Technology Category
- Analytics & Modeling - Computer Vision Software
- Analytics & Modeling - Machine Learning
Applicable Industries
- Equipment & Machinery
- Plastics
Use Cases
- Predictive Maintenance
- Visual Quality Detection
Services
- Data Science Services
- Training
About The Customer
Kaleido AI is a tech company based in Vienna, Austria, with a mission to simplify complex technology. The company creates tools that simplify and accelerate workflows, foster creativity, and enable others to bring new ideas to life. It provides access to the latest advancements in visual AI to everyone, from individuals to businesses of all sizes. In 2019, Kaleido AI introduced remove.bg, an automatic image background remover, and in 2020, it launched Unscreen, an online software that removes video backgrounds. These tools dramatically increased the speed at which users could achieve their goals, leading to a surge in their popularity. This success led to Canva acquiring Kaleido AI in early 2021. Later that year, Kaleido AI launched Designify, an AI-powered tool that creates automatic designs for various users, including individuals, car dealerships, and e-commerce websites.
The Challenge
Kaleido AI, a Vienna-based company, is dedicated to simplifying complex technology by creating tools that accelerate workflows and foster creativity. The company introduced remove.bg, an automatic image background remover, and Unscreen, a video background remover, which gained immense popularity and led to its acquisition by Canva in 2021. However, Kaleido AI faced a significant challenge in improving its machine learning models. The company's models required a large volume of high-quality data, but they encountered several edge cases in a specific segmentation task where their model performed poorly. Collecting and labeling tens of thousands of real-world images with a large diversity of patterns, images, backgrounds, and textures was difficult. Open datasets did not have enough high-quality images of this particular class. Kaleido AI initially relied on real-world data to train its segmentation models, but this approach was complex, resource-intensive, and costly.
The Solution
To overcome the challenge, Kaleido AI turned to Scale AI for help in generating synthetic data to improve their model performance on object identification and improve the Intersection over Union (IoU) of their model predictions. Scale’s machine learning engineers analyzed Kaleido AI's sample data and model inferences in Nucleus, Scale’s data curation platform. They identified that the model was performing poorly in segmenting objects in images with complex patterns, shaded or transparent objects, or where there were shadows in the backgrounds of the scenes. Scale focused on these edge cases and generated a sample of 2,650 images of synthetic data with varied lighting, textures, and patterns. However, this first pass was not sufficient to meaningfully improve model performance. The Scale team then did a deep dive into Nucleus to curate data to further identify these problem edge cases. They also introduced the ability to visualize Scale’s synthetic images compared to real images in 2D space. This analysis revealed that Scale needed to include more textured/patterned objects and a wider variety of object types in the synthetic data distribution. In total, Scale generated 14,583 synthetic images covering a total of 12 categories covering patterns, various objects, backgrounds, and textures. With this targeted synthetic data, Kaleido AI achieved an IoU of 0.794.
Operational Impact
Quantitative Benefit
Case Study missing?
Start adding your own!
Register with your work email and create a new case study profile for your business.
Related Case Studies.
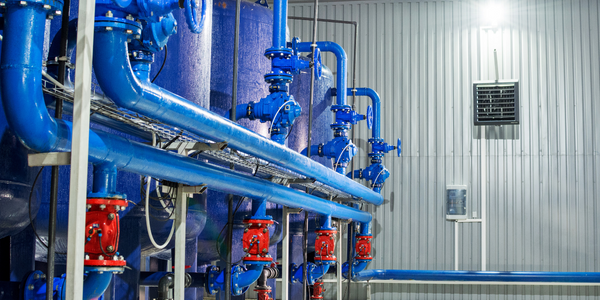
Case Study
Smart Water Filtration Systems
Before working with Ayla Networks, Ozner was already using cloud connectivity to identify and solve water-filtration system malfunctions as well as to monitor filter cartridges for replacements.But, in June 2015, Ozner executives talked with Ayla about how the company might further improve its water systems with IoT technology. They liked what they heard from Ayla, but the executives needed to be sure that Ayla’s Agile IoT Platform provided the security and reliability Ozner required.
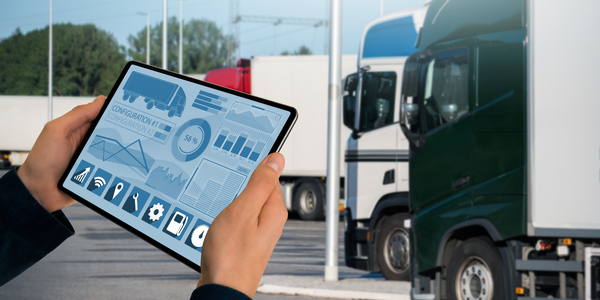
Case Study
IoT enabled Fleet Management with MindSphere
In view of growing competition, Gämmerler had a strong need to remain competitive via process optimization, reliability and gentle handling of printed products, even at highest press speeds. In addition, a digitalization initiative also included developing a key differentiation via data-driven services offers.
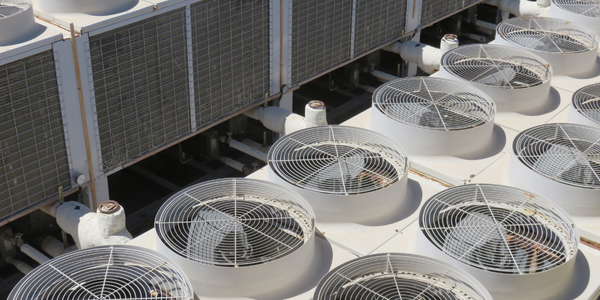
Case Study
Predictive Maintenance for Industrial Chillers
For global leaders in the industrial chiller manufacturing, reliability of the entire production process is of the utmost importance. Chillers are refrigeration systems that produce ice water to provide cooling for a process or industrial application. One of those leaders sought a way to respond to asset performance issues, even before they occur. The intelligence to guarantee maximum reliability of cooling devices is embedded (pre-alarming). A pre-alarming phase means that the cooling device still works, but symptoms may appear, telling manufacturers that a failure is likely to occur in the near future. Chillers who are not internet connected at that moment, provide little insight in this pre-alarming phase.
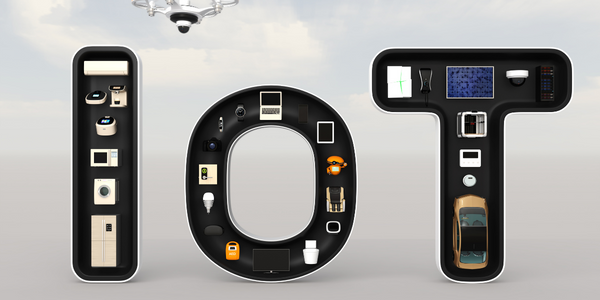
Case Study
Premium Appliance Producer Innovates with Internet of Everything
Sub-Zero faced the largest product launch in the company’s history:It wanted to launch 60 new products as scheduled while simultaneously opening a new “greenfield” production facility, yet still adhering to stringent quality requirements and manage issues from new supply-chain partners. A the same time, it wanted to increase staff productivity time and collaboration while reducing travel and costs.
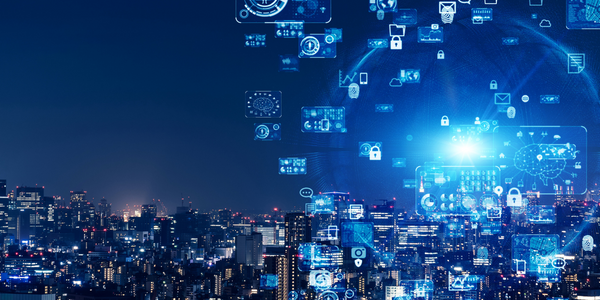
Case Study
Integration of PLC with IoT for Bosch Rexroth
The application arises from the need to monitor and anticipate the problems of one or more machines managed by a PLC. These problems, often resulting from the accumulation over time of small discrepancies, require, when they occur, ex post technical operations maintenance.
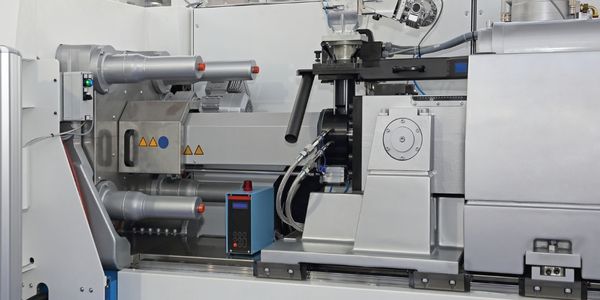
Case Study
Plastic Spoons Case study: Injection Moulding
In order to meet customer expectations by supplying a wide variety of packaging units, from 36 to 1000 spoons per package, a new production and packaging line needed to be built. DeSter wanted to achieve higher production capacity, lower cycle time and a high degree of operator friendliness with this new production line.