公司规模
Mid-size Company
地区
- Asia
国家
- India
产品
- Verloop.io
- AI-powered FAQ builder
技术栈
- Conversational AI
- AI-powered Chatbot
实施规模
- Enterprise-wide Deployment
影响指标
- Customer Satisfaction
- Productivity Improvements
技术
- 功能应用 - 远程监控系统
- 分析与建模 - 机器学习
用例
- 对话机器人
服务
- 软件设计与工程服务
- 系统集成
关于客户
Founded in 2012, Scripbox is India’s leading digital wealth manager. It understands customer needs in the context of their life and wealth stages, providing customized investment solutions to help them meet financial goals. Scripbox uses data, technology, and proprietary algorithms to deliver a full-stack of wealth management solutions, including mutual funds, Indian and International equities, insurance, holistic portfolio construction, and financial advisory. The company is headquartered in Bengaluru, India, and operates in the banking and financial services industry. Scripbox aims to provide seamless and efficient customer service, addressing the growing number of support queries related to investments and withdrawals.
挑战
There are more than 10,000 support tickets that come to Scripbox on a monthly basis. Most queries are about investments and withdrawals, with customers wanting to know about new investment products, the status of their investments, and the purpose of investment. The number of chats has been growing month-on-month for the last 2 years. Customers expect immediate, accurate responses with no wait time, putting immense pressure on agents. More than 50% of queries are received via chat, 35% over calls, and 15% via email. Scripbox needed to strengthen their chat platform to handle queries efficiently and on time. Previous tools failed to handle the complexity of the query flow, leading to a decision to use Verloop.io.
解决方案
Scripbox chose Verloop.io, a platform using conversational AI to solve customer support issues. Verloop.io's product understands each query uniquely, making conversations contextual, relevant, and meaningful. Scripbox aimed to automate responses for frequently asked queries, which were standard but took up agents’ bandwidth due to volume. Verloop.io’s FAQ builder made it simple to add responses to standard queries and keywords. The AI is pre-trained to understand various intents, including sentences with emojis, and is further trained on specific use cases Scripbox encounters regularly. The AI-powered FAQ builder understands the intent behind queries and sends automated responses, eliminating wait times and errors. Verloop.io enabled Scripbox to design multiple bot flows, reducing response times and handling nearly 70% of queries with the bot, enhancing customer satisfaction.
运营影响
数量效益
Case Study missing?
Start adding your own!
Register with your work email and create a new case study profile for your business.
相关案例.
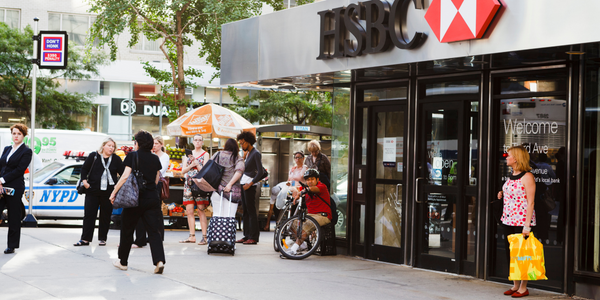
Case Study
HSBC's Transition to Conversational Banking through Intelligent Automation
HSBC, one of the world's largest banking and financial services organizations, was facing a challenge with its customer service operations. With over 19,000 customer service agents, the bank was dealing with a high volume of repetitive tasks that put pressure on its agents. The traditional career path in the contact center world was also leading to inevitable attrition, as it was defined as agent → team manager → department manager → operations manager → head of contact centre. This lack of opportunity as the field narrows held back the chance of reaching the highest possible customer satisfaction with every interaction. Furthermore, HSBC was planning to shift towards Conversational Banking, which was expected to grow interactions considerably and require conversational experts to manage the chatbots.
Case Study
AB InBev: Optimizing Beer Manufacturing with Machine Learning
Anheuser-Busch InBev (AB InBev), a global corporation known for its popular beer brands like Budweiser, Corona, and Stella Artois, was facing challenges in optimizing its beer filtration process. The filtration process, which is crucial for achieving the best beer taste and meeting brand-required turbidity levels, involves many unpredictable variables. The existing technology could only handle basic logic, using meters to monitor and react to adverse conditions such as a change in pressure. AB InBev recognized the potential of machine learning (ML) and artificial intelligence (AI) in leveraging a larger dataset to better predict and prevent potential issues during filtration. However, the company needed a partnership and provider that could enable them to deploy ML quickly and effectively.
Case Study
Domino’s Enhances Customer Experience with Dialogflow’s Conversational Technology
Domino’s, a global pizza restaurant chain, has been at the forefront of digital innovation, offering customers the ability to order pizzas via 'Dom', an ordering bot available online and through the company’s mobile apps. However, with the changing consumer behavior and the need to innovate, the company faced the challenge of building rich conversational experiences powered by natural language understanding (NLU) and machine learning. The company needed a solution that could scale and accommodate the number of intents needed for a complex ordering process. The challenge was to account for the numerous directions a customer could take when ordering from their extensive menu.
Case Study
Dunzo's Transformation: Enhancing Customer Service with AI and Chatbots
Dunzo, an all-in-one 24x7 delivery platform, was facing challenges in maintaining its high standards of customer service as it scaled operations. The company, which fulfills over 2 million orders per month across India’s top 8 cities, was looking for a high-performing tool to handle high volume and support their load. The customer service team realized that 60% of the order-related queries they received were generic, and they wanted to reduce the number of chats that go to an agent. They needed a platform that could provide structured team performance reports to improve their customer experience. Dunzo also wanted to offer service through a familiar and convenient channel for its predominantly GenZ and Millennial customer base, for whom messaging is second nature.
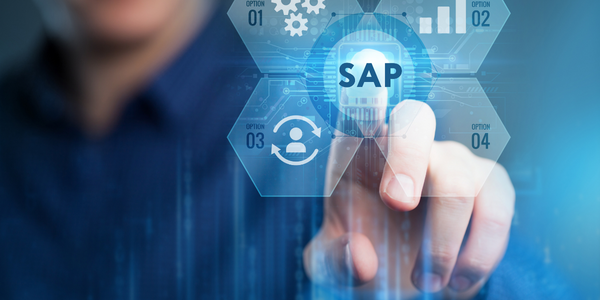
Case Study
SAP's Accelerated Deal Closure through Process Automation
SAP was grappling with the challenge of managing an increasing number of cloud deals. The rise in configurable cloud component deals necessitated a reduction in manual activity and a scaling up and automation of processes for order management, master data maintenance, and financial reporting. The high volume of cloud deals required a swift and efficient response, which was difficult to achieve with the existing manual processes. The challenge was to automate these processes to meet the growing demand and improve efficiency.
Case Study
Nordic Bank Nordea Employs Conversational AI to Scale Customer Service Across Four Markets
Nordea, a leading Nordic bank, was facing a significant challenge in managing the growing volume of customer service requests across its operations in Sweden, Denmark, Norway, and Finland. The bank, which serves over 9 million private customers and more than 500,000 active corporate customers, was receiving thousands of customer service requests daily through various channels including in-person, phone, email, and both live and automated chat. In 2017, Nordea’s Norwegian operation was receiving upwards of 2 million contacts per year across three contact center locations staffed by approximately 150 FTEs. The bank needed a scalable solution to manage this volume while providing consistent and on-brand experiences. They also identified a preference among their customer base to interact via chat, which had the advantage of handling multiple inquiries simultaneously.