公司规模
Mid-size Company
地区
- America
国家
- United States
产品
- Trimble eCognition Developer
- Trimble Inpho
技术栈
- Object-based Image Analysis
- LiDAR
- 3D Simulation
实施规模
- Enterprise-wide Deployment
影响指标
- Cost Savings
- Customer Satisfaction
- Productivity Improvements
技术
- 分析与建模 - 预测分析
- 分析与建模 - 实时分析
- 应用基础设施与中间件 - 数据交换与集成
适用行业
- 可再生能源
- 公用事业
适用功能
- 商业运营
- 销售与市场营销
用例
- 数字孪生
- 远程资产管理
服务
- 软件设计与工程服务
- 系统集成
关于客户
Geostellar is an online solar marketplace that aims to simplify the process of assessing and acquiring solar energy systems for residential properties. The company leverages geospatial technological advancements to provide a customized, real-time system that allows users to evaluate their property's solar potential, analyze financing options, and choose the most favorable solar option with just a few clicks. Geostellar's platform is designed to address the 'soft costs' associated with solar energy adoption, such as lead generation and customer acquisition, which can account for up to 50% of the total cost of solar installations. By automating the process of mapping building footprints and assessing solar potential, Geostellar aims to make solar energy more accessible and affordable for consumers across the United States. The company collaborates with various stakeholders, including local governments and utility companies, to provide comprehensive solar solutions that cater to the unique needs of different regions.
挑战
To achieve its mission to provide an on-demand, e-commerce system that could provide customized solar analytics, Geostellar first needed to have building vectors, the crucial data layer that would enable it to create precise maps and 3D models of all 3,143 counties in the US. Those foundational layers would then allow its system to map any rooftop’s solar potential in real-time. With millions of building footprints to map nationwide, a manual process of identifying and delineating buildings would not be feasible. Geostellar required an image analysis system that would almost completely automate the land classification process. And since each environment would present unique classification challenges, the solution needed the intelligence to quickly and accurately distinguish different structures from vegetative types and map out only buildings. In addition, although Geostellar would predominantly use LiDAR imagery for extracting buildings, not every county in the US has LiDAR data available. So the company also required a flexible system that could easily ingest and accurately classify imagery of variable quality.
解决方案
With geographic targets in mind, Geostellar began scouring available data repositories for high-resolution spatial imagery such as satellite data and LiDAR point clouds, together with ancillary information such as local tax rates, utility rates, precipitation and temperature, and zoning regulations. To address the issue of automatically creating building vectors, Geostellar chose Trimble eCognition object-based image analysis software. 'With eCognition, I can quickly assess the quality of the datasets that I’m working with, tweak the rule sets accordingly, and the software does the rest,' said Dan Koopman, a spatial analyst with Geostellar. 'And it’s fast–depending on county size, it can take one minute to three hours on average to produce a building layer. That’s about 90 minutes of manual time for every one minute of eCognition time, which is significant time savings.' Although the process changes with the geography, once the available data is integrated, eCognition typically analyzes the information to first separate vegetation from impermeable surfaces. Then, based on height, it determines which vegetation is grass and which are trees, and identifies rooftops and roads. It also delineates building footprints and maps them. Those vector maps are then used by Geostellar’s proprietary solar simulation engine to create and provide on-demand rooftop assessments. Needing only a user’s address and average cost of their monthly electricity bill, Geostellar’s geomatics platform runs a 3D simulation to compute how much sun hits their roof annually. It then automatically layers in other data such as local utility rates, property values, and incentives programs and calculates the property owner’s financial prospects for transitioning to solar. In addition to the real-time assessment, it also provides a list of financing options, and vetted manufacturers and installers for consideration. Users then simply click on the most favorable offering.
运营影响
数量效益
Case Study missing?
Start adding your own!
Register with your work email and create a new case study profile for your business.
相关案例.
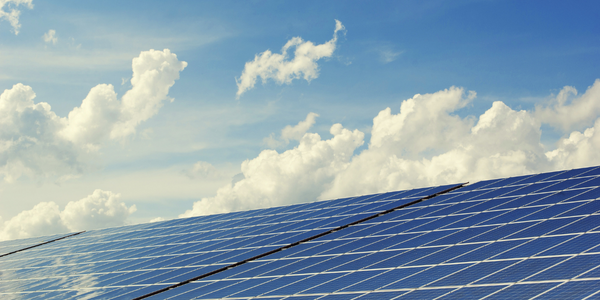
Case Study
Remote Monitoring & Predictive Maintenance App for a Solar Energy System
The maintenance & tracking of various modules was an overhead for the customer due to the huge labor costs involved. Being an advanced solar solutions provider, they wanted to ensure early detection of issues and provide the best-in-class customer experience. Hence they wanted to automate the whole process.
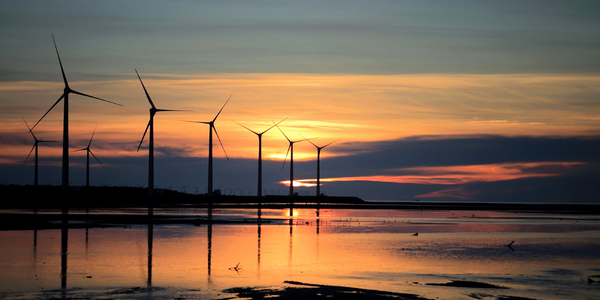
Case Study
Vestas: Turning Climate into Capital with Big Data
Making wind a reliable source of energy depends greatly on the placement of the wind turbines used to produce electricity. Turbulence is a significant factor as it strains turbine components, making them more likely to fail. Vestas wanted to pinpoint the optimal location for wind turbines to maximize power generation and reduce energy costs.

Case Study
IoT Solutions for Smart City | Internet of Things Case Study
There were several challenges faced: It is challenging to build an appliance that can withstand a wide range of voltage fluctuations from as low at 90v to as high as 320v. Since the device would be installed in remote locations, its resilience was of paramount importance. The device would have to deal with poor network coverage and have the ability to store and re-transmit data if networks were not available, which is often the case in rural India. The device could store up to 30 days of data.
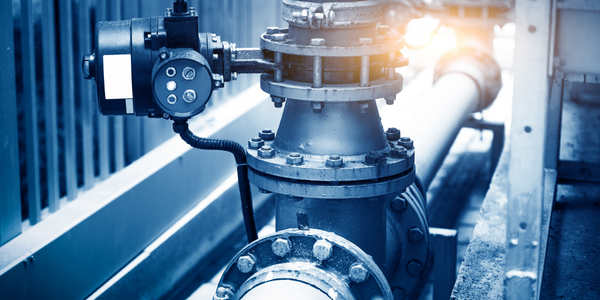
Case Study
Automation of the Oguz-Gabala-Baku water pipeline, Azerbaijan
The Oguz-Gabala-Baku water pipeline project dates back to plans from the 1970’s. Baku’s growth was historically driven by the booming oil industry and required the import of drinking water from outside of the city. Before the construction of the pipeline, some 60 percent of the city’s households received water for only a few hours daily. After completion of the project, 75 percent of the two million Baku residents are now served around the clock with potable water, based on World Health Organization (WHO) standards. The 262-kilometer pipeline requires no pumping station, but uses the altitude differences between the Caucasian mountains and the capital to supply 432,000 m³/d to the Ceyranbatan water reservoir. To the people of Baku, the pipeline is “the most important project not only in 2010, but of the last 20 years.”
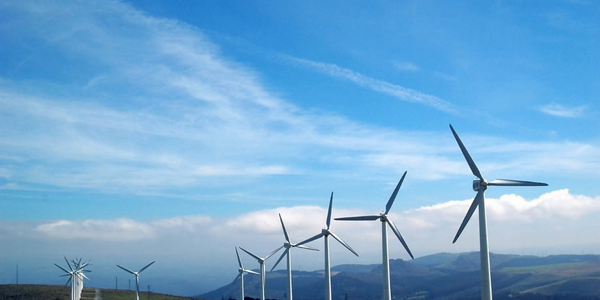
Case Study
Siemens Wind Power
Wind provides clean, renewable energy. The core concept is simple: wind turbines spin blades to generate power. However, today's systems are anything but simple. Modern wind turbines have blades that sweep a 120 meter circle, cost more than 1 million dollars and generate multiple megawatts of power. Each turbine may include up to 1,000 sensors and actuators – integrating strain gages, bearing monitors and power conditioning technology. The turbine can control blade speed and power generation by altering the blade pitch and power extraction. Controlling the turbine is a sophisticated job requiring many cooperating processors closing high-speed loops and implementing intelligent monitoring and optimization algorithms. But the real challenge is integrating these turbines so that they work together. A wind farm may include hundreds of turbines. They are often installed in difficult-to-access locations at sea. The farm must implement a fundamentally and truly distributed control system. Like all power systems, the goal of the farm is to match generation to load. A farm with hundreds of turbines must optimize that load by balancing the loading and generation across a wide geography. Wind, of course, is dynamic. Almost every picture of a wind farm shows a calm sea and a setting sun. But things get challenging when a storm goes through the wind farm. In a storm, the control system must decide how to take energy out of gusts to generate constant power. It must intelligently balance load across many turbines. And a critical consideration is the loading and potential damage to a half-billion-dollar installed asset. This is no environment for a slow or undependable control system. Reliability and performance are crucial.
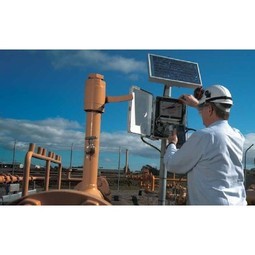
Case Study
GPRS Mobile Network for Smart Metering
Around the world, the electricity supply industry is turning to ‘smart’ meters to lower costs, reduce emissions and improve the management of customer supplies. Smart meters collect detailed consumption information and using this feedback consumers can better understand their energy usage which in turn enables them to modify their consumption to save money and help to cut carbon emissions. A smart meter can be defined in many ways, but generally includes an element of two-way communication between the household meter and the utility provider to efficiently collect detailed energy usage data. Some implementations include consumer feedback beyond the energy bill to include online web data, SMS text messages or an information display in consumers’ premises. Providing a cost-effective, reliable communications mechanism is one of the most challenging aspects of a smart meter implementation. In New Zealand, the utilities have embraced smart metering and designed cost effective ways for it to be implemented. The New Zealand government has encouraged such a move to smart metering by ensuring the energy legislation is consistent with the delivery of benefits to the consumer while allowing innovation in this area. On the ground, AMS is a leader in the deployment of smart metering and associated services. Several of New Zealand’s energy retailers were looking for smart metering services for their residential and small business customers which will eventually account for over 500,000 meters when the multi-year national deployment program is concluded. To respond to these requirements, AMS needed to put together a solution that included data communications between each meter and the central data collection point and the solution proposed by Vodafone satisfied that requirement.