公司规模
Large Corporate
地区
- America
国家
- United States
产品
- SemanticPro Extract & Analyze
技术栈
- Machine Learning
- Natural Language Processing
实施规模
- Enterprise-wide Deployment
影响指标
- Productivity Improvements
- Revenue Growth
技术
- 分析与建模 - 自然语言处理 (NLP)
- 分析与建模 - 机器学习
适用功能
- 销售与市场营销
用例
- 自动化疾病诊断
服务
- 数据科学服务
关于客户
客户是一家大型美国媒体集团,旗下拥有 40 多家出版物。他们与不同的代理商合作销售广告空间,每周收到大约 100 个请求,相当于每年约 5,000 条消息和两倍的附件。这些提案请求 (RFP) 需要根据垂直市场或目标受众等标准进行分类,然后才能发送到相应的部门。该公司正在寻找一种解决方案来自动化此过程,同时保持可接受的准确度。
挑战
该媒体公司拥有 40 多家出版物,每周收到大约 100 个请求,相当于每年约 5,000 条消息和两倍多的附件。这些提案请求 (RFP) 需要根据垂直市场或目标受众等标准进行分类,然后才能发送给相应的部门。挑战在于 RFP 是高度非结构化的文档,格式多种多样,例如电子邮件正文或 Word 或 Powerpoint 中的附件。提取目标非常多样化,在大多数情况下,可供学习的背景非常少,这使得最先进的机器学习系统很难提供令人满意的结果。
解决方案
该公司实施了 SemanticPro Extract & Analyze,这是 Cortical.io 开发的一种解决方案,可以自动从请求中提取、审查和分析关键数据,并且精度很高。该解决方案能够处理没有标点符号的短文本,即使上下文很少或没有上下文,以及不同类型的文档,如 Word、Excel 或 Powerpoint。该解决方案已成功训练,可以识别非常多样化的提取目标,如“活动名称”、“客户”和“代理”,以及非常具体的词汇,如“广告产品类型”。提取结果可以根据用户的喜好以不同的格式或数据库无缝导出。生产阶段完成后,该公司计划实施分类和路由解决方案,将传入的电子邮件路由到相应的部门。
运营影响
数量效益
Case Study missing?
Start adding your own!
Register with your work email and create a new case study profile for your business.
相关案例.
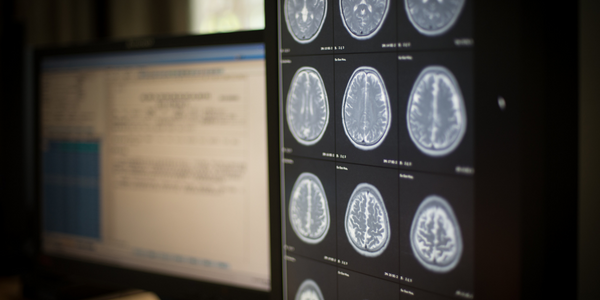
Case Study
Artificial Intelligence and the implications on Medical Imaging
There are several factors simultaneously driving integration of AI in radiology. Firstly, in many countries around the world there is a discrepancy between the number of doctors trained in radiology and the rising demand for diagnostic imaging. This leads to greater demands for work efficiency and productivity. For example, the number of radiology specialists (consultant work- force) in England went up 5% between 2012 and 2015, while in the same period the number of CT and MR scans increased by 29 and 26 percentage points respectively. In Scotland, the gap widened even further (The Royal College of Radiologists 2016). Today, the average radiologist is interpreting an image every three to four seconds, eight hours a day (Choi et al. 2016).Secondly, the image resolution of today’s scanners is continuously improving – resulting in an ever greater volume of data. Indeed, the estimated overall medical data volume doubles every three years, making it harder and harder for radiologists to make good use of the available information without extra help from computerized digital processing. It is desirable, both in radiological research and in clinical diagnostics, to be able to quantitatively analyze this largely unexploited wealth of data and, for example, utilize new measurable imaging biomarkers to assess disease progression and prognosis (O’Connor et al. 2017). Experts see considerable future potential in the transformation of radiology from a discipline of qualitative interpretation to one of quantita- tive analysis, which derives clinically relevant information from extensive data sets (“radiomics”). “Images are more than pictures, they are data,” American radiologist Robert Gillies and his colleagues write (Gillies et al. 2016). Of course, this direction for radiology will require powerful, automated procedures, some of which at least will come under the field of artificial intelligence.
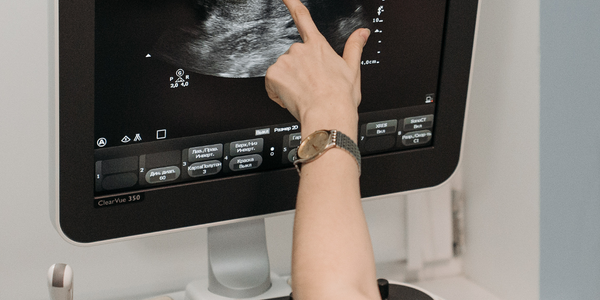
Case Study
Improving Diagnosis Accuracy and Saving Lives
Dr. Partho Sengupta needed a way to accurately identify disease patterns resulting from echocardiograms in order to improve diagnostics and save more lives. Specifically, he wanted to distinguish between two disparate diseases: cardiomyopathy, which directly impacts the heart muscle and often leads to heart failure, and pericarditis, which acts as if the heart is involved but doesn’t actually affect the heart. While both diseases present with similar heart conditions, the treatments are vastly different. For pericarditis, the treatment may include medication and, rarely, surgery. However, if the diagnosis is cardiomyopathy the patient undergoes medical management (i.e. a pacemaker) or in extreme cases, a heart transplant. Misdiagnosis of these disease conditions can put the patient’s life at risk and be very expensive for the hospital. Dr. Sengupta, therefore, looked to Saffron’s Natural Intelligence Platform to help his team increase the diagnosis accuracy of these medical conditions.
Case Study
Population Health Management Platform Anchors Arch Health’s Approach to Lowering Hypertension
Arch Health Partners, a multi-specialty group with over 90 providers at 13 locations in San Diego County, was facing a challenge with its hypertensive patient population. The group's hypertension statistics were below their expectations, and they were struggling to improve the health of these patients. The first step in driving better outcomes was identifying at-risk patients from within a large and growing patient population, which proved to be a more difficult challenge than originally expected. As the organization continued to grow, managing clinical data and improving quality scores became increasingly difficult due to manual processes. The organization realized that to increase the quality and frequency of patient engagement, they would need to automate some of their processes.
Case Study
HeartFlow's Transformation of Heart Disease Diagnosis and Treatment with AWS
HeartFlow, a medical technology company, is revolutionizing the diagnosis and treatment of heart disease with its non-invasive HeartFlow FFRct Analysis. This technology uses deep learning to create a personalized 3D model of the heart, allowing clinicians to better evaluate the impact of a blockage on blood flow and determine the best treatment. However, the company faced a significant challenge. Cardiovascular disease, the world’s leading cause of death, claims more than 17 million lives each year. The most common type, coronary artery disease (CAD), reduces blood flow to the heart, causing chest pain, heart attack, and death. Clinicians need to know if and where there is a blockage, and how it is affecting blood flow. This information is crucial in choosing the best treatment pathway for the patient, such as medical management, stenting or bypass surgery. However, the diagnostic coronary angiogram often used to detect CAD is invasive, expensive, and potentially risky. More than half of patients who undergo the test have no significant blockages, and the procedure can be associated with serious complications.
Case Study
Adaptive Biotechnologies Leverages Azure for Immune Medicine Platform
Adaptive Biotechnologies, a commercial-stage biotechnology company, has been working on harnessing the biology of the adaptive immune system to transform disease diagnosis and treatment. The company has built a proprietary immune medicine platform that decodes the genetic language of the adaptive immune system. However, the challenge was to synthesize this vast system of biology and tap into the full value of the massive clinical immunomics database generated. The company needed high-scale compute resources and machine learning capabilities to unlock the full potential of the research data. The immune system's complexity and the sheer volume of data generated by Adaptive’s dynamic clinical immunomics database, which includes more than 47 billion immune receptors, posed significant challenges.
Case Study
Leveraging IoT in Biomedical Research: A Case Study of Scripps Clinic's Orthopedic Implants
The Scripps Clinic's Shiley Center for Orthopedic Research and Education (SCORE) was faced with the challenge of improving the understanding and design of orthopedic implants, specifically for shoulder arthroplasty. The existing implants, typically made of titanium alloy and lined with plastics, were not expected to last more than 20 years, making them unsuitable for patients under 65. Furthermore, patients often had concerns about the range of motion, strength recovery, and longevity of the new joint. The process of modeling replacements for the meniscus, a crescent-shaped knee cartilage, was also a complex and time-consuming task, requiring the team to start the modeling process from scratch each time they wanted to change the curvature or thickness.