Technology Category
- Functional Applications - Computerized Maintenance Management Systems (CMMS)
- Networks & Connectivity - 5G
Applicable Industries
- Packaging
Applicable Functions
- Maintenance
- Quality Assurance
Use Cases
- Inventory Management
- Supply Chain Visibility
Services
- System Integration
- Testing & Certification
About The Customer
Plastico Dise is a leading manufacturer of plastic packaging products for the food and pharmaceutical industries. With production sites in three South American countries and a team of over 250 employees, Dise is well-equipped to provide quality products and services to companies across the region. The company was looking to reduce machine stops on their production lines and improve visibility into why these stops were happening. To achieve this, they implemented Poka, a Connected Worker app, at two of their production sites in 2018.
The Challenge
Plastico Dise, a manufacturer of plastic packaging products, was facing challenges in managing issues on their production lines. The company was struggling with frequent machine stops and lacked visibility into the reasons behind these stops. The issue management and resolution process was cumbersome and inefficient. When operators encountered a problem, they had to contact maintenance through internal telephones and intercoms. The maintenance team would then log the issues, download work orders to a spreadsheet, print and post the paperwork order schedules to a blackboard in the workshop. This manual process was time-consuming and left room for optimization. Additionally, quality, continuous improvement, and IT issues were reported via email, leading to communication difficulties and tracking issues. The company was operating in silos with limited visibility due to the existence of two separate issue reporting systems.
The Solution
To address these challenges, Dise implemented Poka, a Connected Worker app, in 2018 at two of their production sites. Poka revolutionized how maintenance issues were managed at Dise. The app allowed any operator, regardless of their position or seniority, to upload an incident. The operators could choose the issue type, priority level, and location, add a description, and even attach photos and videos for further context. The relevant department or team would be immediately notified via email or push notification. The problem could also be shared on the factory newsfeed to encourage wider support and group problem-solving. Poka also introduced numerical codes for customizable issue types, which helped in prioritizing and categorizing problems for faster resolution. The maintenance team could track open issues on a digital Kanban board or filter them to identify the most critical or persistent problems. Poka was integrated with Dise's CMMS, Hippo, reducing the need for data reentry and saving significant time and labor.
Operational Impact
Quantitative Benefit
Case Study missing?
Start adding your own!
Register with your work email and create a new case study profile for your business.
Related Case Studies.
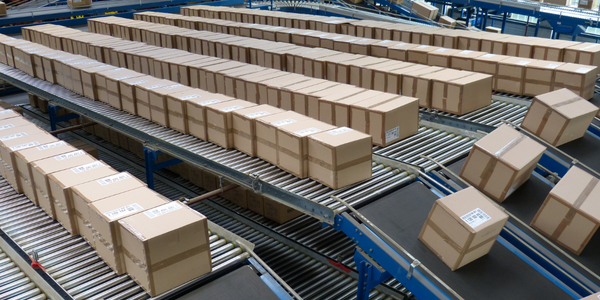
Case Study
IoT Data Analytics Case Study - Packaging Films Manufacturer
The company manufactures packaging films on made to order or configure to order basis. Every order has a different set of requirements from the product characteristics perspective and hence requires machine’s settings to be adjusted accordingly. If the film quality does not meet the required standards, the degraded quality impacts customer delivery causes customer dissatisfaction and results in lower margins. The biggest challenge was to identify the real root cause and devise a remedy for that.
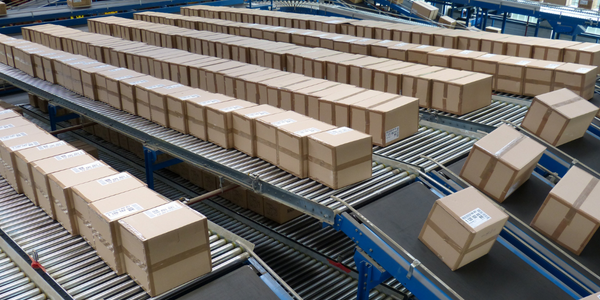
Case Study
Zenon the Ideal Basis for An Ergonomic HMI
KHS develops and produces machines and equipment for filling and packaging in the drinks industry. Because drinks manufacturing, filling and packaging consist of a number of highly complex processes, the user-friendly and intuitive operation of equipment is increasingly gaining in significance. In order to design these processes as simple as possible for the user, KHS decided to introduce a uniform, transparent and standardized solution to the company. The HMI interface should meet the requirement for people with different qualifications and enable them to work on a standard platform.
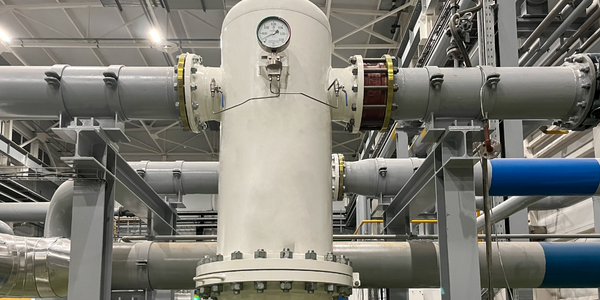
Case Study
Sparks Dynamics Assists Atlas Container Secure a $15,000 BGE Energy Rebate
The ReMASTER Compressed Air Monitoring system was installed in 2015. This system is capable of monitoring compressed air system parameters on a continuous basis and transferring that information to a cloud server which can be accessed by Atlas Container personnel, Industrial Diagnostics and Sparks Dynamics. This information was collected into a database which can be exported to an Excel spreadsheet or displayed graphically using Sparks Dynamics ViewMaster Software. The average annual compressed air electricity expense was estimated to be approximately $116,000. This is based on an incremental $/KWh electric rate of $.091 per KWh and an estimated compressed air energy consumption of 1,279,200 KWH. The implementation phase of Energy Conservation Measures (ECMs) for the Compressed Air System included: • Identification and repair of compressed air leaks • Understanding of compressed air usage per manufacturing machine and installation of shut off valves when the machines are no longer in production mode • Identification of misapplications of compressed air to include blow offs, venturis, and cooling scenarios • Understand system pressure requirements and potential installation of point of use pressure regulation.
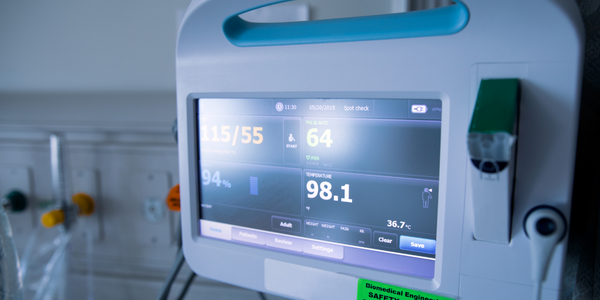
Case Study
Mondi Implements Statistics-Based Health Monitoring and Predictive Maintenance
The extrusion and other machines at Mondi’s plant are large and complex, measuring up to 50 meters long and 15 meters high. Each machine is controlled by up to five programmable logic controllers (PLCs), which log temperature, pressure, velocity, and other performance parameters from the machine’s sensors. Each machine records 300–400 parameter values every minute, generating 7 gigabytes of data daily.Mondi faced several challenges in using this data for predictive maintenance. First, the plant personnel had limited experience with statistical analysis and machine learning. They needed to evaluate a variety of machine learning approaches to identify which produced the most accurate results for their data. They also needed to develop an application that presented the results clearly and immediately to machine operators. Lastly, they needed to package this application for continuous use in a production environment.
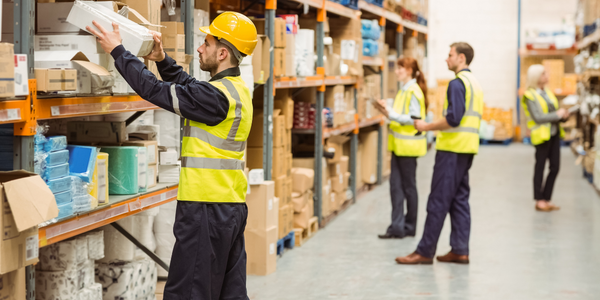
Case Study
Automated Pallet Labeling Solution for SPR Packaging
SPR Packaging, an American supplier of packaging solutions, was in search of an automated pallet labeling solution that could meet their immediate and future needs. They aimed to equip their lines with automatic printer applicators, but also required a solution that could interface with their accounting software. The challenge was to find a system that could read a 2D code on pallets at the stretch wrapper, track the pallet, and flag any pallets with unread barcodes for inspection. The pallets could be single or double stacked, and the system needed to be able to differentiate between the two. SPR Packaging sought a system integrator with extensive experience in advanced printing and tracking solutions to provide a complete traceability system.
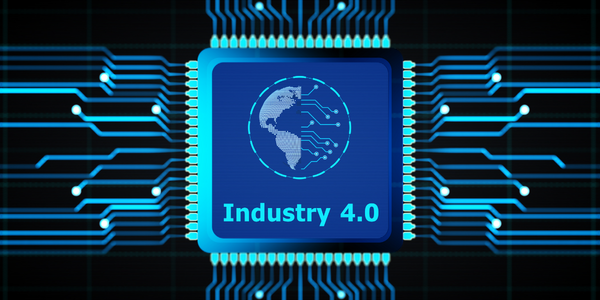
Case Study
Industry 4.0 at ALPLA: Enhancing Factory Efficiency with IoT
ALPLA, a global leader in packaging solutions, faced several challenges as the complexity of their production machinery increased. The need for highly trained specialists in each factory led to higher personnel costs, difficulties in recruiting experienced talent at each location, and costly personnel turnover. Furthermore, less experienced operators running the machines sub-optimally impacted resource consumption and overall equipment effectiveness (OEE). ALPLA also faced the challenge of monitoring visual inspection systems in every line of their plants, which was almost impossible to do manually. In 2016, ALPLA decided to use data from the 900 different types of embedded sensors in each factory to address these issues. However, their initial choice of SQL Server as the data store for the sensor data proved inadequate, as it was unable to cope with their data requirements.