Customer Company Size
SME
Region
- America
- Asia
Country
- United States
Product
- Domo BI & Analytics
Tech Stack
- Shopify
Implementation Scale
- Enterprise-wide Deployment
Impact Metrics
- Productivity Improvements
- Revenue Growth
Technology Category
- Analytics & Modeling - Real Time Analytics
Applicable Functions
- Sales & Marketing
- Procurement
Use Cases
- Predictive Replenishment
- Demand Planning & Forecasting
Services
- Data Science Services
About The Customer
Cozy Earth is a company that specializes in creating sleep products from premium 100% viscose from bamboo fabric. The company's sheets, comforters, and pajamas are designed to help sleepers regulate their temperature throughout the night. Cozy Earth's products have been featured on Oprah’s Favorite Things list four years in a row, and the company has grown 20x over the past three years. Cozy Earth sources all of its raw materials and final products from Asia, and the company must forecast demand as far as nine months in advance of when the final product is needed in stores.
The Challenge
Cozy Earth, a company that manufactures sleep products from bamboo, faced challenges in managing its supply chain and forecasting demand. The company's raw materials and final products are supplied from Asia, requiring precise forecasting up to nine months in advance. Over-ordering could tie up valuable cash in unsold products, while under-ordering could result in missed sales opportunities. Prior to implementing Domo, gathering data to inform forecasting was a complex, manual process that involved downloading reports from each separate channel, copying and pasting data into a spreadsheet, and spending hours working with the data before it was ready for use by decision-makers.
The Solution
Cozy Earth implemented Domo's BI & Analytics solution to leverage data in managing and optimizing its growth. Domo allows Cozy Earth to automatically collect, clean, and combine data from Shopify and third-party sales channels, marketing channels like Facebook and Google, and the supply chain platforms that power its warehouse and delivery systems. This data is made available to every department, allowing all business users to access the data they need, whenever they need it, without having to wait days for a report. In addition to helping the company be smart about how it orders products, Cozy Earth also uses Domo to make intelligent decisions about which social channels to prioritize and which products to feature in its digital marketing.
Operational Impact
Quantitative Benefit
Case Study missing?
Start adding your own!
Register with your work email and create a new case study profile for your business.
Related Case Studies.
Case Study
Pepsico's Transformation to Smarter Sales Forecasting with Designer Cloud
PepsiCo, a global consumer packaged goods company, faced a significant challenge in calibrating sales forecasting to supply the right product quantities to its retailers. The sales forecast incorporated a variety of data, including warehouse data, store stock data, and promotional forecast data, all of which were provided by retailers in different file formats and delivered using various methods. The primary challenge was the speed of preparing a sales forecast. With the existing Microsoft Access and Excel-based processes, the time required to prepare this data was so extensive that analysts could only leverage it once a month or not at all. This inefficiency risked under or oversupplying retailers, potentially impacting PepsiCo's business operations and customer relationships.
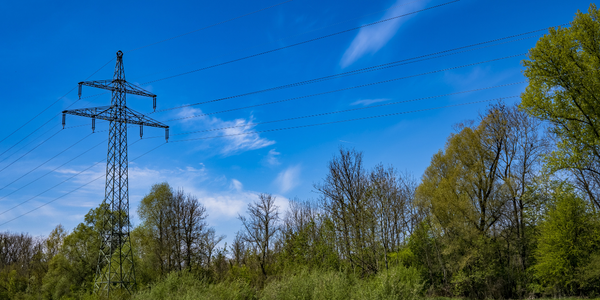
Case Study
Gexa Energy and AutoGrid's Innovative Demand Response Programs in ERCOT
Gexa Energy, a leading retail electricity provider in Texas, was seeking to introduce new demand response programs for its commercial and industrial customers in the Electric Reliability Council of Texas (ERCOT) market. The challenge was to provide a platform that would allow these customers to lower their energy bills by adjusting their energy consumption during peak energy demand or high wholesale electricity prices. The solution needed to be intelligent, scalable, and offer both manual and automated options for adjusting energy consumption. The demand response programs needed to include Emergency Response Service (ERS), Real-Time Price Response (RTPR), and 4 Coincident Peak (4CP).
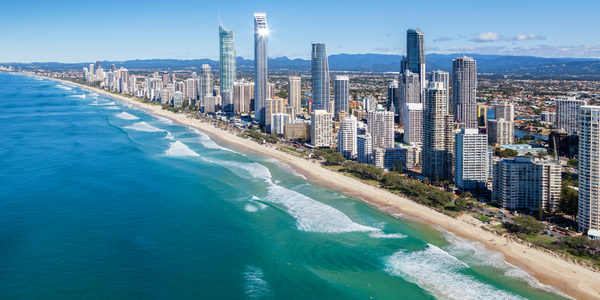
Case Study
ZettaNet's Agile Juniper Network Meets Booming Digital Demand in Australia
ZettaNet, a privately-held company based in Perth, Australia, was facing a significant challenge due to the exponential demand for enterprise network, data center, and cloud services in the region. The company's business growth necessitated an upgrade of their core network to meet the increasing bandwidth requirements of their customers. The customers, which primarily include managed service providers, were demanding 1 Gbps connectivity between locations. These service providers then deliver network, data center, cloud, and voice services to a diverse range of customers including local businesses, schools, hospitals, residential communities, and government offices in Western and Southern Australia. The challenge for ZettaNet was to meet this high-capacity network services demand while maintaining profitability.
Case Study
Blue Bottle Coffee Enhances Ordering Accuracy and Reduces Waste with ML-Driven Demand Forecasting
Blue Bottle Coffee (BBC), a global coffee roaster and retailer, faced a significant challenge in managing the supply of pastries across its international network of cafes. The company was using a manual ordering system, where cafe leaders estimated the required quantity of pastries based on historical sales data, current inventory, and growth projections. This system was effective when BBC had a few cafes, but with over 70 cafes worldwide, it became inefficient and inaccurate. The inaccuracies led to either under-ordering, causing sell-outs and customer dissatisfaction, or over-ordering, resulting in food waste and profit loss. The suboptimal utilization of pastries was also affecting BBC's bottom line. Therefore, BBC needed a scalable, precise, and predictive ordering solution to improve pastry ordering accuracy, reduce food waste, and meet its sustainability goals.
Case Study
MARS Incorporated: Leading a Global Digital Transformation
MARS Incorporated, a multinational manufacturer, faced significant challenges in digitizing and standardizing processes across its mid-markets globally. The company's reliance on legacy solutions necessitated continuous, time-consuming upgrades and made data compilation and comparison across different regions and business units difficult. Excel spreadsheets were extensively used for forecasting in smaller markets, leading to disconnected processes, siloed working environments, and increased risk of data inconsistencies and inaccuracies in demand forecasting. MARS also struggled with a lack of visibility across its midmarket footprint, scattered critical data across various systems and spreadsheets, a low degree of automation, and insufficient statistical analytics for demand planning. The absence of a standard process for demand planning made it challenging to consolidate KPIs and gain a comprehensive view of demand trends and supply chain performance.
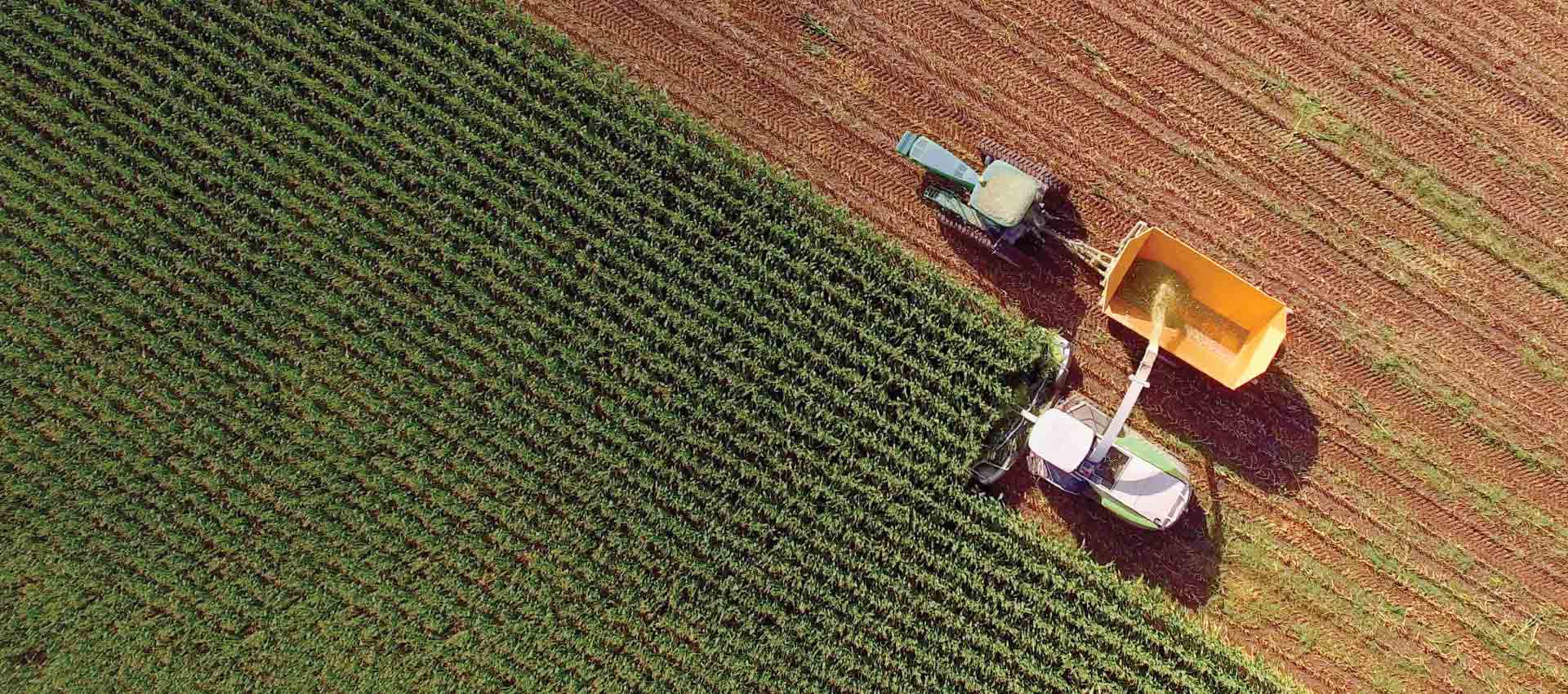
Case Study
Enterprise AI for Demand Forecasting and Production Scheduling in Global Agribusiness
A global agribusiness and food manufacturer, producing over 80 million pounds of food products per year across eight production lines, faced significant challenges in demand forecasting and production scheduling. The company's only customer, a global retailer, exhibited highly variable demand, leading to discrepancies with the manufacturer's weekly demand forecast. Traditional demand forecasting solutions, based on statistical algorithms, were unable to cope with the short lead times and daily sales orders due to the short shelf life of food products. This resulted in unfulfilled customer orders. Additional rule-based solutions procured to improve production scheduling also failed to optimize schedules and significantly improve manufacturing operations.