How to build a data-enabled business model
Your company provides products or services. Maybe it has existed and thrived for decades, or maybe it's a new company trying to break into a competitive market. Regardless, data and digital technology will be a part of your company's future business model, or you will struggle to remain relevant. Companies are buildings competitive advantages into every aspect of their business model, from value proposition to pricing model to distribution channels, by leveraging digital technologies to be more agile, efficient, and differentiated.
Just like the consumer Internet, it is useful to think of the Internet of Things (IoT) as a system rather than a technology. The system is composed of both mature technologies like sensors and cutting-edge technologies like machine learning. Today, it is considered to be one of the ‘Big Four Tech Trends’ that are allowing companies all over the world to build more intelligent operations.
A typical IoT tech stack is typically made up of three layers. The application layer is where users access the system via websites, mobile apps, or wearable devices. The cloud layer aggregates data from operations and Internet systems and provides the computing infrastructure to store, process, and manage data. Finally, the edge layer is integrated into the physical world, where data is accessed and actions are automated.
Digitalization has already had a major impact on the global economy. Over the past decade, the list of the most valuable companies in the world has changed drastically. The top 10 companies traditionally consisted of oil companies and banks. However, tech companies almost completely occupy the top spots today. Apple built a consumer IoT and Paas (Platform as a Service) empire that is now valued at seven times more than former valuation leader Exxon Mobile.
Despite the increasingly obvious need to adapt to the new technology landscape, mature corporates are often slow to evolve their business models. There are a few key reasons for this:
- Large companies ignore opportunities that look small today but can become high-growth businesses tomorrow.
- Traditional accounting metrics usually pull resources towards growth in mature businesses.
- Emergent opportunities often have limited market data. Companies are risk-averse to investing until the opportunity matures, by which time there will be entrenched incumbents.
Despite the organizational challenges, building data-enabled revenue streams is more important than ever. Taking a look at the numbers, 70% of the executives that we spoke to for this report said that the Covid-19 outbreak is likely to accelerate the pace of their digital transformation. Companies that delay in building digital competencies risk finding themselves with commoditized offerings and inefficient operations as their competitors move forward.
In this article, we’ll discuss the various business model configurations that are applicable to mature companies by building data-enabled solutions on top of their legacy services, products and processes.
We’ll walk you through the process of defining the right IoT business model for your company and the benefits that you can gain from business model differentiation. We will also introduce the five principles of IoT business models, and provide an example of a formerly analog company that made the leap to a data-driven operation.
Finding the Right IoT Business Model for Your Company
When approaching IoT business models for the first time, it’s important to keep a few things in mind.
First, implementing and operating a data-enabled business model requires a mindset shift by the company. You are evolving how you create, capture and distribute value so it is important to understand that different skillsets and ways of working will be needed for the new business extension.
Of course, the existing business will not become obsolete. IoT solutions combine the applications, technologies, and requirements of operation technology (OT) and internet technology (IT). Possessing OT domain expertise is a strong competitive advantage when competing in IoT markets against startups or tech companies with an IT background.
Companies like Bosch, Caterpillar, and Omron have with strong operation technology competencies in domains like machinery, sensors, and M2M communications. This provides them with insight into how real-time operational data can be used to improve process control, asset monitoring, and other situations.
Internet technology companies tend to have stronger core competencies in programming languages, cloud services, and machine learning. They may be migrating to the IoT market from more traditional consumer or enterprise IT markets where customer needs are more standardized and data is easier to process.
Building an IoT business requires combining OT and IT technologies and mindsets.
Building an IoT business model requires the development of a complex technology stack and value chain. Suppliers and partners can fill technical capability gaps in an internal team. However, companies must develop a clear vision of their objectives. Four activities are useful for developing this vision:
- Extend the scope of vision to the ecosystem level by mapping relevant stakeholders, their contributions, and their own goals. Identify where goals overlap or conflict.
- Track and visualize value streams within the stakeholder network. Move beyond simply tracking linear value chains between suppliers and customers and consider the roles of tech partners, channel partners, consortiums, government entities, and other stakeholders.
- Focus on the value proposition for the customer rather than on the goals of the legacy business. It is very easy for the strategic decisions around a business extension to be held hostage by the priorities of the existing business. This is one reason that startups frequently outcompete mature corporates in new markets.
- Begin considering data as an asset within and beyond the direct opportunity. Explore how data can support improving existing services or create internal efficiencies.
Making the IoT Business Model Work For You
Whereas a traditional product mindset reacts to existing needs and lifestyles for customers, an IoT product mindset addresses real-time, emergent needs in a predictive manner. That means, that rather than a company chasing after a need or a trend, by implementing IoT, the company can stay ahead of the curve. By implementing an IoT business model, a company is able to become more agile in segmenting or evolving how it creates as it learns from the market. There are three basic IoT business model variants to consider:
| ![]() |
IoT business models are highly differentiated, but all involve one or more iterations of these three models. Each of these three variants can be sold using any of 18 sales models archetypes.
The beauty of building solutions around data is that you have granular, objective data into what works and what does not work. Data-enabled solutions are also inherently more flexible than traditional product sales since it is easier to package the offering in creative ways.
Most companies seek to monetize their new IoT solutions. However, the 'low hanging fruit' that justifies the initial business case is often related to internal value creation. It is always worth exploring how better visibility into how a product is purchased or used can support the internal sales, service, and R&D teams.
By focusing on the problems and needs of customers, companies differentiate solutions, access new channels and build new growth pillars to boost revenues. Meanwhile, by leveraging IoT solutions for internal operations, they can improve efficiency, reduce cycle times, and optimize their cost structure.
The essence at the heart of both monetization and internal value creation is building a direct line of visibility into customers' needs and behaviors.
The Five Principles of IoT Business Models
The five principles of IoT business models are an important navigation tool when establishing your IoT business model. They are:
- Align the business model to overall company strategy: To ensure strategic fit, you should validate at an early stage whether and how the opportunity contributes to the overall company strategy. This can be done in the idea selection phase, using checklists that represent key values or strategic goals of the company.
- Identify key drivers of value: Adopt a user-centered approach when defining the IoT offering, starting by defining the value proposition and assessing which parts of the solution are central to the value proposition.
- Assign tasks to internal and external stakeholders according to their capabilities: To minimize costs and efforts and maximize value, use a resource-based view to allocate resources. Determine what capabilities are required for the IoT offering and which stakeholders can best provide them. Do not aim to build the entire stack if partners are available.
- Future-proof business models at an early stage: Consider how to ensure the sustained economic viability of the business model as early as possible. Aspects might include data rights, technological evolution, human resources, or network dynamics. Expect both the solution and the external dynamics to evolve constantly.
- Document and validate assumptions that have an impact on the business model: Throughout the business model development process, many assumptions are made about target groups, market size, user acceptance, technical feasibility, costs, etc. It is crucial to document them and to explore how much they affect the business model.
Regardless of the objective or solution, implementation will follow a multi-step process that generally requires a focused yet iterative approach. The four basic steps towards defining a data-driven business model include:
- Ideation - start broad and maintain an open mind.
- Qualification - refine the scope of an idea before investing in it.
- Validation - validate that the concept shows promise through 'lean startup' tactics before investing heavily in R&D.
- Design - run an iterative process to move from prototype to MVP to beta in order to ensure that you are building the right solution.
- Market Entry - it generally makes sense to enter a niche or secondary market first in order to receive feedback and refine the solution before entering the mass or primary market.
Throughout these processes, a variety of stakeholders will be involved but a core team should typically own the process from end-to-end. This is the model that startups follow and that venture investors prefer. Handoffs in responsibility tend to dilute the vision or leave to confusion. The ideation phase takes between one and three weeks and involves figuring out the problem in question and the data required to solve it.
A data-enabled business model can differ from a traditional business model in four dimensions. Some companies radically rethink the entire business. However, modifying one dimension can already provide significant differentiation in the market.
- Product - The most obvious change is adding new functionality to the product. This could come in the form of smart sensors, automation of the physical device, or complementary software built on top of the existing asset base.
- Revenue Model - Data-enabled businesses have more flexibility in revenue models since data about system performance or usage is available. This data opens up possibilities for usage-based or benefit-based pricing models that are not feasible with a 'dumb' system.
- Distribution Channels - The path to market for a digitalized solution can often differ. For example, SaaS is more suitable for e-commerce than traditional enterprise software. Ecosystem sales are also more viable since the data acquired by use of a system is often valuable to a range of other businesses. This provides those businesses with an incentive to integrate the business with their offerings.
- Prospecting Strategy - Many producers of industrial products have very limited visibility into the questions "Who is using our product? How are they using it? And where?". Insight derived from product usage data can enable more precise segmentation and improve R&D and sales effectiveness.
The Schindler Case Study
For almost 15 years, Swiss multinational company Schindler has been building IoT solutions into its business. Schindler has launched a powerful digital platform that monitors elevators and escalators. This platform provides access to data that enables new product and service offerings for customers, as well as internal efficiency gains.
With a B2B model that generates, organizes, integrates, analyzes, visualizes, and makes decisions using data, Schindler can use AI to monitor machinery in real-time via sensors, identify and forecast maintenance issues, recommend measures needed, and send a technician to implement these measures.
Schindler’s implementation of IoT has made their product safer for users, while allowing them to build new forms of technology for use by external customers, including its myPORT service, which gives building users higher security and seamless personalized transport between buildings.
Data-enabled business models allow a company to increase the value generated from products and service for customers, internal users, and partners. The low marginal cost of digital solutions also allows higher margins after R&D costs are accounted for. With real-time data collection and large storage bases, companies have the power to ask themselves, “Where is the value left on the table?” and quickly answer the question with solutions that will benefit multiple layers of their businesses.
POV: Asia Digital Landscape - Business Model Innovation in Asia
Multinational companies tend to centralize their core research and product development capabilities in their home regions. For American and European companies, this often means that their businesses in Asia are large in terms of the number of employees and revenue generated, but weak in terms of local technical capability.
Due to this situation, business model innovation makes sense as a strategic priority in Asia. It is easier to implement business model localization or to add software-enabled functionality than it is to localize the core product. It is also often more useful to localize digital solutions. Consider the case of Schindler. Elevators function the same globally in terms of engineering dynamics. There is little need for Schindler to localize elevator R&D, and they may prefer to keep their core know-how centralized to reduce intellectual property risk. However, adjacent services such as in-elevator marketing, regulatory compliance, servicing, and building management must often be localized to meet unique needs in each market. Their R&D efforts in Asia can thus focus on developing digital products and services that meet needs in local markets.
Related Insights.
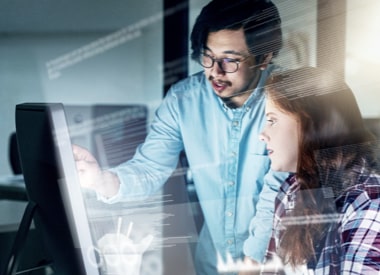
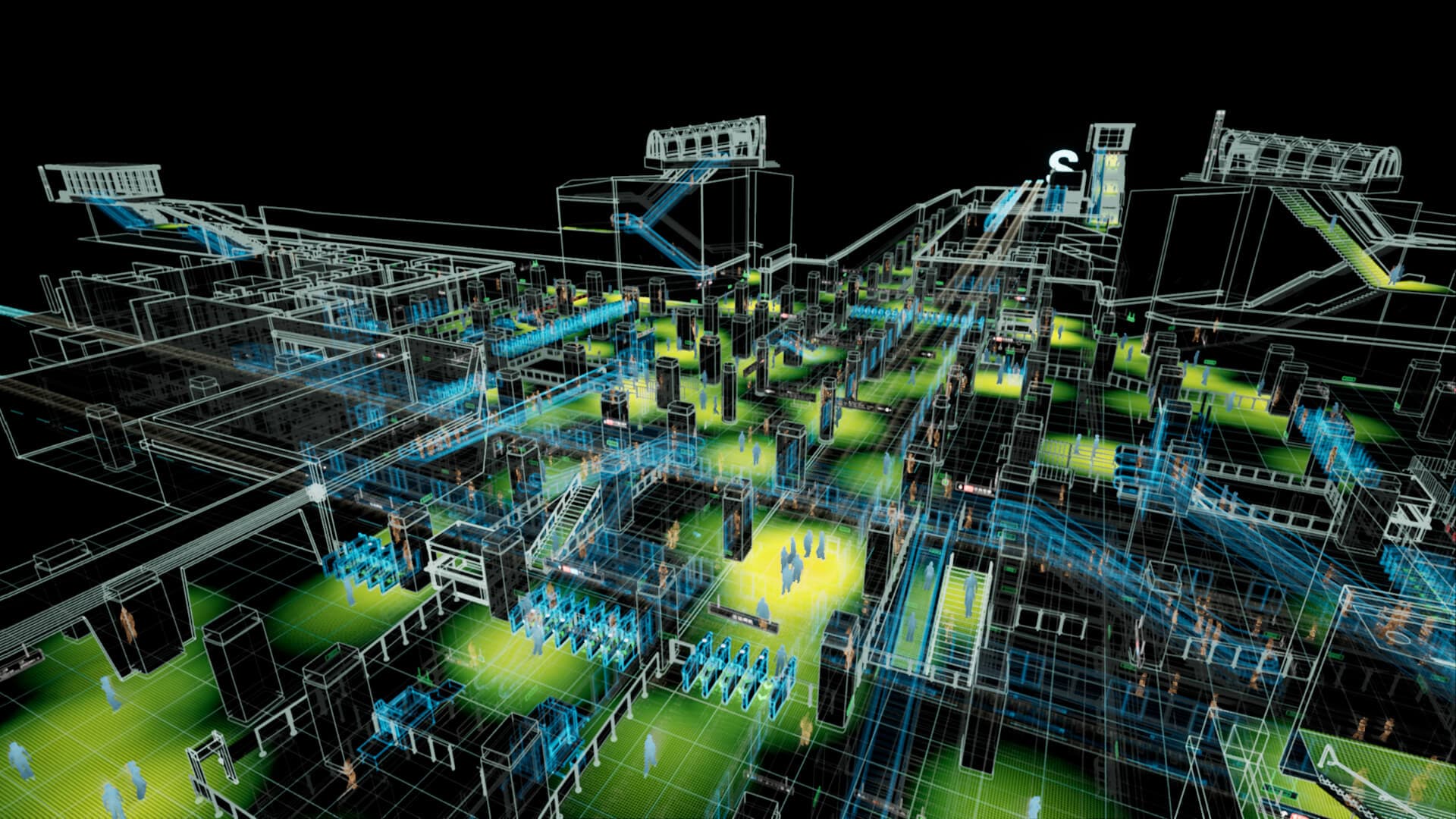
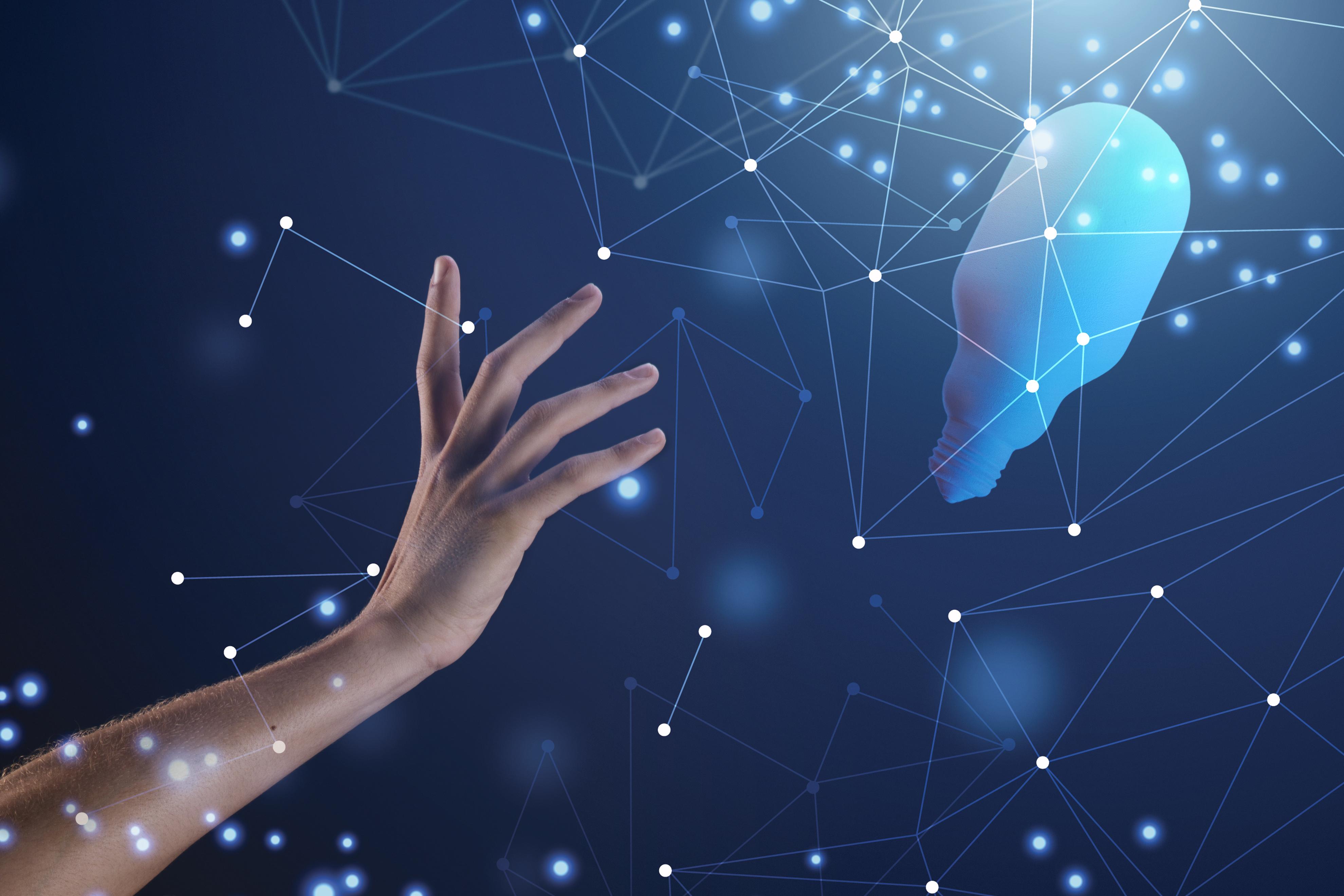
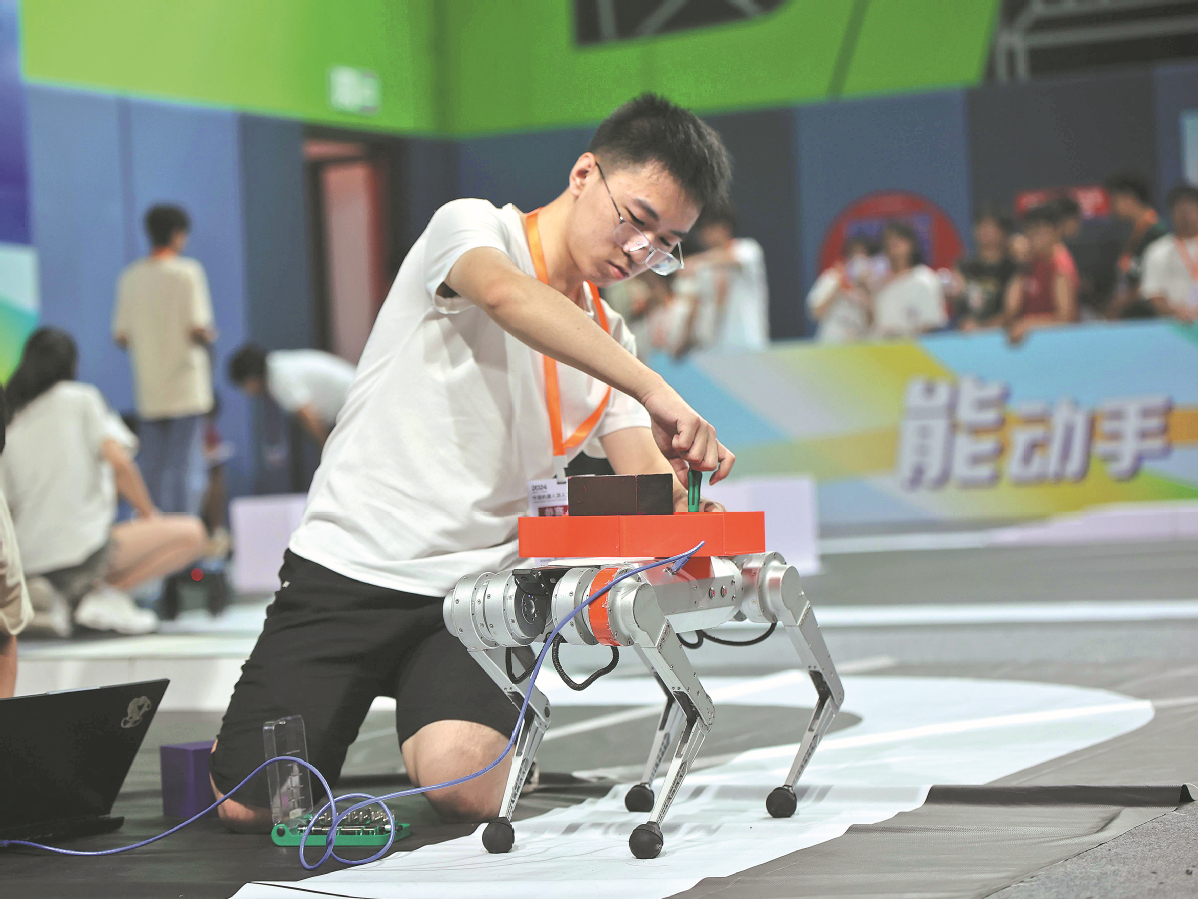
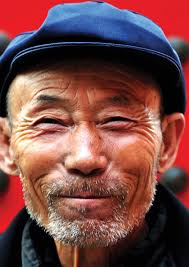
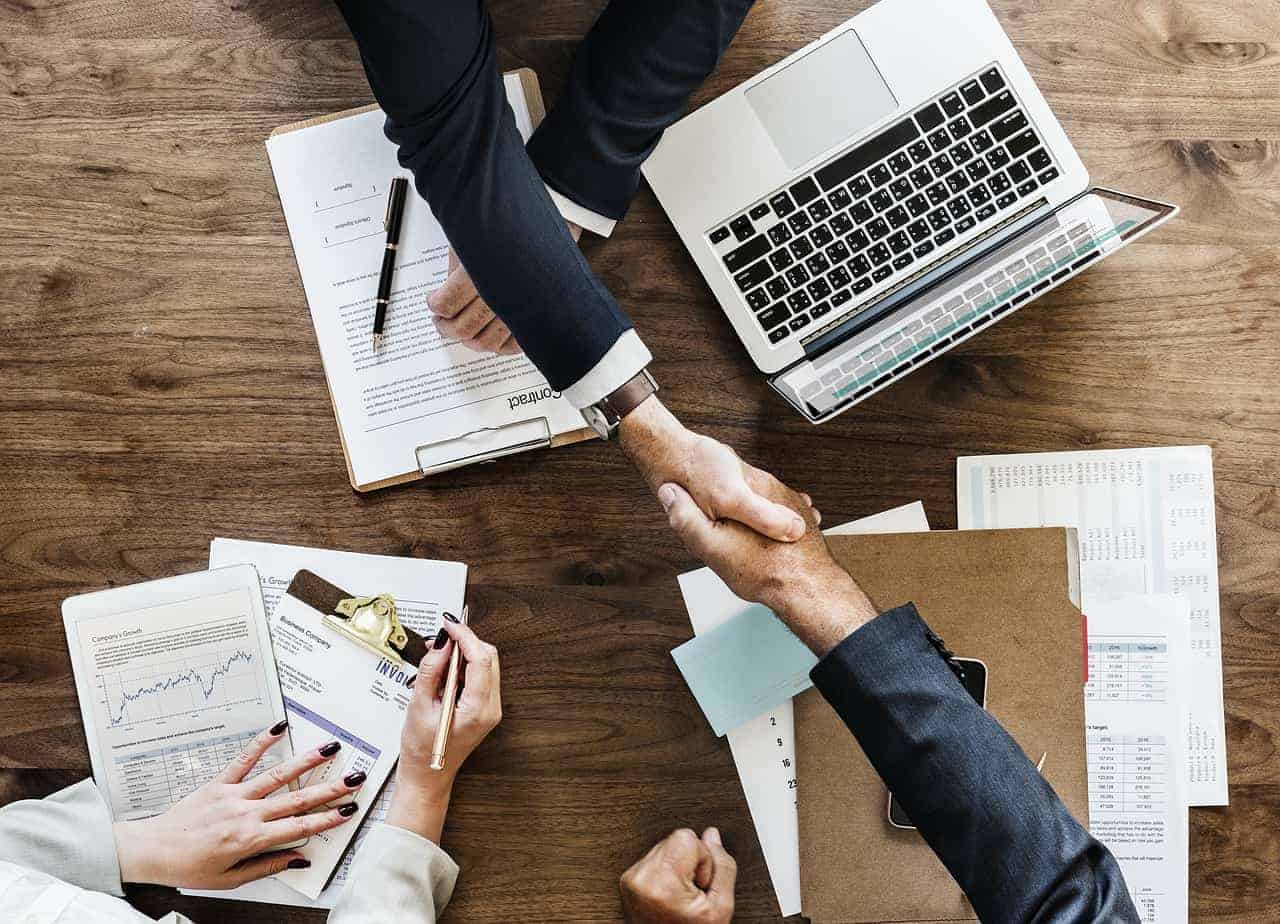