公司规模
SME
地区
- America
- Asia
国家
- United States
产品
- Domo BI & Analytics
技术栈
- Shopify
实施规模
- Enterprise-wide Deployment
影响指标
- Productivity Improvements
- Revenue Growth
技术
- 分析与建模 - 实时分析
适用功能
- 销售与市场营销
- 采购
用例
- 补货预测
- 需求计划与预测
服务
- 数据科学服务
关于客户
Cozy Earth 是一家专门生产睡眠产品的公司,采用优质 100% 竹纤维粘胶纤维。该公司的床单、棉被和睡衣旨在帮助睡眠者整晚调节体温。Cozy Earth 的产品连续四年登上奥普拉最喜欢的东西榜单,公司在过去三年中增长了 20 倍。Cozy Earth 的所有原材料和最终产品都来自亚洲,公司必须提前九个月预测最终产品在商店上市前的需求。
挑战
Cozy Earth 是一家生产竹制睡眠产品的公司,在管理其供应链和预测需求方面面临挑战。该公司的原材料和最终产品均由亚洲供应,需要提前九个月进行精确预测。订购过多可能会导致宝贵的现金被滞留在未售出的产品中,而订购不足则可能导致错失销售机会。在实施 Domo 之前,收集数据以进行预测是一个复杂的手动过程,需要从每个单独的渠道下载报告,将数据复制并粘贴到电子表格中,并花费数小时处理数据,然后才能供决策者使用。
解决方案
Cozy Earth 实施了 Domo 的 BI 和分析解决方案,以利用数据来管理和优化其增长。Domo 允许 Cozy Earth 自动收集、清理和整合来自 Shopify 和第三方销售渠道、Facebook 和 Google 等营销渠道以及为其仓库和配送系统提供支持的供应链平台的数据。这些数据可供每个部门使用,允许所有业务用户随时访问他们需要的数据,而无需等待数天才能获得报告。除了帮助公司明智地订购产品外,Cozy Earth 还使用 Domo 做出明智的决策,确定优先考虑哪些社交渠道以及在其数字营销中展示哪些产品。
运营影响
数量效益
Case Study missing?
Start adding your own!
Register with your work email and create a new case study profile for your business.
相关案例.
Case Study
Pepsico's Transformation to Smarter Sales Forecasting with Designer Cloud
PepsiCo, a global consumer packaged goods company, faced a significant challenge in calibrating sales forecasting to supply the right product quantities to its retailers. The sales forecast incorporated a variety of data, including warehouse data, store stock data, and promotional forecast data, all of which were provided by retailers in different file formats and delivered using various methods. The primary challenge was the speed of preparing a sales forecast. With the existing Microsoft Access and Excel-based processes, the time required to prepare this data was so extensive that analysts could only leverage it once a month or not at all. This inefficiency risked under or oversupplying retailers, potentially impacting PepsiCo's business operations and customer relationships.
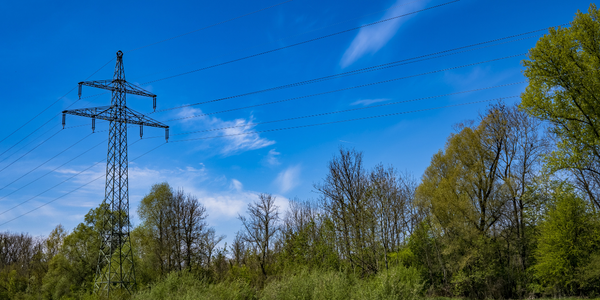
Case Study
Gexa Energy and AutoGrid's Innovative Demand Response Programs in ERCOT
Gexa Energy, a leading retail electricity provider in Texas, was seeking to introduce new demand response programs for its commercial and industrial customers in the Electric Reliability Council of Texas (ERCOT) market. The challenge was to provide a platform that would allow these customers to lower their energy bills by adjusting their energy consumption during peak energy demand or high wholesale electricity prices. The solution needed to be intelligent, scalable, and offer both manual and automated options for adjusting energy consumption. The demand response programs needed to include Emergency Response Service (ERS), Real-Time Price Response (RTPR), and 4 Coincident Peak (4CP).
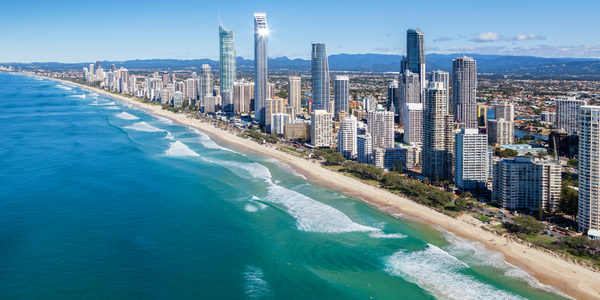
Case Study
ZettaNet's Agile Juniper Network Meets Booming Digital Demand in Australia
ZettaNet, a privately-held company based in Perth, Australia, was facing a significant challenge due to the exponential demand for enterprise network, data center, and cloud services in the region. The company's business growth necessitated an upgrade of their core network to meet the increasing bandwidth requirements of their customers. The customers, which primarily include managed service providers, were demanding 1 Gbps connectivity between locations. These service providers then deliver network, data center, cloud, and voice services to a diverse range of customers including local businesses, schools, hospitals, residential communities, and government offices in Western and Southern Australia. The challenge for ZettaNet was to meet this high-capacity network services demand while maintaining profitability.
Case Study
Blue Bottle Coffee Enhances Ordering Accuracy and Reduces Waste with ML-Driven Demand Forecasting
Blue Bottle Coffee (BBC), a global coffee roaster and retailer, faced a significant challenge in managing the supply of pastries across its international network of cafes. The company was using a manual ordering system, where cafe leaders estimated the required quantity of pastries based on historical sales data, current inventory, and growth projections. This system was effective when BBC had a few cafes, but with over 70 cafes worldwide, it became inefficient and inaccurate. The inaccuracies led to either under-ordering, causing sell-outs and customer dissatisfaction, or over-ordering, resulting in food waste and profit loss. The suboptimal utilization of pastries was also affecting BBC's bottom line. Therefore, BBC needed a scalable, precise, and predictive ordering solution to improve pastry ordering accuracy, reduce food waste, and meet its sustainability goals.
Case Study
MARS Incorporated: Leading a Global Digital Transformation
MARS Incorporated, a multinational manufacturer, faced significant challenges in digitizing and standardizing processes across its mid-markets globally. The company's reliance on legacy solutions necessitated continuous, time-consuming upgrades and made data compilation and comparison across different regions and business units difficult. Excel spreadsheets were extensively used for forecasting in smaller markets, leading to disconnected processes, siloed working environments, and increased risk of data inconsistencies and inaccuracies in demand forecasting. MARS also struggled with a lack of visibility across its midmarket footprint, scattered critical data across various systems and spreadsheets, a low degree of automation, and insufficient statistical analytics for demand planning. The absence of a standard process for demand planning made it challenging to consolidate KPIs and gain a comprehensive view of demand trends and supply chain performance.
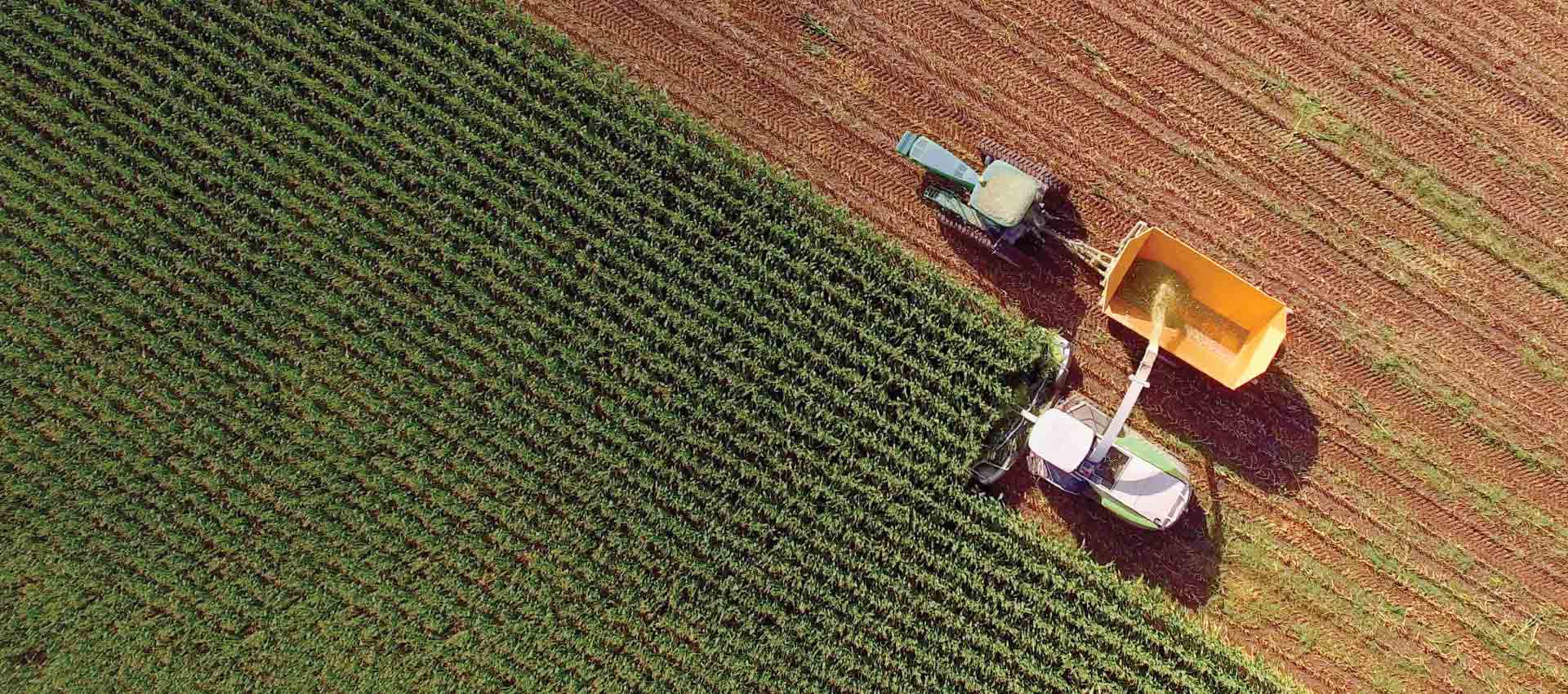
Case Study
Enterprise AI for Demand Forecasting and Production Scheduling in Global Agribusiness
A global agribusiness and food manufacturer, producing over 80 million pounds of food products per year across eight production lines, faced significant challenges in demand forecasting and production scheduling. The company's only customer, a global retailer, exhibited highly variable demand, leading to discrepancies with the manufacturer's weekly demand forecast. Traditional demand forecasting solutions, based on statistical algorithms, were unable to cope with the short lead times and daily sales orders due to the short shelf life of food products. This resulted in unfulfilled customer orders. Additional rule-based solutions procured to improve production scheduling also failed to optimize schedules and significantly improve manufacturing operations.