Customer Company Size
SME
Region
- America
Country
- United States
Product
- Sisense
Tech Stack
- Embedded Analytics
- Data Warehousing
- ETL (Extract, Transform, Load)
Implementation Scale
- Enterprise-wide Deployment
Impact Metrics
- Customer Satisfaction
- Productivity Improvements
- Revenue Growth
Technology Category
- Analytics & Modeling - Big Data Analytics
- Analytics & Modeling - Predictive Analytics
- Application Infrastructure & Middleware - Data Exchange & Integration
Applicable Industries
- Automotive
- E-Commerce
- Healthcare & Hospitals
Applicable Functions
- Business Operation
- Sales & Marketing
Services
- Data Science Services
- Software Design & Engineering Services
- System Integration
About The Customer
Known Factors provides business intelligence services and solutions for a variety of industries including real estate, travel, automotive, e-commerce, healthcare, and political campaigns. Most of their clients come to them after they have failed to implement a BI solution themselves. Their data is usually complex and distributed, requiring significant efforts to prepare the data that most BI tools cannot provide. As a data warehouse and BI expert, Mike van Thiel, CEO and co-founder, was even having issues with the steep learning curve of the BI solutions he looked at prior to Sisense. Once Sisense was deployed, it was a much easier sell to clients, which greatly improved Known Factors bottom line.
The Challenge
Many companies today often have data that is so complex or distributed, it needs a dedicated team of specialists to clean and maintain it, and only then can a BI tool be useful. There’s a lot of work preparing complicated and dirty data, and many businesses need a simple way to prepare data that most BI tools on the market cannot provide. Mike saw that even after implementing a BI solution for customers. There was often such a steep learning curve, the customer would still be unable to build reports and dashboards independently. Mike’s customers typically come to him after they've failed with another BI solution. His company then takes on managing the client's internal data, some of which as been around for 10+ years, and is big, disparate, and dirty. His team would then wrangle the data together into a BI solution for the client. Mike saw that even after implementing a BI solution for customers, it often had such a steep learning curve, the customer would still be unable to build reports and dashboards independently. It was important that his customers could be self-sufficient, so he wanted to standardize on a tool that he knew would be a win for them.
The Solution
After evaluating and using different top-tier BI tools, Mike was still left without a solution. He's a very technical guy and the effort to produce a single report with any of the other tools was too extreme. When he tried Sisense’s trial, he was pleasantly surprised as he was able to build meaningful dashboards without investing any time in learning how to use Sisense beforehand. It was obvious that this was exactly what his customers needed. Known Factors is using Sisense in their political vertical to help candidates win elections based on data. They created a data warehouse based on parameters such as voter attributes, election results, campaign finance, and political history. Sisense dashboards and reports are used to deliver an easily operated statistical analysis of a voting district. “When someone runs for office, whether it is for governor or city council, they basically have to start a little business,” Mike explained. However, they don’t want to spend hundreds of thousands of dollars on a 3-6 month campaign. Known Factors provides a service where users (strictly political figures running for office) gain access to these comprehensive political dashboards and reports, and then help them analyze this data in order to glean meaningful insights.
Operational Impact
Case Study missing?
Start adding your own!
Register with your work email and create a new case study profile for your business.
Related Case Studies.
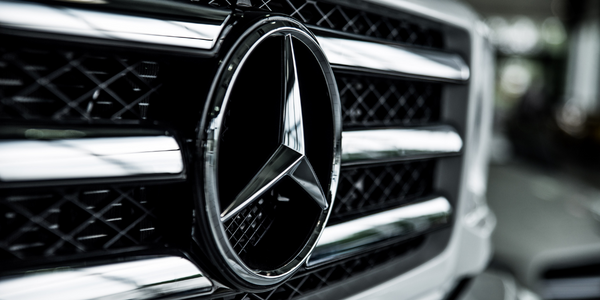
Case Study
Integral Plant Maintenance
Mercedes-Benz and his partner GAZ chose Siemens to be its maintenance partner at a new engine plant in Yaroslavl, Russia. The new plant offers a capacity to manufacture diesel engines for the Russian market, for locally produced Sprinter Classic. In addition to engines for the local market, the Yaroslavl plant will also produce spare parts. Mercedes-Benz Russia and his partner needed a service partner in order to ensure the operation of these lines in a maintenance partnership arrangement. The challenges included coordinating the entire maintenance management operation, in particular inspections, corrective and predictive maintenance activities, and the optimizing spare parts management. Siemens developed a customized maintenance solution that includes all electronic and mechanical maintenance activities (Integral Plant Maintenance).
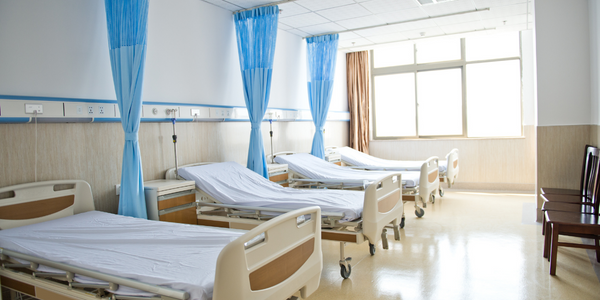
Case Study
Hospital Inventory Management
The hospital supply chain team is responsible for ensuring that the right medical supplies are readily available to clinicians when and where needed, and to do so in the most efficient manner possible. However, many of the systems and processes in use at the cancer center for supply chain management were not best suited to support these goals. Barcoding technology, a commonly used method for inventory management of medical supplies, is labor intensive, time consuming, does not provide real-time visibility into inventory levels and can be prone to error. Consequently, the lack of accurate and real-time visibility into inventory levels across multiple supply rooms in multiple hospital facilities creates additional inefficiency in the system causing over-ordering, hoarding, and wasted supplies. Other sources of waste and cost were also identified as candidates for improvement. Existing systems and processes did not provide adequate security for high-cost inventory within the hospital, which was another driver of cost. A lack of visibility into expiration dates for supplies resulted in supplies being wasted due to past expiry dates. Storage of supplies was also a key consideration given the location of the cancer center’s facilities in a dense urban setting, where space is always at a premium. In order to address the challenges outlined above, the hospital sought a solution that would provide real-time inventory information with high levels of accuracy, reduce the level of manual effort required and enable data driven decision making to ensure that the right supplies were readily available to clinicians in the right location at the right time.