Customer Company Size
SME
Region
- America
Country
- Canada
Product
- CovidWisdom app
- Pachyderm
Tech Stack
- Machine Learning
- AI architecture
- Data Science
- Docker containers
Implementation Scale
- Enterprise-wide Deployment
Impact Metrics
- Productivity Improvements
- Innovation Output
Technology Category
- Analytics & Modeling - Machine Learning
- Application Infrastructure & Middleware - Data Exchange & Integration
Applicable Industries
- Healthcare & Hospitals
- Cities & Municipalities
Applicable Functions
- Business Operation
Use Cases
- Predictive Maintenance
- Public Transportation Management
Services
- Data Science Services
About The Customer
Riskthinking.AI is a company that specializes in measuring the financial risk of climate change. They work with companies and governments to help them make the best decisions when it comes to uncertain futures. For example, they might help an electric company decide whether to rebuild transformers in the same spots that caused forest fires in the past or to put them in a different configuration to reduce the chance of starting another fire in the future. They also help companies figure out how quickly they can ramp up a solar farm and where they should put it. However, they realized early on that while they had experts in predicting the future, they did not have expertise in building AI architecture.
The Challenge
Riskthinking.AI, a company specializing in measuring the financial risk of climate change, was in the early phases of ramping up their internal AI infrastructure when they took on the CovidWisdom project. The project was a response to a call from the Canadian government to assess the economic impact of major pandemic policies. The challenge was to predict the best way to implement societal-level responses like lockdowns with the minimum amount of damage to daily life and the economy. However, the team realized they had experts in predicting the future but not in building AI architecture. They had data scientists working on laptops, pulling and pushing data over VPNs to remote work spots, and even building their own Docker containers. They needed to move from ad hoc to MLOps.
The Solution
Riskthinking.AI decided to use Pachyderm, a platform that allowed their scientists to focus on the complexity of models rather than the complexity of figuring out which model was trained on which version of the dataset. It gave them the foundation to work with data and deploy any ML tool they wanted inside their machine learning loop. As Riskthinking.AI’s data scientists got more comfortable with pachctl and the command line, they used Pachyderm to run multiple models simultaneously and to visualize backtesting results with easy to understand images. The best performing model got automatically pushed to the application for the current day. The visualizations were not just for the data science team. They could also share their progress with non-technical or less technical stakeholders.
Operational Impact
Quantitative Benefit
Case Study missing?
Start adding your own!
Register with your work email and create a new case study profile for your business.
Related Case Studies.
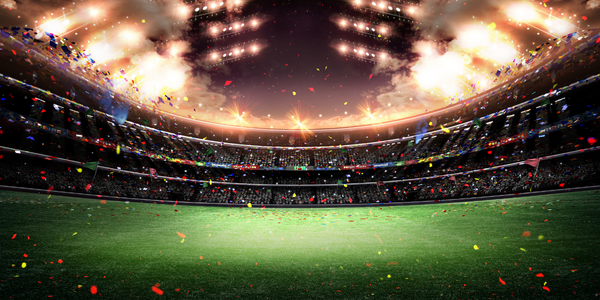
Case Study
Turning A Stadium Into A Smart Building
Honeywell created what it called the “intelligent system” for the National Stadium in Beijing, China, turning the venue for the opening and closing events at the 2008 Summer Olympics into a “smart building.” Designed by highly controversial artist Ai Weiwei, the “Bird’s Nest” remains one of the most impressive feats of stadium architecture in the world. The 250,000 square meter structure housed more than 100,000 athletes and spectators at a time. To accommodate such capacity, China turned to Honeywell’s EBI Integrated Building Management System to create an integrated “intelligent system” for improved building security, safety and energy efficiency.
.png)
Case Study
Smart Street Light Network (Copenhagen)
Key stakeholders are taking a comprehensive approach to rethinking smart city innovation. City leaders have collaborated through partnerships involving government, research institutions and solution providers. The Copenhagen Solutions Lab is one of the leading organizations at the forefront of this movement. By bringing together manufacturers with municipal buyers, the Copenhagen Solutions Lab has catalyzed the development and deployment of next-generation smart city innovations. Copenhagen is leveraging this unique approach to accelerate the implementation of smart city solutions. One of the primary focus areas is LED street lighting.
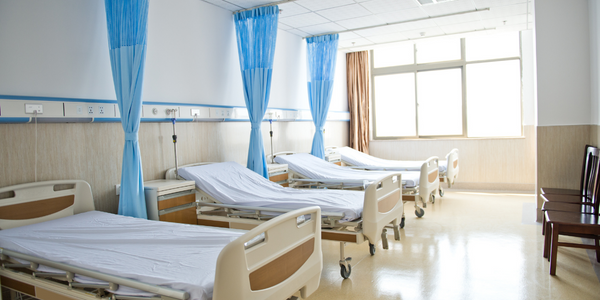
Case Study
Hospital Inventory Management
The hospital supply chain team is responsible for ensuring that the right medical supplies are readily available to clinicians when and where needed, and to do so in the most efficient manner possible. However, many of the systems and processes in use at the cancer center for supply chain management were not best suited to support these goals. Barcoding technology, a commonly used method for inventory management of medical supplies, is labor intensive, time consuming, does not provide real-time visibility into inventory levels and can be prone to error. Consequently, the lack of accurate and real-time visibility into inventory levels across multiple supply rooms in multiple hospital facilities creates additional inefficiency in the system causing over-ordering, hoarding, and wasted supplies. Other sources of waste and cost were also identified as candidates for improvement. Existing systems and processes did not provide adequate security for high-cost inventory within the hospital, which was another driver of cost. A lack of visibility into expiration dates for supplies resulted in supplies being wasted due to past expiry dates. Storage of supplies was also a key consideration given the location of the cancer center’s facilities in a dense urban setting, where space is always at a premium. In order to address the challenges outlined above, the hospital sought a solution that would provide real-time inventory information with high levels of accuracy, reduce the level of manual effort required and enable data driven decision making to ensure that the right supplies were readily available to clinicians in the right location at the right time.
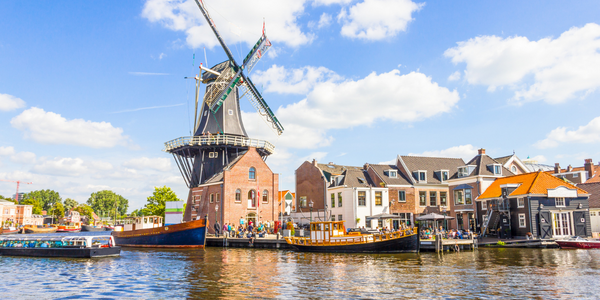
Case Study
Buoy Status Monitoring with LoRa
The Netherlands are well-known for their inland waterways, canals, sluices and of course port activities. The Dutch Ministry of Infrastructure indicates that there are thousands of buoys and fixed items in and near water environments that would profit from IoT monitoring. One of the problems with buoys for example, is that they get hit by ships and the anchor cable breaks. Without connectivity, it takes quite some time to find out that something has happened with that buoy. Not to mention the costs of renting a boat to go to the buoy to fix it. Another important issue, is that there is no real-time monitoring of the buoys at this moment. Only by physically visiting the object on the water, one gains insight in its status.
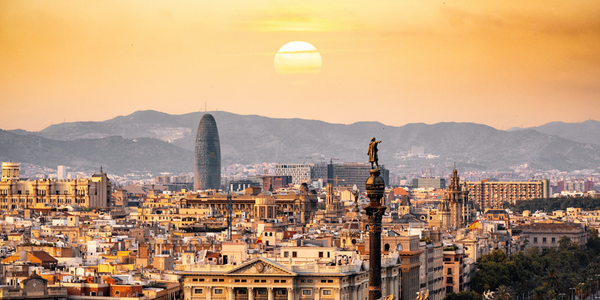
Case Study
Barcelona Case Study
Barcelona’s heavy traffic and its associated high levels of pollution were the primary factors that motivated some companies and universities to work on strategies for improving traffic in the city centre. Bitcarrier is one of the technologies involved in the In4Mo Project, whose main objective is to develop the applications that form the core of smart mobility, one of the fundamental pillars of the smart city concept.