Snorkel AI
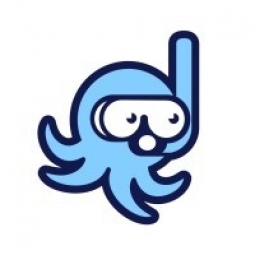
概述
总部
美国
|
成立年份
2019
|
公司类型
私营公司
|
收入
$10-100m
|
员工人数
51 - 200
|
网站
|
推特句柄
|
公司介绍
Snorkel AI makes AI development fast and practical by transforming manual AI development processes into programmatic solutions. Snorkel AI enables enterprises to develop AI that works for their unique workloads using their proprietary data and knowledge 10-100x faster.
Supplier missing?
Start adding your own!
Register with your work email and create a new supplier profile for your business.
实例探究.
Case Study
Automating KYC Verification with AI: A Case Study of a Global Custodial Bank
A global custodial bank was facing a significant challenge in its Know Your Customer (KYC) process. Analysts and investment managers were spending over 10,000 hours annually reviewing and transcribing 10-Ks, which are critical for verifying a company’s identity, establishing a risk profile, and informing multiple business processes. The bank was processing over 10,000 documents each year, with each document taking 30-90 minutes to review. The process was further complicated by the fact that 10-Ks come in various formats, and if any information was missing or incorrect, analysts had to spend additional time hunting it down. This not only lengthened the customer onboarding process but also gave competitors an opportunity to swoop in. The bank had tried to solve the problem using a rule-based system, but it proved to be rigid and could only identify a narrow scope of information for certain document formats/layouts. The system also required frequent updates due to constant changes in regulations across several regions, which took months to implement.
Case Study
Big Four Consulting Firm Leverages NLP for Efficient Auditing with Snorkel Flow
A globally renowned consulting firm, with a history spanning over a century, was seeking to enhance its auditing capabilities by leveraging artificial intelligence. The firm's reputation hinged on its ability to conduct thorough audits, irrespective of their size, complexity, or location. The firm's experts were spending significant time manually reviewing various accounting, auditing, and industry information, a process that was both time-consuming and costly. The firm estimated that each auditor search lasted 10 minutes and cost $50-60 on average. The firm's data science team was tasked with streamlining news monitoring to anticipate changes in capital markets, regulatory trends, or technological innovation. They aimed to use custom NLP models to automatically analyze, categorize, and extract key client information from various sources. However, they faced challenges in labeling training data for the machine learning algorithms. It took three experts a week to label 500 training data points, and they found it nearly impossible to adapt to changes in data or business goals on the fly.
Case Study
Georgetown University’s CSET Leverages Snorkel Flow for NLP Applications in Policy Research
The Center for Security and Emerging Technology (CSET) at Georgetown University was faced with the challenge of building NLP applications to classify complex research documents. The goal was to surface scientific articles of analytic interest to inform data-driven policy recommendations. However, the team found that a large-scale manual labeling effort would be impractical. They initially experimented with the Snorkel Research Project, which allowed them to programmatically label 90K data points within weeks, achieving 77% precision. However, the collaboration between data scientists and subject-matter experts was time-consuming and inefficient, involving spreadsheets, Slack channels, and Python scripts. This workflow made improving data and model quality a slow process. The team was constrained by inefficient tooling to auto-label, gain visibility into data, and improve training data and model quality. The lack of an integrated feedback loop from model training and analysis to labeling also meant that data scientists and subject matter experts had to spend long cycles re-labeling data to match evolving business criteria. These challenges limited the team’s capacity to deliver production-grade models, shorten project timelines, and take on more projects.
Case Study
Scaling Clinical Trial Screening at MSKCC with Snorkel Flow
Memorial Sloan Kettering Cancer Center (MSKCC), the world’s oldest and largest cancer center, was faced with the challenge of identifying patients as candidates for clinical trial studies by classifying the presence of a relevant protein, HER-2. The process of reviewing patient records for HER-2 was laborious and time-consuming as it required clinicians and researchers to sift through complex, variable patient data. The data science team at MSKCC wanted to use AI/ML to classify patient records based on the presence of HER-2, but the lack of labeled training data was a significant bottleneck. Labeling data, especially complex patient records, required clinician and researcher expertise and was prohibitively slow and expensive. Even when experts were able to manually annotate training data, their labels were at times inconsistent, limiting model performance potential.
Case Study
Enhancing Proactive Well Management: Schlumberger's Use of Snorkel Flow
Schlumberger, a leading provider of technology and services for the energy industry, faced a significant challenge in extracting crucial information from a vast array of daily reports. These reports, ranging from daily drilling reports to well maintenance logs, each had their unique structure and format, making it difficult for Schlumberger’s team to quickly extract the necessary information. The team attempted to automate the information extraction using Named Entity Recognition (NER), but off-the-shelf ML models failed to identify the scientific terms related to the Exploration and Production (E&P) industry. Creating a domain-specific training dataset was time-consuming and not scalable, taking anywhere from 1-3 hours per document. The team needed to identify 18 different industry-specific entities and automatically associate data with these entities. However, the rich information was buried within tabular and raw text in PDFs with varied formatting across reports from different companies. There was also poor collaboration between domain experts and data scientists, with cumbersome file sharing and ad-hoc meetings.
Case Study
Accelerating NLP Application Development with Foundation Models: A Pixability Case Study
Pixability, a data and technology company, provides advertisers with the ability to accurately target content and audiences on YouTube. However, with over 700 million hours of YouTube content being watched daily, Pixability faced the challenge of continuously and accurately categorizing billions of videos to ensure ads run on brand-suitable content. Their existing natural language processing (NLP) model for classifying videos was not performing strongly enough. The process of labeling training data for the machine learning solution was slow due to reliance on external data labeling services that required multiple iterations. Collaboration was constrained due to limited time domain experts and data scientists had to solve for ambiguous labels. Additionally, valuable information within titles, descriptions, content, and tags was difficult to normalize.