Product
- Anodot
Tech Stack
- Machine Learning Algorithms
Implementation Scale
- Enterprise-wide Deployment
Impact Metrics
- Productivity Improvements
- Digital Expertise
Technology Category
- Analytics & Modeling - Real Time Analytics
- Analytics & Modeling - Predictive Analytics
Applicable Functions
- Discrete Manufacturing
Use Cases
- Predictive Maintenance
- Real-Time Location System (RTLS)
Services
- Data Science Services
About The Customer
Uprise is an ad-tech company that specializes in performance-advertising. The company uses its machine learning algorithms to target the best performing ad placements for its customers. Uprise develops its software using the 'continuous delivery' approach, which means that the team pushes around 20 new software releases into production each day. The company needs to keep track of hundreds of thousands of metrics, which is the lifeblood of its business. Each new release can affect the ad-tech platform’s performance (positively or negatively), so it is crucial to monitor results in a timely fashion to determine if the new release should be kept in production or rolled back.
The Challenge
Uprise, an ad-tech company, uses a 'continuous delivery' approach for its software development, pushing around 20 new software releases into production each day. Each new release can affect the platform’s performance, making it crucial to monitor results in a timely fashion to determine if the new release should be kept in production or rolled back. The ad tech environment itself has many moving parts, each of which is a potential point of failure. These can include server issues, changes at the ad affiliates, introduction of ad blocking software, or even fraud. Whenever a problem occurs, isolating the source can require complex, time-consuming analysis. Identifying issues in the first place is also tricky, since network traffic behaves seasonally. With the traffic naturally reaching various peaks and valleys throughout the day, noticing a 20% loss or gain at any given point is next to impossible.
The Solution
Uprise uses Anodot to track KPIs such as revenue, spend, fill-rate, and performance. With Anodot, Uprise can monitor everything, letting Anodot do the work of identifying anomalies. Anodot alerts much earlier since it is looking for the metric anomalies, rather than relying on static thresholds. Anodot provides the exact timestamp where an anomaly began, so the Uprise team can correlate it to a specific software release. Anodot is so easy to use that every single Uprise employee has a login to Anodot – from R&D to devops/IT to business intelligence – and most are using it all the time.
Operational Impact
Case Study missing?
Start adding your own!
Register with your work email and create a new case study profile for your business.
Related Case Studies.
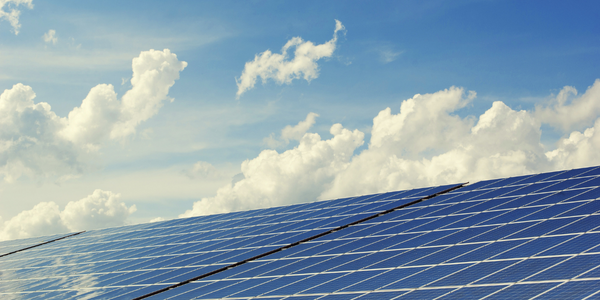
Case Study
Remote Monitoring & Predictive Maintenance App for a Solar Energy System
The maintenance & tracking of various modules was an overhead for the customer due to the huge labor costs involved. Being an advanced solar solutions provider, they wanted to ensure early detection of issues and provide the best-in-class customer experience. Hence they wanted to automate the whole process.
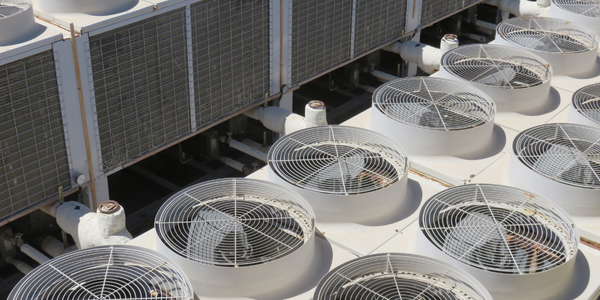
Case Study
Predictive Maintenance for Industrial Chillers
For global leaders in the industrial chiller manufacturing, reliability of the entire production process is of the utmost importance. Chillers are refrigeration systems that produce ice water to provide cooling for a process or industrial application. One of those leaders sought a way to respond to asset performance issues, even before they occur. The intelligence to guarantee maximum reliability of cooling devices is embedded (pre-alarming). A pre-alarming phase means that the cooling device still works, but symptoms may appear, telling manufacturers that a failure is likely to occur in the near future. Chillers who are not internet connected at that moment, provide little insight in this pre-alarming phase.
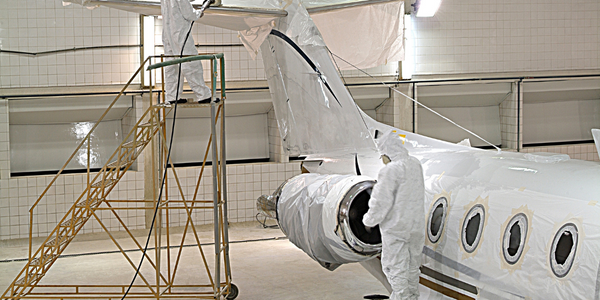
Case Study
Aircraft Predictive Maintenance and Workflow Optimization
First, aircraft manufacturer have trouble monitoring the health of aircraft systems with health prognostics and deliver predictive maintenance insights. Second, aircraft manufacturer wants a solution that can provide an in-context advisory and align job assignments to match technician experience and expertise.
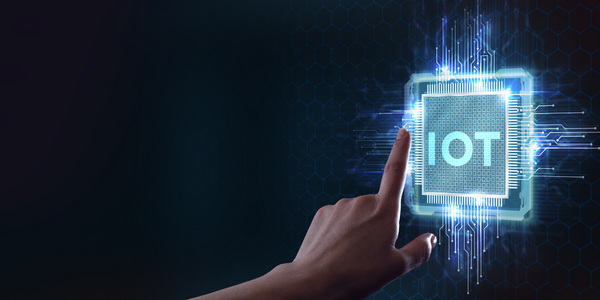
Case Study
Leading Tools Manufacturer Transforms Operations with IoT
Stanley Black & Decker required transparency of real-time overall equipment effectiveness and line productivity to reduce production line change over time.The goal was to to improve production to schedule, reduce actual labor costs and understanding the effects of shift changes and resource shifts from line to line.
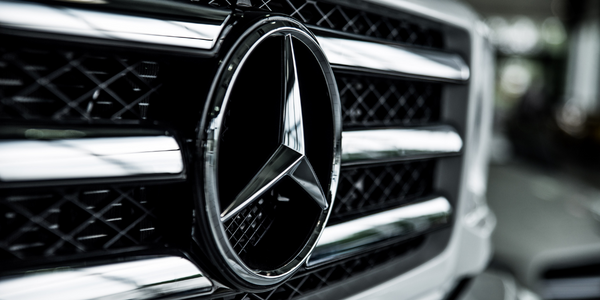
Case Study
Integral Plant Maintenance
Mercedes-Benz and his partner GAZ chose Siemens to be its maintenance partner at a new engine plant in Yaroslavl, Russia. The new plant offers a capacity to manufacture diesel engines for the Russian market, for locally produced Sprinter Classic. In addition to engines for the local market, the Yaroslavl plant will also produce spare parts. Mercedes-Benz Russia and his partner needed a service partner in order to ensure the operation of these lines in a maintenance partnership arrangement. The challenges included coordinating the entire maintenance management operation, in particular inspections, corrective and predictive maintenance activities, and the optimizing spare parts management. Siemens developed a customized maintenance solution that includes all electronic and mechanical maintenance activities (Integral Plant Maintenance).