公司规模
Large Corporate
地区
- America
国家
- United States
产品
- AtScale
- Amazon Redshift
- Tableau
- Power BI
技术栈
- Cloud Data Management
- Data Warehousing
- Business Intelligence Tools
实施规模
- Enterprise-wide Deployment
影响指标
- Cost Savings
- Productivity Improvements
技术
- 分析与建模 - 实时分析
- 基础设施即服务 (IaaS) - 云计算
适用行业
- 汽车
适用功能
- 商业运营
- 离散制造
用例
- 工厂可见化与智能化
- 预测性维护
服务
- 云规划/设计/实施服务
- 数据科学服务
关于客户
Toyota is an international automotive company that was looking to consolidate 35+ constituent North American companies into a single structure. This required a transformation of their data warehousing and analytics architecture across the business. The company's IT department was tasked with creating a semantic layer that supported high performance analytics that could be leveraged by all business analyst teams. Prior to the project’s implementation, analysts would often need to wait weeks for manual data engineering to take place, hindering their ability to provide actionable insights on key business questions. The company's backend infrastructure was partly to blame for the slow query response time, as data was siloed across thousands of individual data marts.
挑战
Toyota, an international automotive company, was faced with the challenge of consolidating 35+ constituent North American companies into a single structure. This required a transformation of their data warehousing and analytics architecture across the business. The IT department was tasked with creating a semantic layer that supported high performance analytics that could be leveraged by all business analyst teams. Prior to the project’s implementation, analysts would often need to wait weeks for manual data engineering to take place. This delay hindered their ability to provide actionable insights on key business questions. The company's backend infrastructure was partly to blame for the slow query response time, as data was siloed across thousands of individual data marts.
解决方案
With AtScale’s semantic layer underpinning their efforts, Toyota's IT department migrated data from a variety of legacy tools to an Amazon Redshift data warehouse. Once their data was in the warehouse, they could leverage AtScale to enable multidimensional modeling of their data, allowing them to connect their Redshift data warehouse on the back end with data visualization tools like Tableau and PowerBI on the front end. With AtScale, Toyota was able to scale its analytics program to support thousands of user using a range of BI tools. This eliminated the friction associated with moving thousands of SQL queries into a single data warehouse, enabling better user access to data. This also made improved governance possible, keeping data more secure as well as ensuring that various definitions were uniform and accurate across the company.
运营影响
数量效益
Case Study missing?
Start adding your own!
Register with your work email and create a new case study profile for your business.
相关案例.
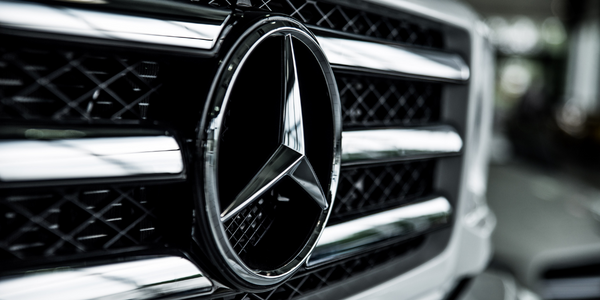
Case Study
Integral Plant Maintenance
Mercedes-Benz and his partner GAZ chose Siemens to be its maintenance partner at a new engine plant in Yaroslavl, Russia. The new plant offers a capacity to manufacture diesel engines for the Russian market, for locally produced Sprinter Classic. In addition to engines for the local market, the Yaroslavl plant will also produce spare parts. Mercedes-Benz Russia and his partner needed a service partner in order to ensure the operation of these lines in a maintenance partnership arrangement. The challenges included coordinating the entire maintenance management operation, in particular inspections, corrective and predictive maintenance activities, and the optimizing spare parts management. Siemens developed a customized maintenance solution that includes all electronic and mechanical maintenance activities (Integral Plant Maintenance).
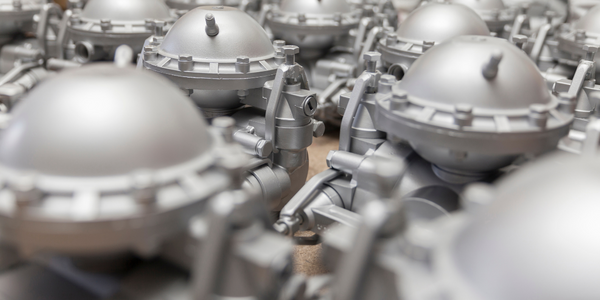
Case Study
Monitoring of Pressure Pumps in Automotive Industry
A large German/American producer of auto parts uses high-pressure pumps to deburr machined parts as a part of its production and quality check process. They decided to monitor these pumps to make sure they work properly and that they can see any indications leading to a potential failure before it affects their process.