公司规模
Large Corporate
地区
- America
国家
- United States
产品
- H2O
技术栈
- Hadoop
- R
- Java
实施规模
- Enterprise-wide Deployment
影响指标
- Cost Savings
- Productivity Improvements
技术
- 分析与建模 - 大数据分析
- 分析与建模 - 预测分析
适用功能
- 商业运营
用例
- 欺诈识别
服务
- 数据科学服务
关于客户
The customer is a global insurance company that is seeking to detect and prevent claims fraud in its Workman's Compensation business. The company has a growing business and needs a more automated approach to handle the increasing volume of claims. The company has data scientists who use R for advanced analytics, but they were facing challenges in scaling their analytics to handle Hadoop-level data volumes. The company needed a solution that could provide sophisticated predictive analytics on large datasets and enable rapid deployment of fraud detection models.
挑战
The insurance company was facing a significant challenge with claims fraud, which is estimated to cost the industry $80 billion annually in the United States alone. The existing process for detecting suspicious claims was entirely manual, relying on the judgment and experience of professional claims examiners. This approach was not scalable for a growing business and was time-consuming due to the need to pull information from multiple systems. The company had consolidated data from various sources into a Hadoop data store, which included a mix of structured and unstructured data. However, Hadoop lacked the capability for sophisticated predictive analytics, and extracting the data to an analytic server was time-consuming.
解决方案
The company implemented H2O, an open-source machine learning platform, to address its challenges. H2O was co-located in the company's Hadoop cluster, allowing analysts to discover insights in the data without extracting it or taking samples. Data scientists could interact with H2O using R, but all of the work was performed in H2O where it was deployed, in the Hadoop cluster. This approach enabled the company to leverage its large datasets for predictive analytics. When an analytics project was completed, H2O exported predictive models as Plain Old Java Objects (POJOs). These POJOs could run anywhere in the organization that Java runs, enabling rapid deployment of fraud detection models in various systems.
运营影响
数量效益
Case Study missing?
Start adding your own!
Register with your work email and create a new case study profile for your business.
相关案例.
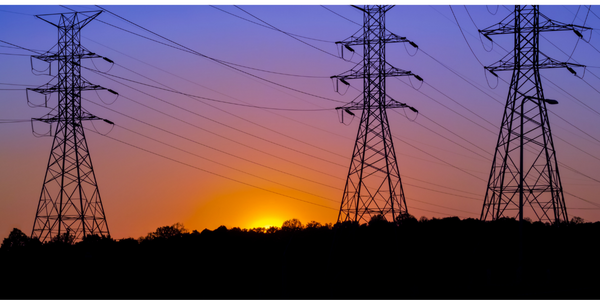
Case Study
Largest Production Deployment of AI and IoT Applications
To increase efficiency, develop new services, and spread a digital culture across the organization, Enel is executing an enterprise-wide digitalization strategy. Central to achieving the Fortune 100 company’s goals is the large-scale deployment of the C3 AI Suite and applications. Enel operates the world’s largest enterprise IoT system with 20 million smart meters across Italy and Spain.
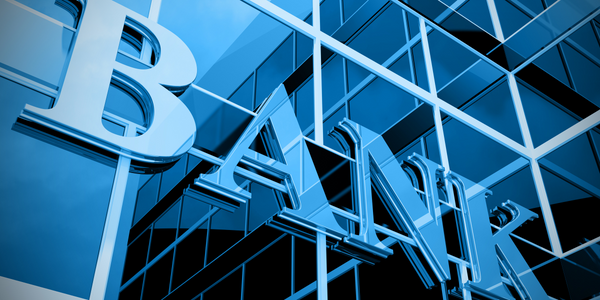
Case Study
KeyBank's Digital Transformation with Confluent's Data in Motion
KeyBank, one of the nation's largest bank-based financial services companies, embarked on a national digital bank initiative following the acquisition of Laurel Road, a digital consumer lending business. The initiative aimed to build a digital bank focused on healthcare professionals looking to refinance student loans and buy homes. A significant challenge was reducing the time to market for new products by democratizing data and decoupling systems across the IT landscape. Like many large enterprises, KeyBank had a variety of vendor applications, custom applications, and other systems that were tightly coupled to one another. New projects often required developing specific point-to-point integrations for exchanging data, which did not address the needs of other downstream systems that could benefit from the same data.
Case Study
BharatPe: Leveraging Google Cloud for Enhanced Data Analytics and AI to Promote Digital Payments
BharatPe, a fintech company founded in 2018, aimed to make digital payments more accessible for over 10 million small offline merchants and kirana store owners in India. However, the company faced challenges in managing the massive amounts of data generated daily from payment processing to business analysis. Prior to using Google Cloud, BharatPe managed its legacy data warehouse with limited capacity to run a large number of queries. The company ran key performance indicator (KPI) reports, without the ability to understand real-time data patterns. Loading three months of data for quarterly reports took more than 30 minutes on the legacy system, and in some cases, queries failed because the system could not scale to support analytical needs. Additionally, BharatPe operates in a multi-cloud environment for disaster recovery and needed a data platform that could run queries against data, regardless of where it resides.
Case Study
Barclays Enhances Customer Experience with IBM BPM Solution
Barclays, a global bank operating in over 50 countries and serving nearly 60 million customers, faced a significant challenge in improving and streamlining the customer experience. The bank's relationships with customers were multifaceted, with customers engaging with the bank through various channels such as mobile, online, and branch locations. The bank had identified 400 different customer journeys, and the challenge was to align these across the enterprise and improve them in a way that positively impacted customers quickly. The director of operations at Barclays, Mike Gamble, recognized the need to transform the bank's processes around customer journeys. He aimed to enhance the understanding of how various functions contribute to delivering customer experiences and then expedite the rollout time of these new processes to achieve the end goal for the customer.
Case Study
Bank BRI: Revolutionizing Financial Inclusion in Asia with Digital Banking
Bank Rakyat Indonesia (Bank BRI), one of the largest banks in Indonesia, was faced with the challenge of increasing financial inclusion among unbanked Indonesians. The bank had an ambitious target of having 84 percent of Indonesians participating in the banking system by 2022. However, the bank's legacy technologies were proving to be a hindrance in achieving this goal. Each of the bank's products had their own public APIs, which were difficult to manage, secure, and monetize. Additionally, the process of onboarding new partners using host-to-host and VPN technology was time-consuming, taking up to six months. The bank also faced the challenge of reaching a largely rural population, with an estimated $8.3 billion in currency being held outside the banking system.
Case Study
Stabilizing an Avalanche of Data: Comcast's Journey with Data360 Analyze
Comcast Corporation, the second largest broadcasting and cable television company in the world by revenue, was facing significant challenges due to the volume and variety of operational and billing data. With over 20 million customers across 40 states, the company needed to establish standardized processes and automate data flows to overcome a wide range of billing, finance, compliance, supply chain, and marketing challenges. In 2009, the company was dealing with massive amounts of data that were causing major revenue assurance challenges. The customer billing review interface required the processing of a billion records every month, within very tight timeframes. The company’s decentralized accounting and finance operations, with 20 different regions and 30 different accounting practices, further compounded these issues.