Aravo Solutions
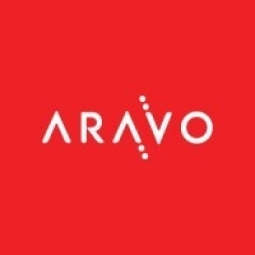
概述
公司介绍
Aravo delivers the market’s smartest third-party risk and resilience solutions, powered by intelligent automation. For more than 20 years now, Aravo’s combination of award-winning technology and unrivaled domain expertise has helped the world’s most respected brands accelerate and optimize their third-party management programs, delivering better business outcomes faster and ensuring the agility to adapt as programs evolve.
Supplier missing?
Start adding your own!
Register with your work email and create a new supplier profile for your business.
实例探究.
Case Study
InMobi's Transition to Databricks Lakehouse: A Case Study on Streamlining Data Processing and Enhancing Advertising Effectiveness
InMobi, a company specializing in targeted mobile advertising, was grappling with the challenges of managing a complex legacy infrastructure and a multicloud data warehouse. The company's data processing requirements had escalated to 20+ terabytes per hour, leading to skyrocketing costs and the creation of data silos that hindered collaboration and data sharing. The proprietary nature of their multicloud data warehouse also posed significant challenges. InMobi's existing system was overly complex, prone to outages, and extremely costly to scale. The company realized that their current system was slowing down their ability to innovate and was keeping their engineering resources tied up in maintenance tasks. InMobi sought a single system that could address multiple issues, consolidate their disjointed systems into a single platform, and free up their engineers to focus on higher-value tasks such as developing machine learning and large language models.
Case Study
Democratizing Data for Supply Chain Optimization at Johnson & Johnson
Johnson & Johnson, a global consumer goods and pharmaceutical provider, faced significant challenges in managing its supply chain data. The company's growth through acquisitions led to a fragmented data system with disparate priorities and unique configurations. Data was largely being extracted and analyzed manually, limiting opportunities for speed and scalability. The disconnection was negatively impacting customer service and impeding strategic decision-making. The company also faced the challenge of optimizing inventory management and costs on a global scale, which required accurate and abundant data. The inability to understand and control spend and pricing could lead to limited identification of future strategic decisions and initiatives, potentially missing the opportunity to achieve $6MM in upside.
Case Study
Grammarly Enhances Communication with Databricks Lakehouse Platform
Grammarly, a company that provides AI-powered communication assistance, was facing challenges with its legacy, homegrown analytics system. As the company grew, it became increasingly difficult to evaluate large data sets quickly and cost-effectively. The existing system was time-intensive to learn, making it challenging to onboard new hires. It also failed to meet the needs of essential business functions, particularly marketing, sales, and customer success. Analysts often had to resort to copying and pasting data from spreadsheets as the system couldn't effectively ingest the external data needed to answer critical business questions. Reporting was also a challenge as the system didn't support Tableau dashboards. Furthermore, Grammarly sought to unify its data warehouses to scale and improve data storage and query capabilities. The existing setup, with large Amazon EMR clusters running 24/7, was driving up costs. Data silos emerged as different business areas implemented analytics tools individually, and a single streaming workflow made collaboration among teams challenging.
Case Study
Grip's Smart Shipping Solutions through Databricks Lakehouse
Grip, a company that serves e-commerce businesses shipping perishable goods across the U.S., processes hundreds of thousands of orders through its platform each month. The company's challenge was to process and interpret a variety of data points to make the most effective shipping recommendations. The data came from multiple sources including Shopify, ShipStation, different warehouse management systems, APIs for weather data, carrier pricing and delivery time tracking, and customer support systems like Zendesk and Dynamics. The company needed to consolidate this data to suggest the best carrier, ideal refrigerant and insulation, packaging and material, and other shipping logistics. Additionally, once a delivery was made, Grip needed to publish analytics so customers could see orders that went out, where they went, areas of the country that are bottlenecked, and areas of the country that are performing well.
Case Study
Fastned's Sustainable Transportation Revolution Powered by Databricks Lakehouse
Fastned, a pioneer in the fast-charging infrastructure for electric vehicles (EVs), faced a significant challenge as the EV industry grew exponentially. The company started with a small footprint of charging stations and expanded as the industry took root. However, as the size of Fastned’s data grew exponentially, the company needed to migrate away from its legacy system, Redshift, which was resource intensive to scale and expensive for democratizing insights across its teams. Fastned was also faced with the pressures of building more charging stations and ensuring a continued superior user experience, which became increasingly challenging to deliver with its existing tech stack. The company's data team quickly realized that with an increase in data collection points across its network, the legacy AWS Redshift data warehouse would not be able to meet its growing needs. And while Tableau was being leveraged to deliver insights, wide-scale analytics was hindered by high costs.
Case Study
GreenFlex Leverages IoT Data for Energy Efficiency with Databricks Lakehouse Platform
GreenFlex, a European leader in environmental management services, energy efficiency, and environmental impact management, was facing a significant challenge in managing and governing the vast amount of data it was collecting. The company gathers energy consumption data from its customers and uses machine learning to identify consumption anomalies and devise energy strategies. However, as the volume of data grew exponentially, GreenFlex needed a simplified way to manage and govern this data. The company needed to make the data easily and securely accessible for data exploration, business intelligence, and machine learning use cases. Additionally, GreenFlex was maintaining three unconnected workspaces for development, staging, and production workloads, leading to complications in dealing with security and access controls over the tables in each workspace. This design also led to issues with data availability across these workspaces.
Case Study
Honeywell's Data Management Transformation with Delta Live Tables
Honeywell, a global provider of industry-specific solutions, is under increasing pressure to reduce energy use, lower costs, and improve efficiency. Their Energy and Environmental Solutions division uses IoT sensors and other technologies to help businesses manage energy demand, reduce energy consumption and carbon emissions, optimize indoor air quality, and improve occupant well-being. This requires Honeywell to collect vast amounts of data from millions of buildings worldwide. These buildings are equipped with thousands of sensors monitoring factors such as temperature, pressure, humidity, and air quality. In addition to this, data is also collected from external sources like weather and pollution data, and information about the buildings themselves. At peak times, Honeywell ingests between 200 to 1,000 events per second for any building, equating to billions of data points per day. Honeywell's existing data infrastructure was struggling to meet this demand, making it difficult for the data team to query and visualize the disparate data to provide customers with fast, high-quality information and analysis.
Case Study
Optimizing Customer Engagement with Databricks Lakehouse: A Case Study on Iterable
Iterable, a company that helps brands optimize and humanize their marketing, was facing challenges with its data infrastructure. The company needed to build personalized and automated customer experiences for its clients, which required harnessing diverse, complex data sets and facilitating rapid prototyping of machine learning models. However, the infrastructure they initially built with AWS native tools, including EMR, was resource-intensive, costly to maintain, and created significant operational overhead. This made it difficult for Iterable to scale the level of data ingestion and rapid prototyping of machine learning models needed to support its customer requirements and respond quickly to changes in the market. Furthermore, the company's AI solutions had to account for diverse data variables, drifts in the model, new regulatory changes, and a growing demand for more privacy protection.
Case Study
Transforming Viewer Experience with IoT: A Case Study of ITV
Over the past decade, the broadcast television industry has undergone significant changes, largely due to the rise of streaming services. These changes have led to shifting viewer expectations, with people now expecting to be able to access a wide range of high-quality programming at any time and on any device. ITV, a British public broadcast television network, faced the challenge of meeting these changing expectations while also managing vast amounts of content data generated by nearly 40 million viewers. The company formerly relied on multiple legacy data platforms, which resulted in data fragmentation. Its data team was similarly fragmented across marketing, commercial advertising and product experience with its own technology stack. When the company launched its new digital strategy, it became clear it would need to modernize its platform and undergo a massive digital transformation with data at the core. ITV sought a platform that would allow it to consolidate its data sources and use analytics, machine learning, rule-based algorithms, and other tools to understand viewer expectations and behavior and improve the user experience.
Case Study
Transforming Customer Support with IoT: A Freshworks Case Study
Freshworks, a provider of CRM and customer experience solutions, was facing challenges in improving the performance of its customer support organization due to a legacy Hadoop infrastructure and an assortment of data tools. With over 60,000 enterprise customers and multiple product lines, the company was struggling to maintain exceptional customer satisfaction due to the high volume and level of support required. The manual approach to managing help desk tickets was not sufficient to keep up with the demand. The company's internal enterprise data platform, powered by Hadoop, was composed of multiple data and analytics tools, which incurred massive IT overhead to manage upgrades and monitor performance. This environment created performance bottlenecks as data volumes increased, slowing down the customer support team’s ability to efficiently service customers.
Case Study
Revolutionizing Data Accessibility and Analysis at The Hershey Company
The Hershey Company, a renowned name in the retail and consumer goods industry, was facing challenges in data management and analysis. Despite reaching a revenue milestone of $10 billion in 2022, the company was struggling with disconnected data sources, which prevented a single, consistent view of their data. This was a significant obstacle in making fast, data-driven decisions and staying ahead of market changes. The company aimed to build a Commercial Data Store (CDS) to serve as a single source of truth for commercial data across the entire organization. However, the complexity of Hershey’s data environment was further compounded by the fact that the company maintained separate data platforms to handle data for major retail customers.
Case Study
Accelerating Autonomous Vehicle Development with IoT
Incite, a company that provides the Rapid OEM Automotive Data (ROAD) platform to the world's largest automakers, was facing significant challenges in processing the massive amounts of unstructured data generated by test fleets. This data is crucial for building safer autonomous vehicles. However, frequent performance issues made it difficult for Incite to deliver fleet behavior metrics that clients could easily consume. Additionally, customers had to spend hours manually searching individual files for the data they needed, which significantly slowed down product development. The process of ingesting terabytes of data and making it visible in end-user dashboards took two to three weeks, which was a major bottleneck in the development process.
Case Study
Revamping Data Management for Enhanced Patient Care: A Case Study on Integra Life Sciences
Integra Life Sciences, a global provider of medical technologies, faced a significant challenge when the COVID-19 pandemic disrupted the medical supply chain. The company needed a comprehensive view of global supply and demand to ensure the availability of its products for elective surgeries. However, its aging data warehouse limited its supply chain agility, causing delays in accessing critical data on usage patterns, stock levels, and quality issues. The legacy warehouse system, IBM DataStage, was time-consuming and inflexible, hindering the company's ability to respond to changing needs swiftly. The company needed a solution that would provide timely insights into inventory and demand, enabling it to deliver its products more efficiently.
Case Study
Transforming Urban Mobility with IoT: A Case Study on Intelematics
Intelematics, an Australian real-time traffic information provider, was grappling with the challenge of synthesizing massive amounts of data from various traffic and mobile sources. The company's ability to provide insights from historical and real-time traffic trends relied on converting 12 billion rows of traffic data into actionable insights. However, the native systems previously used were tedious and laborious, hampering speed, efficiency, and collaboration across different teams. The company recognized the need to move away from its legacy on-premises infrastructure to keep up with increasing customer requests. The challenge was to process 12 billion road traffic data points every 30 seconds, sourced from millions of sensors and IoT devices on commercial and private vehicles. The native systems impeded the speed and efficiency of the data pipelines used to process bulk data in real time, such as traffic patterns, vehicle movements, and extensive monitoring data.
Case Study
Kaltura's Transformation: Powering Limitless Video Experiences with Databricks and dbt
Kaltura, a company providing live, real-time and on-demand video SaaS solutions, faced the challenge of building a near real-time event pipeline. The data team was tasked with creating a new data product based on streaming events sent from users’ devices. This pipeline would need to capture events and write them directly into a data lake, detecting anomalies and notifying stakeholders of spikes in the number of events. The data engineering team, which had recently transitioned from supporting primarily the company’s cloud TV unit to serving the entire company, was also tasked with replacing the legacy infrastructure with a new data lake platform.
Case Study
Aravo Solutions Powers Fidelity International’s Global Third-Party Risk and Performance Program
Fidelity International (FIL), a global investment and retirement savings business, was in need of a technology partner to manage their global third-party supplier risk and performance management program. The challenge was to maintain a single inventory of all their third-party risk information, identify and segment critical and high-risk suppliers, and streamline the end-to-end third-party risk and performance management processes across the enterprise. The solution needed to provide advanced reporting capabilities and dashboard visualizations for a complete view of risk and performance, ensuring greater governance and standing up to regulatory scrutiny and good business practice. Furthermore, the solution had to be flexible, cost-effective, and able to scale and adapt to change quickly to support Fidelity International’s long-term requirements for success.
Case Study
Fueling Growth with Predictive Models and Improved Customer Experience: A Case Study on Explorium
Explorium, a company that integrates organizations' data with the world's most reliable sources for predictive modeling and informed business decisions, was facing a challenge. The company was seeking to minimize data latency and free its data engineers from the task of building ELT pipelines. Explorium's platform determines the characteristics of the data and identifies potential enrichments it can make. However, the company was struggling with loading the right data quickly, regardless of the technical challenges it faced on the back end. The company was using Amazon EMR to run its ELT pipelines but realized its data engineers were spending too much time building these pipelines. This was slowing down the release of new data products and the onboarding of new data sets to its platform.
Case Study
Enabling Financial Inclusion with Faster Loans through IoT
Finda, a data-driven lending platform in Korea, was facing challenges in managing its data environment due to spikes in data volumes and an increase in data users. The company's complex data environment was made up of different analysis systems used for various analysis demands, making it difficult to extract data insights and value for its customers. Frequent application outages due to scalability issues limited its ability to respond to sudden increases in users or operational activity. The company also struggled with data engineering activities such as table creation, modification, and deletion in the service database, which was used for back-end services. This absorbed valuable resources and impacted SLAs. The core issue was Finda’s legacy data warehouse, which was inefficient in managing storage and resulted in runaway operating costs. The system also required constant maintenance to synchronize the data catalog on both storage environments.