雾计算
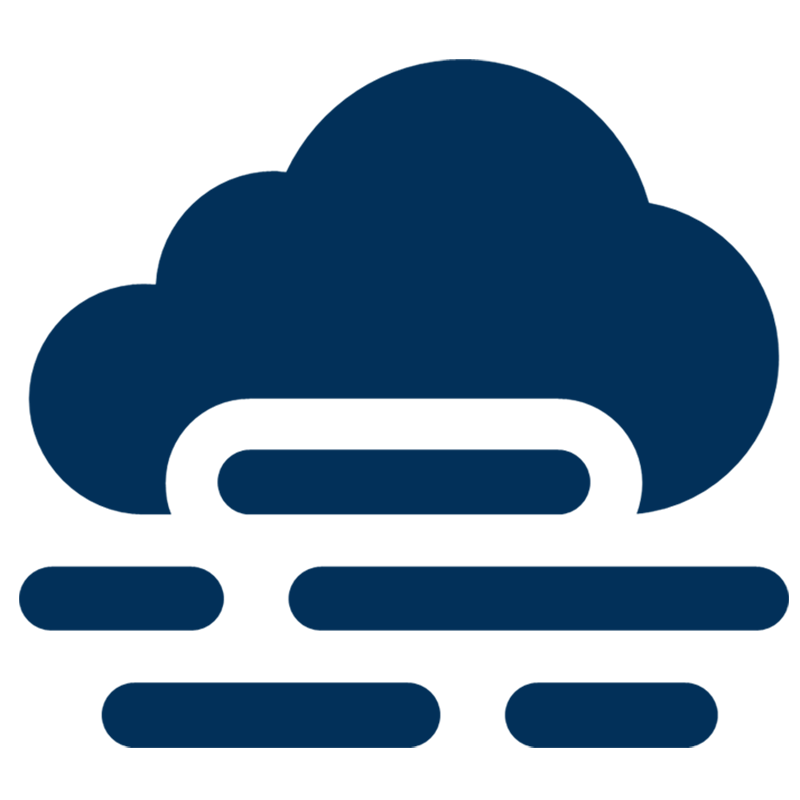
概述
雾计算是指一种去中心化的计算结构,其中包括数据和应用程序在内的资源被放置在数据源和云之间的逻辑位置;它也被称为雾化和雾网络。这样做的目的是将基本的分析服务带到网络边缘,通过将计算资源定位在更靠近需要的地方来提高性能,从而减少数据需要在网络上传输的距离,从而提高整体网络的效率和性能。出于安全原因,也可以部署雾计算,因为它能够分割带宽流量并为网络引入额外的防火墙以提高安全性。
适用行业
- 设备与机械
适用功能
- 离散制造
- 物流仓储
市场规模
商业观点
雾计算对公司有什么价值?
通过增加在更接近创建位置处处理数据的能力,雾计算旨在创建一个延迟更低、上传数据更少的网络,从而提高处理效率。
还有一个好处是,在没有可用带宽的情况下,仍然可以使用雾计算处理数据。它在这些物联网设备和它们连接的云计算基础设施之间提供了一个中介,因为它能够分析和处理更接近数据来源的数据,过滤上传到云端的数据。
雾计算在实时应用中有什么好处?
它广泛用于涉及实时数据的物联网应用。它充当云计算的扩展版本。它是云和最终用户(更接近最终用户)之间的中介。它可以以两种方式使用,既可以在机器与机器之间,也可以在人与机器之间。
- 移动大数据分析
- 大坝的水压
- 智能公用事业服务
- 健康数据
部署挑战
雾计算的主要挑战是什么?
安全挑战在雾计算中占主导地位。
雾计算考虑 SOA 的架构。网络层建立在服务层和应用层之间。因此,雾计算是在传统网络组件之前设计的,传统网络组件是极易受到攻击的安全攻击。
案例研究.
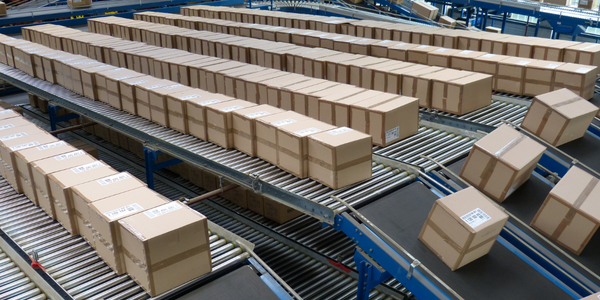
Case Study
IoT Data Analytics Case Study - Packaging Films Manufacturer
The company manufactures packaging films on made to order or configure to order basis. Every order has a different set of requirements from the product characteristics perspective and hence requires machine’s settings to be adjusted accordingly. If the film quality does not meet the required standards, the degraded quality impacts customer delivery causes customer dissatisfaction and results in lower margins. The biggest challenge was to identify the real root cause and devise a remedy for that.
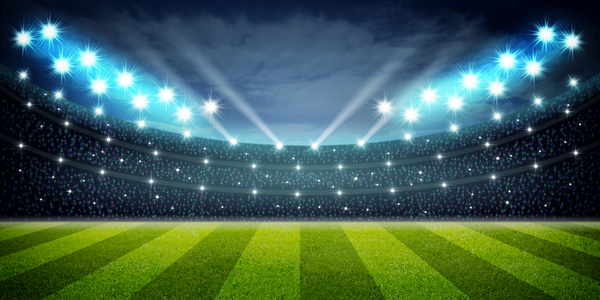
Case Study
Engaging Fans at one of the Largest Stadiums in the USA
Engaging and delighting fans has become the number one priority. However, the identity and behavior of fans within the stadium have been impossible to detect until Sirqul. Furthermore, standalone mobile apps historically have only seen a 5-30% penetration rate, thus leaving venues with poor data, insights and little interaction with the fan itself.This large stadium was looking for a set of recommendations to improve the fan experience, increase revenue and optimize operational efficiency based on this never seen before data.
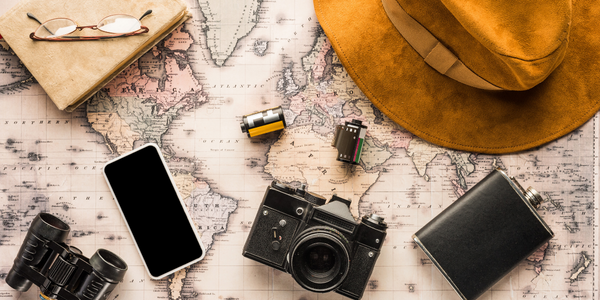
Case Study
Expedia Hosted by 2lemetry Through AWS
Expedia is committed to continuous innovation, technology, and platform improvements to create a great experience for its customers. The Expedia Worldwide Engineering (EWE) organization supports all websites under the Expedia brand. Expedia began using Amazon Web Services (AWS) in 2010 to launch Expedia Suggest Service (ESS), a typeahead suggestion service that helps customers enter travel, search, and location information correctly. According to the company’s metrics, an error page is the main reason for site abandonment. Expedia wanted global users to find what they were looking for quickly and without errors. At the time, Expedia operated all its services from data centers in Chandler, AZ. The engineering team realized that they had to run ESS in locations physically close to customers to enable a quick and responsive service with minimal network latency.