Databricks
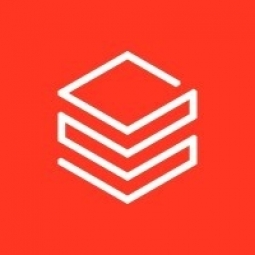
概述
总部
美国
|
成立年份
2013
|
公司类型
私营公司
|
收入
$1-10b
|
员工人数
1,001 - 10,000
|
网站
|
推特句柄
|
公司介绍
Databricks is a data and AI company. More than 10,000 organizations worldwide — including Comcast, Condé Nast, Grammarly, and over 50% of the Fortune 500 — rely on the Databricks Data Intelligence Platform to unify and democratize data, analytics and AI.
Supplier missing?
Start adding your own!
Register with your work email and create a new supplier profile for your business.
实例探究.
Case Study
Amgen's Digital Transformation: Accelerating Drug Development with IoT
Amgen, the world’s largest independent biotech company, embarked on a digital transformation journey to leverage its data for better outcomes across the business. The company faced challenges in improving R&D productivity, optimizing supply chains, and commercialization. The problems that the data teams were looking to solve had drastically changed over the years and were no longer isolated by skillset, department, or function. The most impactful problems were cross-functional and required a collaborative approach. Amgen had a wealth of valuable data within its core verticals - clinical trials, manufacturing, and commercialization. However, increasing volumes of data presented challenges when it came to using that data efficiently. The company was unable to weave together the various aspects of its business, which impacted operational efficiency as it scaled both internally and with its customer base. The key challenge was to make it easy to access and process data in a collaborative manner that ties in different personas with different viewpoints on the data.
Case Study
Transforming the Australian Rail Industry’s Digital Ecosystem with IoT
The Australia Rail Track Corporation (ARTC) is a crucial part of Australia’s supply chain, managing and monitoring goods transportation across 8,500km of rail network. The corporation collects vast amounts of data to ensure the timely delivery of cargo freight. However, ARTC was dealing with the complexity of siloed data across several segregated data sources, which posed inefficiencies and unnecessary complexity. This also impacted the cost of managing a network that is segregated across remote locations in Australia. The data sets were required by more than 150 applications generated from different data sources, meaning any change to the data needed to be altered in several systems. ARTC embarked on a business transformation strategy to digitally modernize and manage physical rail assets more efficiently, while building resilience to readily respond to customer demands, regulatory compliance, and varied world events.
Case Study
Burberry's Bold Fashion Marketing Transformation with IoT
Burberry, a British luxury brand, faced a significant challenge in managing and annotating its thousands of marketing images. The company needed to classify these assets accurately to use them effectively in its marketing campaigns and drive the right action by the right audience. Burberry initially tried using an open-source tool for image annotation, but it had serious drawbacks. The company was looking for a solution that could improve the data for training its models quickly and easily. They wanted to produce labels for thousands of images and place them seamlessly into a model development pipeline for convenient reuse. The challenge was to find a solution that would integrate well with Burberry's existing Databricks implementation, a single, unified analytics platform for all its stakeholders.
Case Study
Creating a Winning Recipe for a Hyperefficient Supply Chain: A Case Study on Barilla
Barilla, the world's largest pasta producer, faced significant challenges due to unpredictable crop yields and rising raw material costs caused by climate change. These disruptions led to product shortages and quality issues, necessitating a more sustainable and resilient supply chain strategy. However, Barilla's legacy on-premises data warehouse was unable to scale their operations effectively, creating silos that hindered their ability to navigate these external threats. The company's supply chain and logistics needed to maintain high-quality standards while maximizing revenue in a low-margin business. As Barilla's distribution expanded to meet growing global demand, it became clear that they needed to modernize their data to enable analytics at scale. With over 1TB of data ingested daily, data analysts struggled to integrate and analyze the necessary data, leading to delays and inefficiencies. Additionally, Barilla's large European footprint required processing sensitive consumer data for GDPR compliance, necessitating a robust data governance plan.
Case Study
Cleanaway's Transformation: Leveraging IoT for a Smarter and Cleaner Australia
Cleanaway, Australia’s leading waste and recycling services provider, faced a significant challenge in managing data from its diverse operations. The company's operations, including waste collection, sorting, and logistics, involved different IT systems, leading to data being scattered across multiple, disjointed sources. This data sprawl was a major obstacle as the complexity and variability of Cleanaway’s services generated large volumes of operational and service data from various sources, including GPS and connected fleet systems, and structured and unstructured data from customer transactions, sales, marketing, and more. The company's ambition to become an efficient and profitable data-first business as part of its Blueprint 2030 objectives was hampered by these data silos, which impeded the sharing of actionable insights and resulted in unreliable insights due to inconsistent data quality.
Case Study
Carvana Enhances Customer Experience with Databricks DLT and Streaming
Carvana, an online used car retailer, developed its Next Generation Communication Platform (NGCP) to provide a seamless car shopping experience. However, the NGCP team faced several challenges related to scale, data quality, and high data warehouse costs. The team initially streamed its conversation and AI data into Google BigQuery, which limited how data engineers could partition and optimize query tables. Data quality was another challenge, with engineers needing to dedupe in the pipeline, but distinct calls on large data frames were slow and caused recomputation on the entire data set. The team also faced data availability challenges, with no process to automatically pick up experiment data as campaigns were configured and run. Maintenance and transparency were another challenge, as a single repo contained both the ETL and business logic. Finally, the data sets produced often contained too many files to be shipped to data warehouses via the Spark Connector, creating a data export bottleneck.
Case Study
Leading Latin America Into an Open Finance Future with IoT
Banco Bradesco, a leading financial institution, was facing challenges in meeting evolving customer expectations due to its legacy data warehouse. The existing system was too cumbersome to share customer data with other entities, with customer, transactional, and consent data scattered across disjointed systems. This lack of collaboration hindered proper data analysis and AI, creating obstacles to fully embrace open finance and the move to become a cloud-first bank. The bank's legacy on-premises infrastructure needed to improve to overcome the technical barriers of sharing 20+ terabytes of customer financial data with outside organizations. Rolling out new applications with over 1,000 siloed databases would take months, making deployment inefficient. Engineers struggled to complete projects, as they were inundated with IT operations work to maintain their infrastructure. Analysts needed help accessing and exploring data promptly, dependent on an overburdened engineering team to provide curated data and reports.
Case Study
Revolutionizing Gaming Experience with IoT: A Case Study on Devsisters' Cookie Run
Devsisters, a global entertainment and gaming app developer, launched Cookie Run in 2013, which quickly became a success with 2.9 million daily active users in Korea and over 10 million downloads within 12 weeks of its launch. However, as the user base grew, Devsisters' on-premises platform began to experience performance issues and became complex to manage and costly to maintain. The company needed a new data analytics platform that data platform engineers and data scientists could use to analyze big data more quickly and seamlessly. The existing infrastructure was associated with high maintenance costs and frequent issues that required support from software engineers, leading to a diversion of resources from enhancements to troubleshooting. Devsisters attempted to solve this by building its own SQL-based data querying environment based on Spark Thrift Server, but the challenges persisted, including non-functional update and delete queries and slow performance.
Case Study
Real-Time Analytics at Scale: Akamai's Transformation with Delta Lake
Akamai, a global content delivery network (CDN) provider, manages approximately 30% of the internet’s traffic through its 345,000 servers spread across more than 135 countries. In 2018, Akamai launched a web security analytics tool to provide its customers with a unified interface for assessing a wide range of streaming security events and perform real-time analysis. This tool ingests approximately 10GB of data related to security events per second, with data volumes increasing significantly during peak retail periods. The tool initially relied on an on-premises architecture running Apache Spark™ on Hadoop. However, Akamai faced challenges in meeting its strict service level agreements (SLAs) of 5 to 7 minutes from when an attack occurs until it is displayed in the tool. The company sought to improve ingestion and query speed to meet these SLAs and provide real-time data to its customers.
Case Study
AT&T's Cloud Migration for Enhanced Customer Service and Operational Efficiency
AT&T, a leading telecommunications company, was grappling with the challenges of managing a massive on-premises legacy Hadoop system. The system was complex and costly, hindering operational agility, efficiency, and the optimal use of engineering resources. AT&T needed to transition to the cloud to better support its hundreds of millions of subscribers. The company was dealing with a highly complex hardware setup, including 12,500 data sources and over 1,500 servers. The on-premises Hadoop architecture was not only expensive to manage but also complex to maintain. Operational costs were high, and additional capital costs were incurred for data centers, licensing, and more. Up to 70% of the on-prem platform had to be prioritized to ensure 50K data pipeline jobs met SLAs and data quality objectives. Engineers were spending more time managing updates, fixing performance issues, and provisioning resources rather than focusing on higher-value tasks. The physical infrastructure's resource constraints also slowed down data science activities, impeding innovation.
Case Study
Block's Transformation of Financial Services with Databricks
Block, a global technology company, was facing challenges in managing a large volume of data crucial for graph-related use cases. This included handling graph databases, leveraging various machine learning tools, and optimizing performance for petabytes of data. Operational inefficiencies and scalability concerns arose due to the fragmented nature of data across diverse business units. The cumbersome data transfers between these systems, combined with the siloed nature of data governance policies, posed auditing and policy enforcement challenges. Block was also in need of a proper implementation and uniformity of data governance policies to ensure compliance with privacy laws like GDPR and CCPA for both customers and internal teams.
Case Study
Lakehouse Empowers CareSource to Streamline Data for Enhanced Healthcare Delivery
CareSource, a nationally recognized healthcare organization, has been experiencing exponential growth over the past 30 years. This growth has led to an influx of new members, which the company's legacy data systems were unable to handle efficiently. The company had to resort to temporary solutions such as running jobs designed for monthly execution on a daily basis, which the systems were not designed to handle. This resulted in the expenditure of significant resources to maintain the systems. The company needed a modern data platform that could scale, perform efficiently, and be future-proof. The platform needed to be cloud-based and capable of serving as a single source of truth for all incoming data.
Case Study
Large Scale ETL and Lakehouse Implementation at Asurion
Asurion's Enterprise Data Service team was tasked with gathering over 3,500 data assets from the entire organization, providing a unified platform where all the data could be cleaned, joined, analyzed, enriched, and leveraged to create data products. The previous iterations of data platforms, built mostly on traditional databases and data warehouse solutions, encountered challenges with scaling and cost due to the lack of compute and storage separation. With increasing data volumes, a wide variety of data types, demand for lower latency and increased velocity, the platform engineering team began to consider moving the whole ecosystem to Apache Spark™ and Delta Lake using a lakehouse architecture as the new foundation. The previous platform was based on Lambda architecture, which introduced problems such as data duplication and synchronization, logic duplication, different ways to deal with late data, data reprocessing difficulty due to the lack of transactional layer, and platform maintenance downtimes.
Case Study
Barracuda Networks' Use of Machine Learning on Databricks Lakehouse for Phishing Attack Prevention
Barracuda Networks, a global leader in security, application delivery, and data protection solutions, was faced with the challenge of handling sophisticated phishing emails. The company had built a powerful artificial intelligence engine that uses behavioral analysis to detect attacks and keep malicious actors at bay. However, the sophistication of attackers in creating malicious emails posed a significant challenge. The company needed to assess and identify malicious messages to protect their customers. Additionally, Barracuda Networks offered impersonation protection, a service that prevents malicious actors from disguising their messages as coming from an official source. However, these targeted phishing attacks required the attacker to have personal details about the recipient, making them harder to detect and block. Furthermore, Barracuda faced difficulties with feature engineering. They needed to utilize the right data and do feature engineering on top of that data, which included email text and statistical data. Before the Databricks integration, building features was more difficult with the labeled data spread over multiple months, particularly with the statistical features. Also, keeping track of the features when the data set grew in size was challenging.
Case Study
Leveraging Data Insights for Personalized Customer Experience: A Case Study on ButcherBox
ButcherBox, a young e-commerce company, operates on a monthly subscription-based model, delivering fresh, organic, and ethically sourced meat and seafood to its customers. However, the company faced significant challenges due to the complexity of its operations and the vast amount of data it had to manage. The data, coming from various sources such as email systems and the company's website, was siloed, preventing complete visibility into critical insights needed for strategic and marketing decisions. The data team struggled to deliver timely and accurate reports and insights. The legacy data warehouse environment was proving to be a hindrance to the company's agility and speed, making it difficult to keep up with the changing needs of their growing customer base, improve supply chain operations, and forecast demand.
Case Study
Revolutionizing Autism Diagnosis in Children through IoT and Machine Learning
The process of diagnosing autism in children has been historically plagued by subjectivity and inconsistency. The existing assessments are lengthy, require in-clinic visits, and there is a growing shortage of specialists. Furthermore, disparities in diagnosis based on socioeconomic status, race, gender, and geography have been observed. The average age of diagnosis in the U.S. is 4.3 years, and parents and caregivers often spend up to 3 years seeking answers. Early diagnosis is crucial as it can greatly alter the course of development and may even erase the signs of autism altogether.
Case Study
Transforming Health Insurance: Collective Health's Data Integration with Delta Live Tables and Structured Streaming
Collective Health, a technology company revolutionizing health insurance, faced a significant challenge in managing and integrating data from various partners. The company's mission to simplify employer-led healthcare and improve health outcomes required a robust, flexible infrastructure that could handle vast amounts of data. However, the company's existing data integration architecture was not equipped to handle the evolving business requirements. The schema was constantly changing, and columns that previously contained data started to contain null values. Moreover, the company needed a solution that could ingest files incrementally without having to go through each file previously ingested. The challenge was to find a solution that could handle these complexities while ensuring data quality and scalability.
Case Study
Leveraging IoT and Machine Learning to Enhance Customer Experience: A Case Study on AIR MILES Reward Program
Since 1992, LoyaltyOne has operated the AIR MILES Reward Program, generating $70 billion in revenue across their network. Despite having billions of data records from collectors and retail partners, AIR MILES struggled to foster stronger touch points with its customers due to a lagging legacy infrastructure. This outdated system prevented the company from gaining a holistic view of their customers, thereby hindering their ability to improve retention and customer lifetime value. AIR MILES wanted to leverage the petabytes of customer data generated by 558 million transactions per year to enhance the collector experience and enable their partners to better engage and drive revenue. However, their existing technology was unable to support this vision. The company's data scientists couldn't access data older than 5 years, and complicated queries limited what they could do with the data they did have access to. Pipeline development alone took 3 to 4 months, stifling their ability to deliver innovative solutions to market in a timely manner.
Case Study
Boosting Customer Conversion with Conversational AI: A Case Study on Aktify
Aktify, a company specializing in conversational AI, aims to help its clients convert their customers through AI-driven SMS conversations. The company's goal is to make these AI agents as effective as possible, which requires drilling into massive volumes of data to find overlooked insights. However, Aktify faced challenges in managing its complex data dependencies, which hindered data democratization. The company needed a way to allow different teams to interact with data as per their needs. For instance, while a data scientist might need raw data, an executive team would prefer pre-aggregated data for quick decision-making. The company also sought to minimize the risk associated with data transformations and to make data management more simple, flexible, and cost-effective.
Case Study
Creating a Unified Customer View Across 200+ Brands: Al-Futtaim's Journey
Al-Futtaim, a major economic driver in the Middle East, operates and distributes a wide range of global brands, generating a wealth of data across these brands. However, their legacy systems struggled to provide a unified view of their customer due to data silos and the inability to scale efficiently to meet their analytical needs. Business intelligence was slow, forecasting was not scalable and was limited to classical algorithms, and customer insights were too generalized to affect outcomes faster. Their data teams were unable to maximize the promise of their data due to memory and CPU constraints, outdated libraries, and inflexible tooling. Data analysts had to wait four to six hours for small amounts of data to process, which slowed both time-to-insights as well as time-to-market of new ideas. The extra time, effort, and resources required to redesign, implement, test, and launch data projects added unnecessary costs, created a greater divide between business units, and ultimately slowed innovation.
Case Study
AT&T's Transformation: From Legacy Infrastructure to Cloud-Based Lakehouse for Enhanced Fraud Detection
AT&T, a leading communication service provider, was facing challenges with its legacy on-premises architecture. Despite having 182 million wireless customers and handling 10 million transactions per second, the company was struggling to stay ahead of fraudsters. The existing infrastructure was complex and failed to deliver the innovation required for an optimal customer experience. The company was using rule-based technology for fraud detection, which was reactive rather than proactive, making it difficult to stay ahead of sophisticated fraud attempts. The process was not only protracted, inefficient, and resource-heavy, but also expensive. AT&T also struggled to gain real-time insights and automation necessary to optimize dispatch. The company could not unify data points to match a technician’s troubleshooting skills to the customer issue and location, leading to increased operational costs and a negative impact on customer experience.
Case Study
Global Media Giant Condé Nast Enhances Data Architecture for Scalability and Efficiency
Condé Nast, a global media company that cultivates 37 of the world’s most influential and iconic brands, was planning its global expansion. However, the company realized that its data architecture was too complex to provide the scalability it needed. The company had stored its data in siloed systems, with five different data sources integrated with its then query engine, Presto. Data engineers ran ETL jobs and processes on Databricks Lakehouse and stored the data in Amazon S3. They also created tables in Databricks and pointed them to the storage layer in AWS S3. The data warehousing team used Informatica to build data models, stored the results in S3, and worked with data engineers to point that data set back toward Presto so that teams could access it in data queries. This complex and siloed data architecture was hindering the company's growth and expansion plans.
Case Study
Personalizing Talent Acquisition with IoT: A Case Study of 104 Corporation
104 Corporation, Taiwan’s leading online recruitment solutions provider, was facing challenges due to their legacy cloud tooling and the need for a modern data infrastructure. Despite having access to terabytes of highly valuable, private data, including personal information, engagement details, and transaction information, the company struggled to scale pipeline costs effectively and spent significant resources on maintenance operations. This was slowing their ability to be truly data-driven. Their existing systems were siloed, preventing data teams from gaining relevant, timely insights for informed decision-making. The company was hampered by specialty ETL tooling like EMR and a legacy data warehouse in SAP Sybase that were disparate and complex to scale. As the company continued to expand, the increasing quantity of data became significantly more challenging to manage and utilize.
Case Study
Empowering Marketers with Next-Gen Customer Intelligence: A Case Study on Acxiom
Acxiom, a customer intelligence company, was facing the challenge of managing and analyzing the increasing volume of data captured by their customers. As digital experiences evolved across various channels and platforms, reaching consumers and meeting their expectations became a moving target. The company helps brands understand people through data, enabling them to create ethical, data-driven solutions that drive business growth through better customer experiences. However, the proper collection and usage of data was becoming increasingly complex. The company realized they needed to migrate to the cloud to scale and support hundreds of billions of transactions a month, while maintaining data privacy and security. The challenge was to continue capitalizing on their long heritage of identity data management in a safe, scalable, and cost-effective manner.
Case Study
Arkadium's Transformation: From Traditional Gaming to Data-Driven Success
Arkadium, a leading creator of online games for adults, faced significant challenges in storing and processing large amounts of unstructured and semi-structured data. The company's traditional SQL-based on-premises data warehouses were unable to keep up with the volume of data being processed, which was close to 500GB in just one pipeline. This data was crucial for analyzing user behavior and driving customer monetization, engagement, and retention. Additionally, the company's hyper-growth led to a data swamp with inconsistent data, as each team was independently storing its data in various locations. This was a major challenge for Arkadium, as they needed to unify all data for effective analysis and decision-making.