AI for manufacturing - Chinese market AI adoption trends
Over the past several years, the global manufacturing industry has experienced a trade war, Brexit, a global pandemic, and now war in Europe. These supply and demand shocks have forced manufacturers to manage a series of supply chain, labor shortage and inflation challenges. In response, companies have accelerated investment in digital transformation as they seek to improve agility and cost competitiveness. This trend is particularly important in China, as manufacturing contributes 26% of GDP, equaling over $4 trillion dollars.
Global Manufacturing AI Revenue
Million U.S Dollars
China Manufacturing AI Revenue2
Million U.S Dollars
Artificial intelligence (AI) technologies are a critical component of the manufacturing digital transformation. AI is a branch of computer science that involves building systems capable of performing tasks that typically require human intelligence. AI systems train algorithms with data to solve a wide range of problems, from optimizing production scheduling to identifying product defects. Traditionally, AI systems have run on cloud infrastructure due to their high computing requirements, but a new generation of edge computing hardware is bringing AI to the factory floor. | ![]() |
While the first large scale AI use cases, such as Google search, relied on ‘big data’, industrial AI solutions often rely on small data sets. Although manufacturing data is enormous in aggregate, each AI deployment may only access data from a few dozen machines. When big data is unavailable, high-quality data becomes critical. Special machine learning (ML) algorithms are also being designed for tasks such as machine vision and predictive maintenance, with the goal of improving the accuracy level that can be achieved with a limited data set.
Industrial AI applications tend to be very narrow, meaning they focus on realizing one specific activity. The objectives of AI solutions can be categorized into three groups: managing product complexity, process complexity and value chain complexity. For example, growing demand for mass customization requires systems capable of making rapid production planning decisions dynamically without human input.
- Product complexity: AI enables companies to manufacture sophisticated products more efficiently. For example, each automobile contains about 30,000 components, all of which are separately produced and assembled into a single unit.
- Process complexity: The process to assemble an automobile includes hundreds of discrete actions, from component assembly to painting, to quality assurance. Manufacturers are using AI to simulate human cognitive abilities such as logical reasoning and visual perception to optimize processes.
- Value chain complexity: The Internet of Things allow companies to assemble real time data from dozens of sources to help predict supply chain disruptions that would impact production. As COVID closed factories and ports, these capabilities helped manufacturers forecast and manage logistical disruptions.
Selection of the best AI solution for a specific task depends on multiple technical and business factors. Every system must account for the three key technical considerations: (1) the availability of data sources, (2) the effectiveness of alternative algorithms, and (3) the system architecture that connects data collection, storage, processing, and outcome reporting. These technical constraints interact with business requirements, user experience requirements, and the organization’s technical or physical constraints.
The first step in deploying AI solutions into the real world is precisely documenting user requirements. Clarification is critical since every business requirement will impact the technical solution. Although this appears to be straightforward, communication gaps between business and technical teams are a common source of project failure.
Significant implementation challenges also exist in the data preparation, model training, and AI production stages of the AI value chain. Most manufacturers do not have access to the ideal data set. This could be due to a lack of sufficient data, human error in data collection, or the effort required to process data for use.
To help businesses understand how AI can be applied in the manufacturing sector, we have identified use cases for nine core manufacturing functions.
- Demand planning: AI enables organizations to optimize product availability by decreasing out of stocks and spoilage. It also can be used to project sales patterns. L’Oreal built AI algorithms to predict demand based on a wide variety of data gathered from social media, weather, and financial markets.
- Product Development: Organizations can expediate product development by deriving concrete insights from customer data and demands. Nissan is in the test phase of using AI to design its cars. To comply with new regulations, algorithms are used to calculate the knock-off effect on modifications to existing models when designing upgrades.
- Production: The required product assembly time that is needed to match demand (known as TAKT) can be reduced by using algorithms to streamline manufacturing processes to increase output. Mitsubishi Electric automated calculation of the rate, speed, and acceleration of their industrial robots to achieve a 10% reduction in TAKT.
- Process control: AI can help organizations optimize processes to achieve production levels with enhanced consistency, economy, and safety. BMW leverages AI to identify recurring patterns in a process to increase hourly output in the press shop.
- Quality control: Machine vision is widely used in product quality inspections to improve uniformity, speed and cost efficiency. Audi installed an image recognition system based on deep learning at its Ingolstadt press shop to identify even the finest cracks on metal sheets.
- Maintenance: Algorithms can predict asset failure to reduce downtime by analyzing data prior to earlier equipment failures. General Motors analyzes sensor on motors and servos together with operational data to spot early signs of failure in industrial robots.
- Inventory management: Predictive systems can also be used to track real time inventory levels and consumption rates, enabling organizations to optimize inventory levels while avoids stockouts.
- Energy management: Because energy consumption is a continuous process, it is one of the easiest production areas to optimize with machine learning algorithms. Identifying waste can both reduce energy bills and help companies meet sustainability targets.
- Environment, health, and safety (EHS): AI systems can be used to both track risk factors and to automate response mechanism in the case of a health and safety event.
POV: Asia Digital Landscape – AI adoption in China
The Chinese market has made incremental progress in AI adoption in manufacturing in recent years. However, China’s adoption rate remains low with an average of 9% according to the 2021 China Industrial Application of AI report by Lead Leo. Electronic, automotive, and petrochemical are the top 3 industries by adoption. The low adoption rate is due both to the immaturity of the domestic industrial AI supplier base, as well as the relatively low rate of process standardization at manufacturers. China also has a much lower labor cost than advanced economies, which impacts the business case for capital investment in sensors, robotics, and algorithm training.
Beyond manufacturing, China has established itself as the second most prominent AI ecosystem, ranking after the United States in all categories except for papers and citations. The market for AI applications is advancing rapidly and enjoys governmental support.
Total Estimated Investments in AI Start-ups ($ billion) | 2021 Artificial Intelligence Index |
Source: Crunchbase, Stanford University
China’s industrial AI market is expected to grow rapidly due to improving conditions in the political, economic, social, and technological domains. The government is encouraging development with a number of policy initiatives. For example, China was the first country to allow the filing of AI patents. Strong access to finance has also contributed to China becoming the second largest base of AI solution vendors after the US. AI research is typically published openly, allowing industry players to learn from one another and rapidly improve product-market fit. The local data science talent base is also growing rapidly due China’s decades-long effort to improve STEM education.
Foreign firms in China should be aware of the practical challenges presented by China’s AI ecosystem. Unlike in Western economies, most AI patents in China are filed by universities and research institutes. University-industry linkages are relatively weak, and technology transfer remains limited. In addition, the uncertainty of the business environment incentivizes companies and investors to favor applied AI research that can bring quick revenue, rather that investing in basic research. As a result, there are many “me too” business models that are built on open-source technologies with relatively weak competitive barriers and breakthroughs in core technologies are rare. The significant gray area in Chinese privacy policy also presents a risk, leading many companies to postpone initiatives until the regulatory situation is clarified.
As with any emerging digital technology, the rapid pace of development presents opportunities and risks. Companies that postpone development of local AI capabilities in China may hope to mitigate learning costs and risks and later enter with a fast follower strategy. However, gaining the organizational competence to identify requirements, collect data, and generate meaningful insights is time consumptive and could lead to a lengthy gap in capabilities as first movers capture market share. We recommend investing early but conservatively to build competence and lay a foundation for rapid technology adoption as significant business opportunities are identified.
IoT ONE Insights
Download PDF.
Your PDF report was sent successfully to your inbox!
Related Insights.
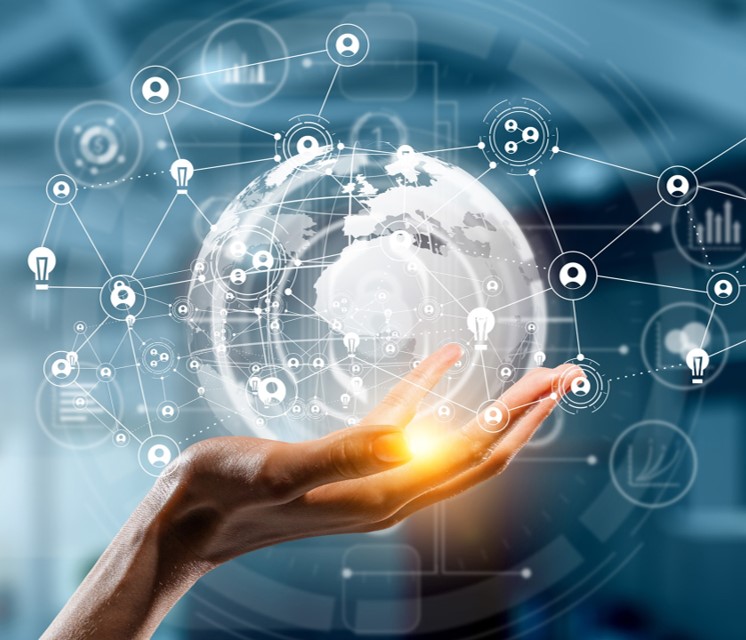
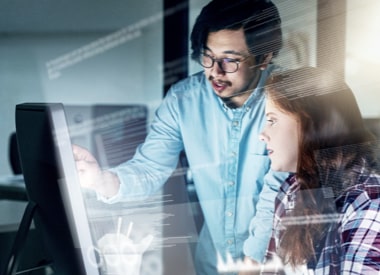
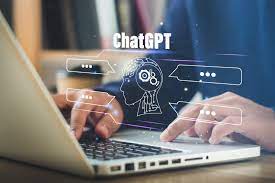
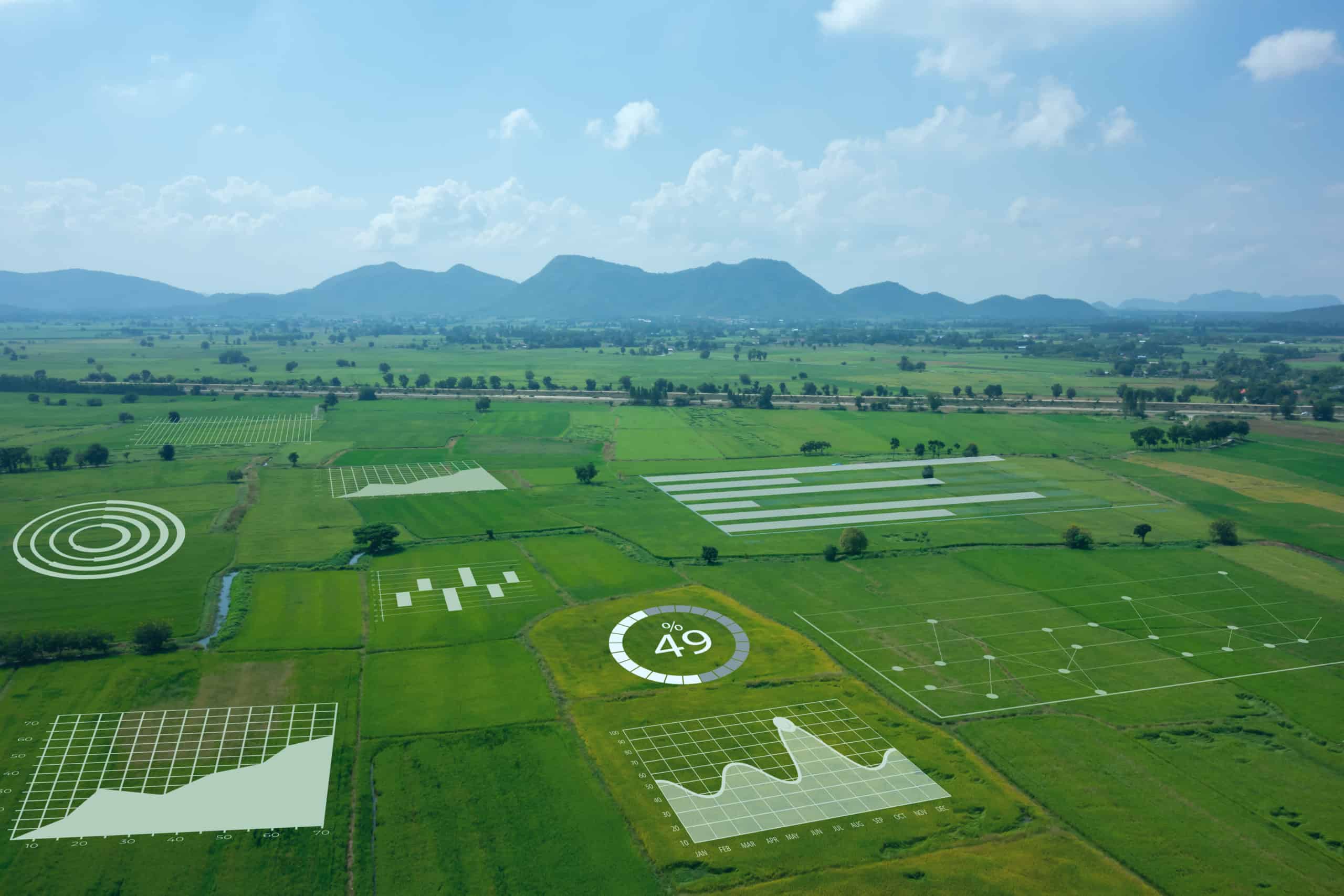
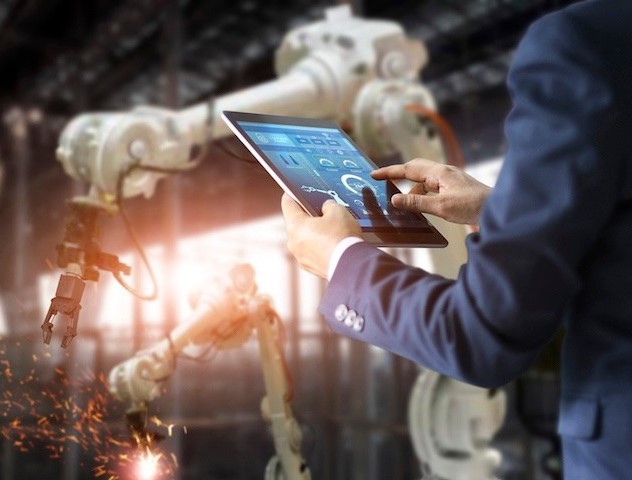