large volume of listings and had looked to leverage AI and machine learning (ML) for multiple use-cases - image moderation, predicting churn, forecasting credit, measuring performance of listings. They realized early-on in their development that they needed machine learning techniques to manage user data, user retention and ensure the customer experience on their app lives up to their reputation. Doing this manually was not scaling so there was a real need to automate their ML process.
Case Study
Acordo Certo Reduces Consumer Debt in Brazil with H2O.ai
Consumer debt was rising in Brazil, and it was becoming challenging for both consumers and debtors to find solutions. The target market of debtors and the ensuing volume of data to be analyzed were both large and therefore, challenging. Acordo Certo had a small team of data scientists, who were using traditional methods to collect and analyze data, as well as to build and deploy predictive and scored models. However, due to the large database of customers, there was a need to increase the agility of model development and accuracy, as well as improve overall trust in AI by making the results of machine learning algorithms transparent to business partners, such as retailers and banks.
Case Study
Machine Learning to Save Lives
Kaiser Permanente, an integrated healthcare system, was facing a challenge with patients who undergo an unplanned transfer to the Intensive Care Unit (ICU). These patients experienced significantly worse outcomes than those directly admitted to the ICU. They represented about a fourth of all Kaiser ICU admissions, a fifth of all deaths in the hospital, and about an eighth of all of the hospital days. The patients who experienced an unplanned transfer to the ICU experienced two to five times the mortality of patients who are directly admitted to the ICU, and they would stay in the hospital an average of 8 to 12 days more than patients who are directly admitted to the ICU. The challenge was to identify these patients ahead of time who are likely to crash and be rushed to the ICU, and intervene before the deterioration.
Case Study
Hortifrut Optimizes Distribution of Blueberries with AI
Transporting fruit from the farm may take weeks, so Hortifrut had to predict the quality of produce upon arrival. Not being able to do this accurately can impact customer experience and revenue loss. But getting such predictions accurately can be a difficult task given the complexity of the distribution channel, weather data, variety of datasets, shipping times and more. If traditional machine learning methods and toolkits were used, it could easily take months to build accurate predictions that can be reliably deployed. This may also require hiring additional data science talent on the team, hence requiring additional time and budget to achieve the aforementioned business goal.
Case Study
Shipment Volume Forecasts Powered by AI at Senko Group
Senko Group Holdings, a large integrated logistics service provider, was facing challenges in manpower planning due to the shortage of workers in Japan. The company needed to maintain a high level of customer service despite the inability to cut operational tasks. The logistics staff was burdened with the task of predicting shipment volumes from their warehouses, a task that was time-consuming and complex. The company initially tried using R and IBM SPSS for AI-based shipment volume forecasts, but found them challenging for the logistics staff to use for actual operations. The structure of the model was complicated and required a great deal of effort for creating models as well as for applying feature engineering.
Case Study
Increasing Effectiveness of Real Estate Marketing with H2O.ai
G5, Inc., a leading marketing optimization company for the real estate industry, was facing a challenge with its lead generation process. The company found that only 14% of its call leads were productive, resulting in low job satisfaction, high turnover for leasing agents, and low conversion numbers. G5 wanted to solve this by using machine learning to identify stronger leads that would more likely result in sales. However, the company didn’t have dedicated data science resources to create the needed machine learning models. The implementation of machine learning could prove to be time consuming, expensive, and a barrier to innovation.
Case Study
MarketShare Enhances Its Marketing Analytics Services Using H2O
Cross-channel attribution, revenue optimization, and forecasting are among the biggest pain points in brand marketing today. Marketing teams need to see a complete picture of their effectiveness but are often limited to partial data in spreadsheets that take months to analyze. As a result, CMOs and analysts often throw darts in the dark when forecasting the outcome of their marketing investments - severely restricting an organization's ability to reach more customers and grow. Organizations are often buried under an avalanche of data, which is often collected on an automated basis, as a byproduct of everyday operations. The process of moving from 'insights' to 'prescriptive action,' as a result, becomes a major challenge.
Case Study
AIMIA Transforms Customer Loyalty with AI
AIMIA, a global leader in customer engagement and loyalty solutions, faced challenges in predicting customer churn and detecting fraud due to the lack of relevant datasets and the steady evolution of fraudulent behavior. The development of machine learning techniques in the face of these challenges was difficult. There was a need to increase the agility of model development, build newer use-cases quickly, iterate on them faster, improve overall trust in AI by making the results of machine learning algorithms transparent to business stakeholders, as well as benchmark the performance of the models already in production.
Case Study
AI Helps Match Patients with Specialists to Improve Health Outcomes
Finding the right specialist is the first step to receiving the right care. However, consumers are not equipped to navigate the complex and confusing healthcare system. It can be challenging for patients to discover which specialist they should approach for different health situations and, even with a referral from a primary physician, it can still be a long process until they find the right specialist who can accurately treat them while also providing a satisfactory patient experience. Finding the right match between patient and doctor can solve major problems and save lives.
Case Study
H2O.ai Empowers MarketAxess to Innovate in Capital Markets
The lack of liquidity in bond markets has traditionally made predicting bond prices challenging. In order to provide higher transparency and find the most consistent and accurate prices to help traders become more confident as price makers, MarketAxess relied on machine learning to develop Composite+, a proprietary algorithmic pricing engine for corporate bonds that leverages a range of proprietary and industry data sources, with updates up to every 15 seconds.
Case Study
AI Improves Overall Business Relationships for Jewelers Mutual
Jewelers Mutual, a leading provider of insurance for jewelers and consumers in the United States and Canada, recognized the need to invest in analytics, AI, and machine learning to improve overall customer experiences. Their business relies on effectively protecting their jeweler customers’ businesses and providing personal insurance directly to consumers with innovative customer experiences. They collected data from losses, customers, and multiple other sources which weren’t tapped into before. They started their AI journey a few years ago by implementing Gradient Boosting Machine, and then moving to an AutoML solution from DataRobot. However, they realized that they needed greater transparency of a solution and needed to have an explainable AI component.
Case Study
More Accurate, Real-time Risk Score with Fast Time-to-Market
Airvantage, a South African cellular telecommunication provider, was facing a challenge with its Prepaid Airtime Advance System (PAS). The system, which advances airtime, data, or mobile money to subscribers, was using a series of static business rules to make airtime advance decisions. However, these rules were overly cautious, leading to a high number of false negatives - refusing credit to desirable customers. Airvantage needed a solution that would allow them to accurately assess the risk of advancing credit to their subscribers, without unnecessarily declining potential customers. They sought a data science toolkit that could help them build a more accurate risk model, and after evaluating over 30 toolkits and platforms, they decided to use H2O Driverless AI.
Case Study
Leveraging Large Scale Data Sets
The insurance company was facing a significant challenge with claims fraud, which is estimated to cost the industry $80 billion annually in the United States alone. The existing process for detecting suspicious claims was entirely manual, relying on the judgment and experience of professional claims examiners. This approach was not scalable for a growing business and was time-consuming due to the need to pull information from multiple systems. The company had consolidated data from various sources into a Hadoop data store, which included a mix of structured and unstructured data. However, Hadoop lacked the capability for sophisticated predictive analytics, and extracting the data to an analytic server was time-consuming.