Technology Category
- Analytics & Modeling - Machine Learning
- Analytics & Modeling - Predictive Analytics
Applicable Industries
- Education
- Equipment & Machinery
Applicable Functions
- Sales & Marketing
- Warehouse & Inventory Management
Use Cases
- Picking, Sorting & Positioning
- Time Sensitive Networking
Services
- Data Science Services
About The Customer
Databricks is a business that prides itself on helping data teams solve the world’s biggest challenges. It was founded in 2013 by the original creators of Apache Spark, Delta Lake and MLflow. Built on a modern Lakehouse architecture in the cloud, Databricks combines the best of data warehouses and data lakes to offer an open and unified platform for data and AI. The company has rapidly expanded, leading to the need for centralized and documented data. However, data silos were appearing around the business, including on the marketing team, where data was stored in its own data warehouse.
The Challenge
Chris Klaczynski, a Marketing Analytics Manager at Databricks, was tasked with supporting the primary marketing objectives of driving pipeline generation, growing the database, and improving ROI. However, as Databricks rapidly expanded, the need for centralized and documented data became more and more apparent. Data silos were appearing around the business, including on Chris’ marketing team, where data was stored in its own data warehouse. It was critical that Chris’ newly-built dashboards were supplied with trustworthy, timely data for marketing operations to keep running smoothly. However, without dedicated engineering resources, and in the face of a rapidly expanding marketing team, scaling with demand became next to impossible. Databricks faced a number of challenges with their traditional data warehouse, including issues with their Salesforce and Marketo pipelines, issues appending data natively to existing tables, and schema changes that were always breaking pipelines, resulting in outages and stale, untrustworthy data.
The Solution
Chris began looking for a low-code, turnkey solution that would give his team the reliable pipelines they needed. He decided on a three-point strategy: Get out of the data engineering ‘jungle’ and into insights and predictive analytics, hire analysts and Digital SMEs, not Data Engineers and DevOps, and be self-sufficient with data pipelines and utilize Delta and AutoML. Fivetran immediately stood out as a solution to Chris’ data pipeline needs. In trialing the product, the setup was quick and simple. Chris’s team now uses Fivetran to bring in data from all of their core marketing source systems: Marketo, Salesforce, Facebook Ads and Google Analytics. Once in their lakehouse, Chris’ team joins the data with other sources from Product and Central Teams, transforming it in a meaningful way to run Data Science, machine learning, and generate vital Tableau dashboards for analysis.
Operational Impact
Quantitative Benefit
Case Study missing?
Start adding your own!
Register with your work email and create a new case study profile for your business.
Related Case Studies.
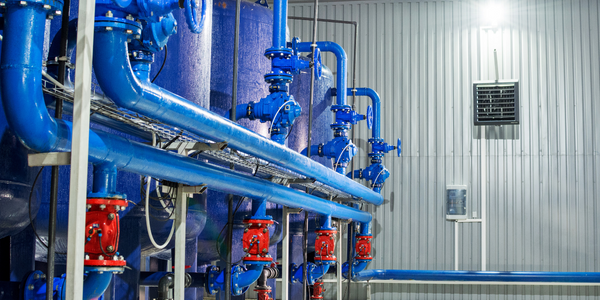
Case Study
Smart Water Filtration Systems
Before working with Ayla Networks, Ozner was already using cloud connectivity to identify and solve water-filtration system malfunctions as well as to monitor filter cartridges for replacements.But, in June 2015, Ozner executives talked with Ayla about how the company might further improve its water systems with IoT technology. They liked what they heard from Ayla, but the executives needed to be sure that Ayla’s Agile IoT Platform provided the security and reliability Ozner required.
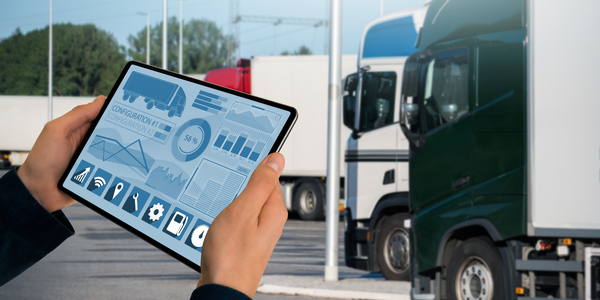
Case Study
IoT enabled Fleet Management with MindSphere
In view of growing competition, Gämmerler had a strong need to remain competitive via process optimization, reliability and gentle handling of printed products, even at highest press speeds. In addition, a digitalization initiative also included developing a key differentiation via data-driven services offers.
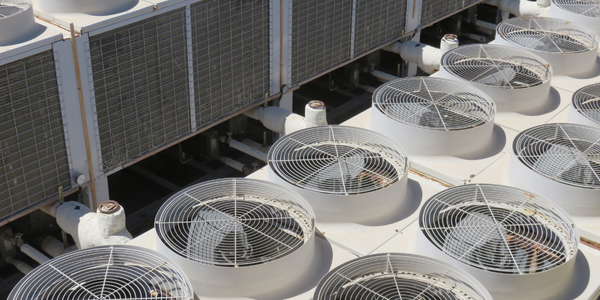
Case Study
Predictive Maintenance for Industrial Chillers
For global leaders in the industrial chiller manufacturing, reliability of the entire production process is of the utmost importance. Chillers are refrigeration systems that produce ice water to provide cooling for a process or industrial application. One of those leaders sought a way to respond to asset performance issues, even before they occur. The intelligence to guarantee maximum reliability of cooling devices is embedded (pre-alarming). A pre-alarming phase means that the cooling device still works, but symptoms may appear, telling manufacturers that a failure is likely to occur in the near future. Chillers who are not internet connected at that moment, provide little insight in this pre-alarming phase.
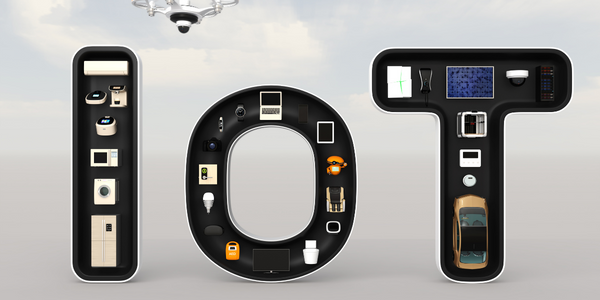
Case Study
Premium Appliance Producer Innovates with Internet of Everything
Sub-Zero faced the largest product launch in the company’s history:It wanted to launch 60 new products as scheduled while simultaneously opening a new “greenfield” production facility, yet still adhering to stringent quality requirements and manage issues from new supply-chain partners. A the same time, it wanted to increase staff productivity time and collaboration while reducing travel and costs.
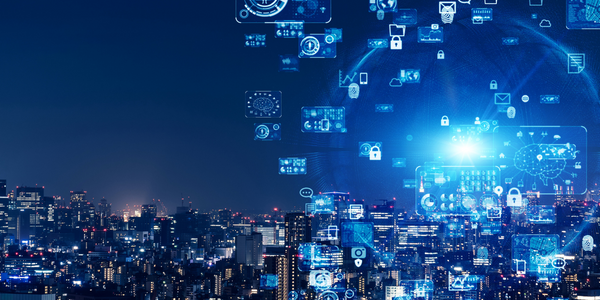
Case Study
Integration of PLC with IoT for Bosch Rexroth
The application arises from the need to monitor and anticipate the problems of one or more machines managed by a PLC. These problems, often resulting from the accumulation over time of small discrepancies, require, when they occur, ex post technical operations maintenance.
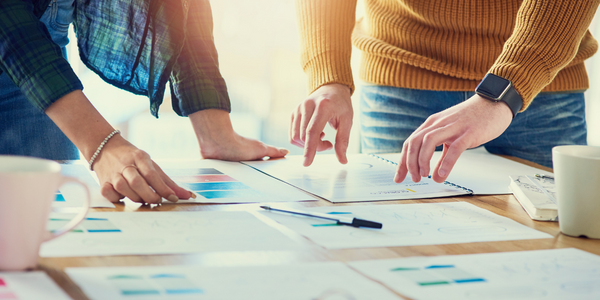
Case Study
Data Gathering Solution for Joy Global
Joy Global's existing business processes required customers to work through an unstable legacy system to collect mass volumes of data. With inadequate processes and tools, field level analytics were not sufficient to properly inform business decisions.