Technology Category
- Functional Applications - Warehouse Management Systems (WMS)
- Platform as a Service (PaaS) - Application Development Platforms
Applicable Industries
- Equipment & Machinery
- Finance & Insurance
Applicable Functions
- Sales & Marketing
- Warehouse & Inventory Management
Use Cases
- Leasing Finance Automation
- Time Sensitive Networking
Services
- Data Science Services
- System Integration
About The Customer
Blend is a fintech startup founded in 2012 that has risen to prominence in the banking software space. The company announced its IPO in July 2021. In the last year alone, Blend enabled financial services firms to process nearly $1.4 trillion in loan applications, handling an average of more than $5 billion in transactions every day. Blend's vision is to re-architect banking software around the consumer, providing a customer experience in line with the service offered by other disruptive, digital-first applications and businesses. This requires massive internal coordination, which is central to the roles of Ryan Dunlap and William Tsu, who work on the Customer Success Operations team at Blend. Their team’s purpose is to build out large, innovative business systems to enable the business to move quickly.
The Challenge
Blend, a fintech startup, was facing a significant challenge with its data ingestion process. Despite having adopted a modern data stack approach with Redshift at its core, getting data into and out of the data warehouse was proving to be a complex and time-consuming task. The process of pulling a single column from Salesforce or changing a field could take weeks, limiting access to time-critical data. The team was unable to prototype and rapidly iterate, and had to release straight to production to test their solutions, causing further complications for the operations team. As the company expanded, new tools like Asana, Marketo, and Lever were introduced to manage workflows and processes, each requiring data to be synced inside them to be effective. With the data engineering team’s limited bandwidth, they did not have the capacity to maintain a rapidly expanding list of SaaS platforms. This led to a decision point: commit to in-house tooling, or look for external providers.
The Solution
In their search for a solution, Blend's team laid out clear criteria for assessing ELT and Reverse ETL solutions, including ease of use, connector support, infosec requirements, and alerting capabilities. They needed a tool that was self-serve and would allow the team to deploy rapidly, with little to no dependency on data engineering. Fivetran emerged as a clear frontrunner for handling the extract, load, and transformation process. For Reverse ETL, Hightouch’s impact was immediate and transformative. The Hightouch debugger and Slack integration is now used by the Blend team to understand when the Reverse ETL process has failed, enabling a greater degree of trust in data accuracy across the board. The finance team, in particular, benefited from access to reliable data. Thanks to Fivetran and Hightouch’s Asana connectors, Blend’s finance team is now able to monitor and enforce time entry, understand project timelines, and close out services team financial reporting in three business days, where it previously took up to seven.
Operational Impact
Quantitative Benefit
Case Study missing?
Start adding your own!
Register with your work email and create a new case study profile for your business.
Related Case Studies.
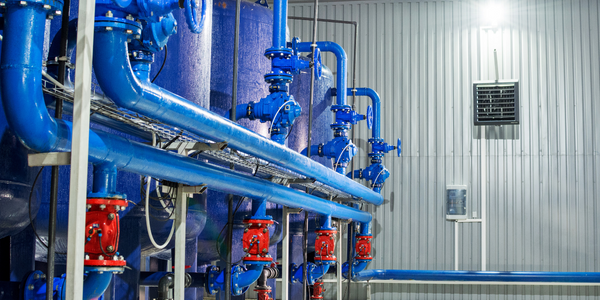
Case Study
Smart Water Filtration Systems
Before working with Ayla Networks, Ozner was already using cloud connectivity to identify and solve water-filtration system malfunctions as well as to monitor filter cartridges for replacements.But, in June 2015, Ozner executives talked with Ayla about how the company might further improve its water systems with IoT technology. They liked what they heard from Ayla, but the executives needed to be sure that Ayla’s Agile IoT Platform provided the security and reliability Ozner required.
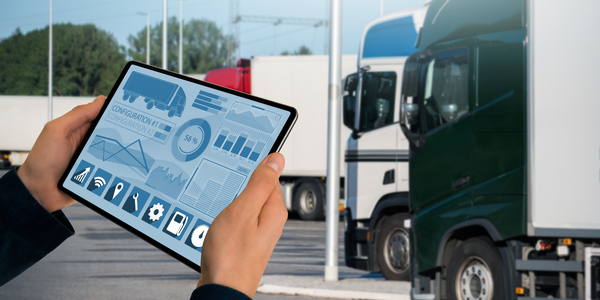
Case Study
IoT enabled Fleet Management with MindSphere
In view of growing competition, Gämmerler had a strong need to remain competitive via process optimization, reliability and gentle handling of printed products, even at highest press speeds. In addition, a digitalization initiative also included developing a key differentiation via data-driven services offers.
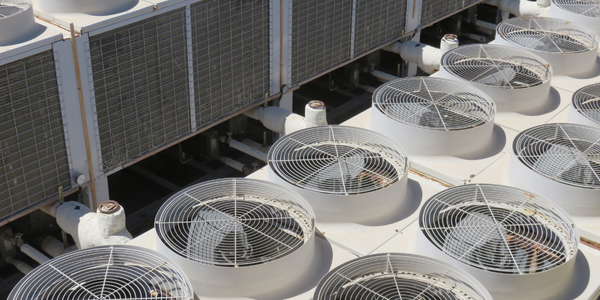
Case Study
Predictive Maintenance for Industrial Chillers
For global leaders in the industrial chiller manufacturing, reliability of the entire production process is of the utmost importance. Chillers are refrigeration systems that produce ice water to provide cooling for a process or industrial application. One of those leaders sought a way to respond to asset performance issues, even before they occur. The intelligence to guarantee maximum reliability of cooling devices is embedded (pre-alarming). A pre-alarming phase means that the cooling device still works, but symptoms may appear, telling manufacturers that a failure is likely to occur in the near future. Chillers who are not internet connected at that moment, provide little insight in this pre-alarming phase.
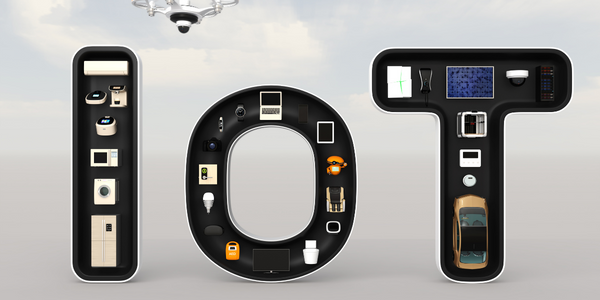
Case Study
Premium Appliance Producer Innovates with Internet of Everything
Sub-Zero faced the largest product launch in the company’s history:It wanted to launch 60 new products as scheduled while simultaneously opening a new “greenfield” production facility, yet still adhering to stringent quality requirements and manage issues from new supply-chain partners. A the same time, it wanted to increase staff productivity time and collaboration while reducing travel and costs.
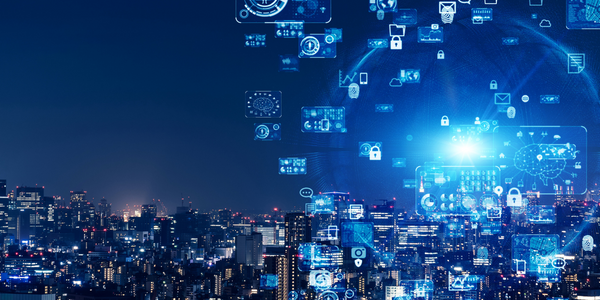
Case Study
Integration of PLC with IoT for Bosch Rexroth
The application arises from the need to monitor and anticipate the problems of one or more machines managed by a PLC. These problems, often resulting from the accumulation over time of small discrepancies, require, when they occur, ex post technical operations maintenance.
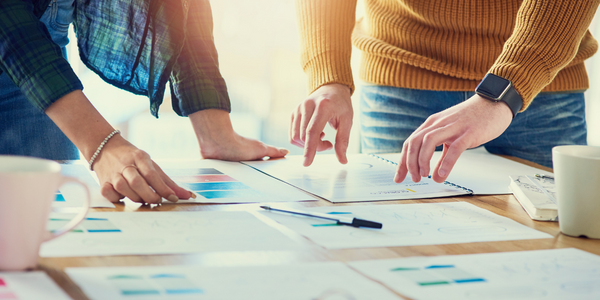
Case Study
Data Gathering Solution for Joy Global
Joy Global's existing business processes required customers to work through an unstable legacy system to collect mass volumes of data. With inadequate processes and tools, field level analytics were not sufficient to properly inform business decisions.