Technology Category
- Analytics & Modeling - Machine Learning
- Cybersecurity & Privacy - Security Compliance
Applicable Industries
- Automotive
- Equipment & Machinery
Applicable Functions
- Procurement
- Quality Assurance
Use Cases
- Leasing Finance Automation
- Personnel Tracking & Monitoring
About The Customer
The customer is a top financial services company that provides portfolio management, lending solutions, asset retention, and more, via proprietary underwriting and loan management technologies and systems. The company has a growing agent workforce and a limited QA staff. The company's agent teams are highly consultative, helping clients throughout the full process of loan procurement. Agents work with clients to sell loans, collect payments, and if needed, pursue collections. These multiple touchpoints provide opportunities for discovery and upsell on loan plans.
The Challenge
A leading financial services company was facing challenges in maintaining compliance and optimizing agent performance due to limited QA staff and increasing agent headcount. The company relied on spreadsheets and six-page QA evaluation forms to score and grade agent performance, which only allowed them to grade 10% of agent interactions each month. This limited QA coverage resulted in a lack of clear insights into how agents were successfully pitching lending solutions to clients, making it impossible to replicate successful strategies across their workforce. Additionally, as the company increased its agent headcount without growing its QA analyst headcount, it needed more compliance oversight. The challenge was to scale service, revenue, and performance while maintaining quality, compliance, and customer experience.
The Solution
The company adopted Observe.AI Auto QA, an AI and machine learning solution that automates the QA evaluation process. This allowed the company to increase QA volume and transform its agent performance improvement strategy to improve compliance and increase revenue growth across the business. The solution enabled the company to track agent performance and identify its top three QA attributes across all their calls, including monitoring agents for professionalism, agent disclosure of mandated compliance statements, and ensuring agents follow identity verification procedures. Observe.AI also helped the company unify their conversation analytics, QA evaluation process, and coaching workflows under one platform, reducing more than four different applications to one and cutting the time it takes to complete QA evaluations in half. The company was also able to optimize agent talk-tracks for revenue generation and close-rates, and gain 100% confidence in compliance.
Operational Impact
Quantitative Benefit
Case Study missing?
Start adding your own!
Register with your work email and create a new case study profile for your business.
Related Case Studies.
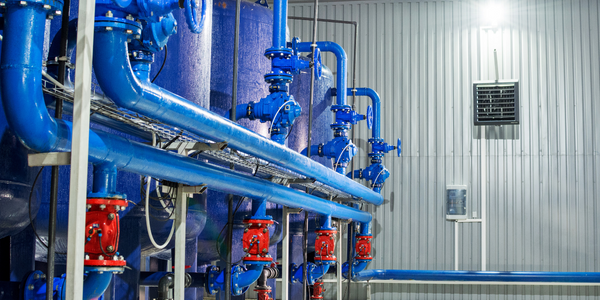
Case Study
Smart Water Filtration Systems
Before working with Ayla Networks, Ozner was already using cloud connectivity to identify and solve water-filtration system malfunctions as well as to monitor filter cartridges for replacements.But, in June 2015, Ozner executives talked with Ayla about how the company might further improve its water systems with IoT technology. They liked what they heard from Ayla, but the executives needed to be sure that Ayla’s Agile IoT Platform provided the security and reliability Ozner required.
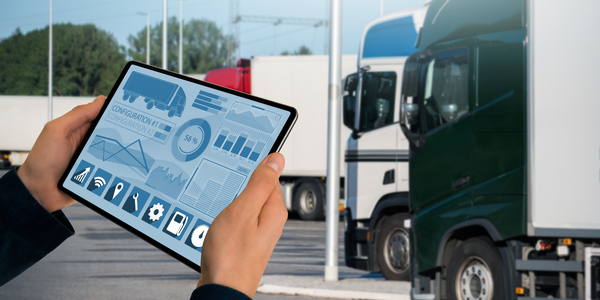
Case Study
IoT enabled Fleet Management with MindSphere
In view of growing competition, Gämmerler had a strong need to remain competitive via process optimization, reliability and gentle handling of printed products, even at highest press speeds. In addition, a digitalization initiative also included developing a key differentiation via data-driven services offers.
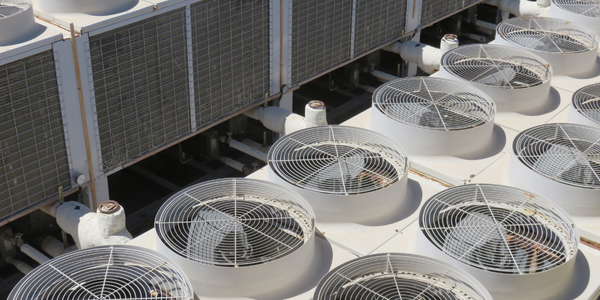
Case Study
Predictive Maintenance for Industrial Chillers
For global leaders in the industrial chiller manufacturing, reliability of the entire production process is of the utmost importance. Chillers are refrigeration systems that produce ice water to provide cooling for a process or industrial application. One of those leaders sought a way to respond to asset performance issues, even before they occur. The intelligence to guarantee maximum reliability of cooling devices is embedded (pre-alarming). A pre-alarming phase means that the cooling device still works, but symptoms may appear, telling manufacturers that a failure is likely to occur in the near future. Chillers who are not internet connected at that moment, provide little insight in this pre-alarming phase.
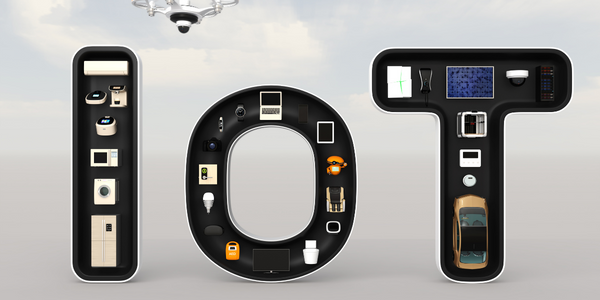
Case Study
Premium Appliance Producer Innovates with Internet of Everything
Sub-Zero faced the largest product launch in the company’s history:It wanted to launch 60 new products as scheduled while simultaneously opening a new “greenfield” production facility, yet still adhering to stringent quality requirements and manage issues from new supply-chain partners. A the same time, it wanted to increase staff productivity time and collaboration while reducing travel and costs.
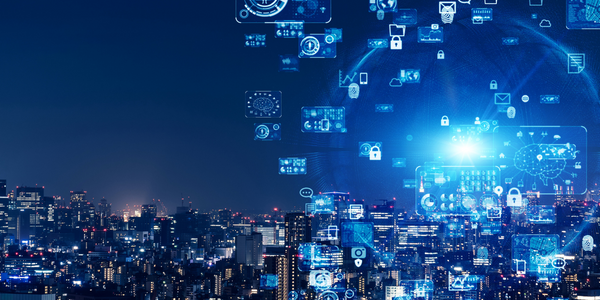
Case Study
Integration of PLC with IoT for Bosch Rexroth
The application arises from the need to monitor and anticipate the problems of one or more machines managed by a PLC. These problems, often resulting from the accumulation over time of small discrepancies, require, when they occur, ex post technical operations maintenance.
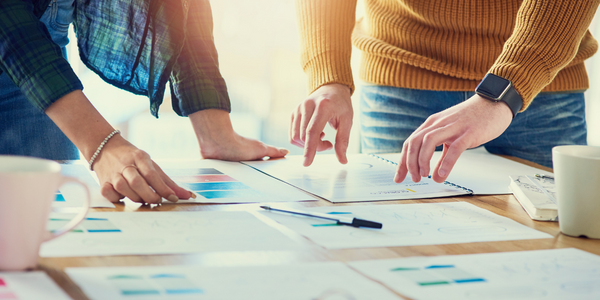
Case Study
Data Gathering Solution for Joy Global
Joy Global's existing business processes required customers to work through an unstable legacy system to collect mass volumes of data. With inadequate processes and tools, field level analytics were not sufficient to properly inform business decisions.