Dataiku
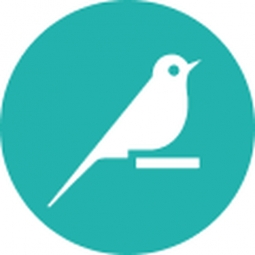
概述
公司介绍
Dataiku 是一个集中式数据平台,可将企业的数据之旅从大规模分析转移到企业 AI。通过为数据专家和探索者提供共同基础、最佳实践库、机器学习和人工智能部署/管理的捷径以及集中、受控的环境,Dataiku 是数据驱动型公司的催化剂。联合利华、通用电气和 FOX 新闻集团等客户使用 Dataiku 来确保他们快速行动并随着他们收集的数据量成倍增长。通过消除障碍,Dataiku 确保为影响业务的模型和创造性解决方案提供更多机会,使团队能够更快、更智能地工作。
主要客户
联合利华、通用电气、福克斯
物联网应用简介
技术栈
Dataiku的技术栈描绘了Dataiku在平台即服务 (paas), 应用基础设施与中间件, 和 分析与建模等物联网技术方面的实践。
-
设备层
-
边缘层
-
云层
-
应用层
-
配套技术
技术能力:
无
弱
中等
强
Supplier missing?
Start adding your own!
Register with your work email and create a new supplier profile for your business.
实例探究.
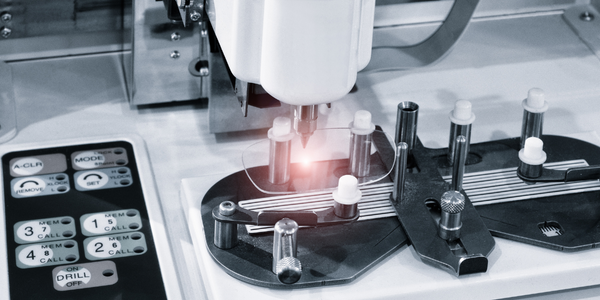
Case Study
Improving Manufacturing Processes with Essilor
Seeing that one of their goals is to find ways to better answer consumer and business needs, the Global Engineering (GE) team was facing the challenge of improving processes and performance of the surfacing machines to significantly improve their production by using the increasing volume of data."We wanted a data science platform that would allow us to solve our business use cases very quickly. Thanks to Dataiku and its collaborative platform, which is agile and flexible, data science has become the norm and is now used more widely within our organization and around the world," said Cédric Sileo, Data Science Leader at Global Engineering, Essilor.
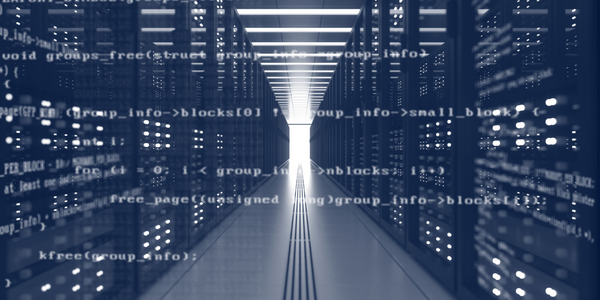
Case Study
U.S. Venture Leverages Dataiku to Streamline Data Efforts and Save Thousands of Hours
U.S. Venture, a company operating in diverse industries such as automotive aftermarket, energy, and technology, faced significant challenges in managing and analyzing customer data due to its complexity. The company struggled with creating enterprise tools and processes that could eliminate silos and promote collaboration. The Data and Analytics team at U.S. Venture, established in 2018, initially focused on data warehousing and basic reporting. However, they soon realized the need for advanced analytics at scale. The team faced difficulties in maintaining models and disparate data sources, which could quickly become unmanageable without the right people and tools. Additionally, the team's data scientists and analysts were using a varied set of tools and coding mechanisms, leading to a lack of standardization and collaboration. The individual team members built their own components that lived in different places and were created via their own tools, saved on personal computers, with no visibility for other team members about where projects were and how they were created or functioned.
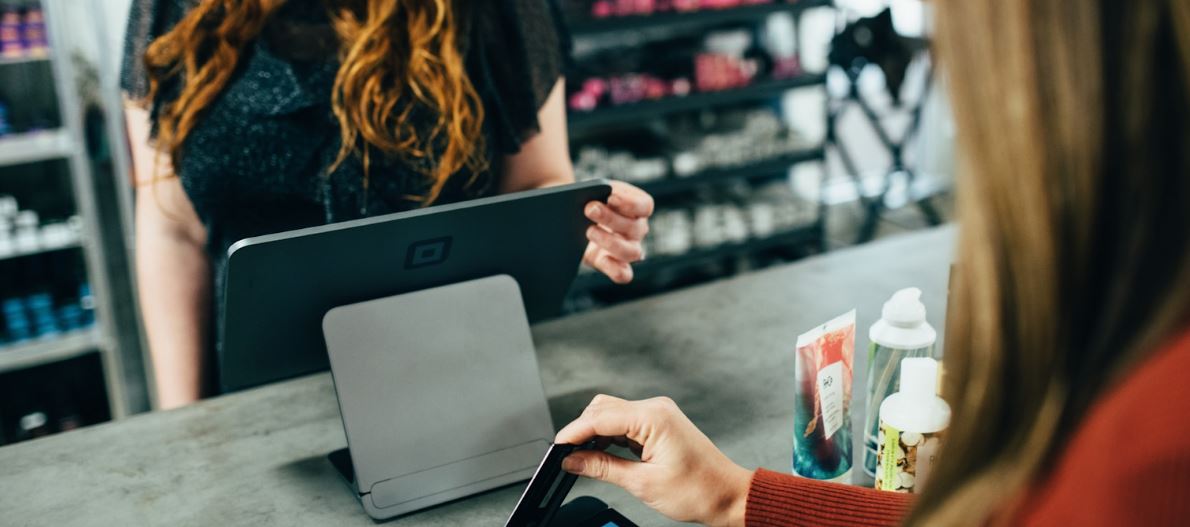
Case Study
Revolutionizing Dynamic Pricing with Pricemoov and Dataiku
Pricemoov, a yield management solution provider, faced a significant challenge in handling and cleaning data from old SI systems, Oracle, or MySql. The data was dirty and required a full-time developer to perform long ETL (extract-transform-load) steps in PHP for cleaning. Once cleaned, the datasets were painstakingly entered into a model, as they were custom-built pipelines. The replication and deployment process for the next customer was taking weeks. This slow and inefficient process was hindering Pricemoov's ability to provide optimal pricing suggestions and solutions to its customers in a timely manner.
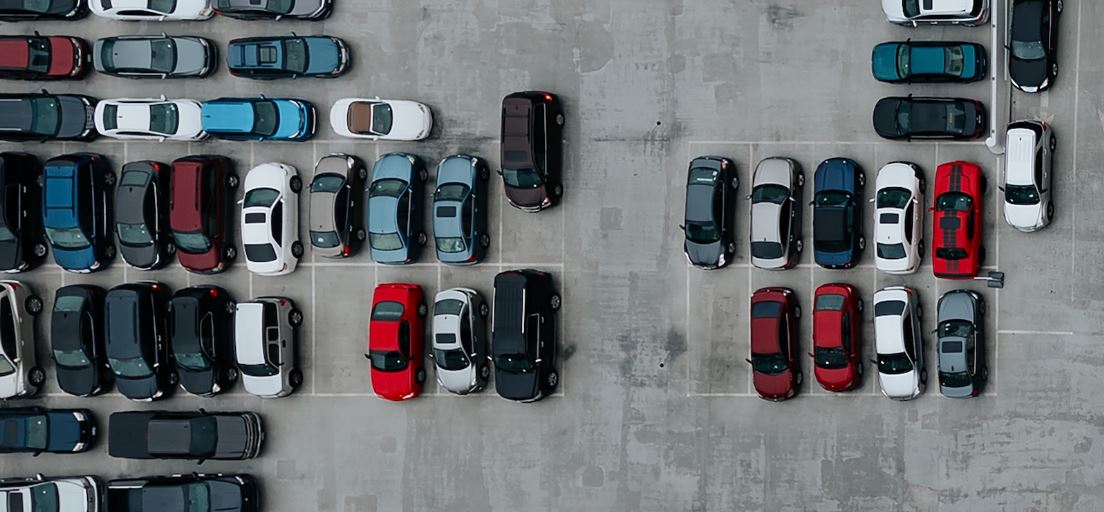
Case Study
Revolutionizing Car Rental Industry: Europcar Mobility Group's Data-Driven Approach
Europcar Mobility Group, a global mobility service provider operating in 130 countries, was facing challenges in accurately predicting fluctuations in demand for car rentals at airports based on market changes. The International Air Transport Association predicted an increase of 2.35 billion annual passengers by 2037, particularly in the Asia-Pacific region, which would significantly impact Europcar's operations. To address this, Europcar aimed to build an application using data from various sources, including fleet traffic, passenger volume, reservation and billing data, and data on new airline routes. However, the data was scattered across different locations, in different formats, and was massive in volume, posing a significant challenge.
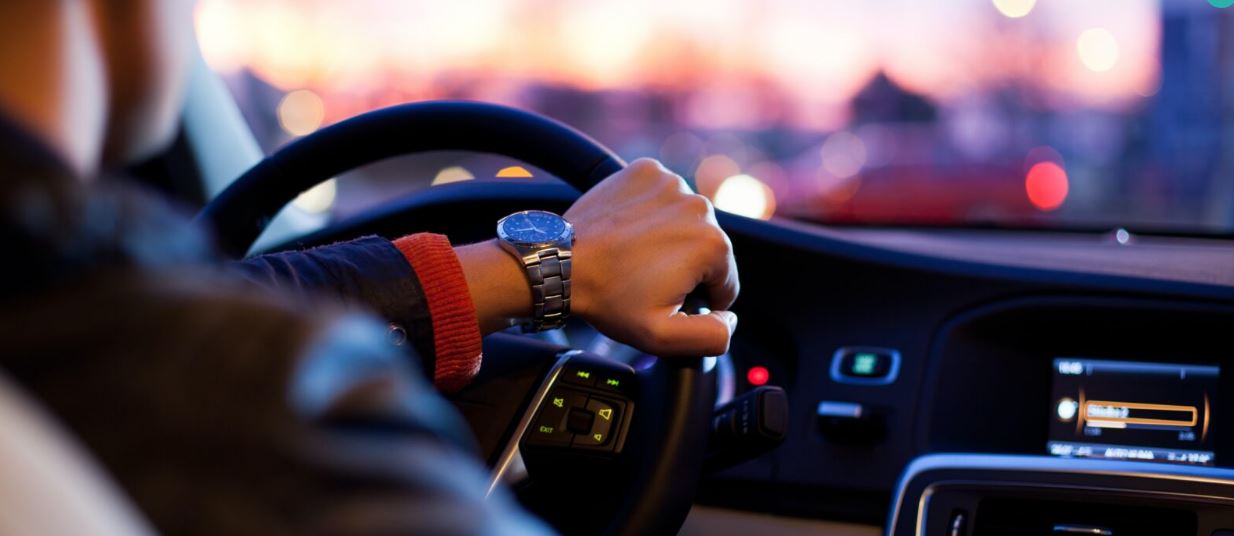
Case Study
Heetch's Elastic AI Strategy Development with Dataiku
Heetch, a French mobility company, was struggling with the management of large quantities of data gathered from drivers, passengers, and global operations. As the company grew, the costs of their data warehouse were spiraling out of control and performance was suffering due to the increasing volume of data. They needed a solution that would allow anyone in the organization to work with large amounts of data while also ensuring optimized resource allocation. The challenge was to find a way to leverage big data with good performance and at reasonable costs, which required serious computational power, optimized resource consumption, and isolated environments for development and production. Managing all these aspects was becoming increasingly complex for the organization.
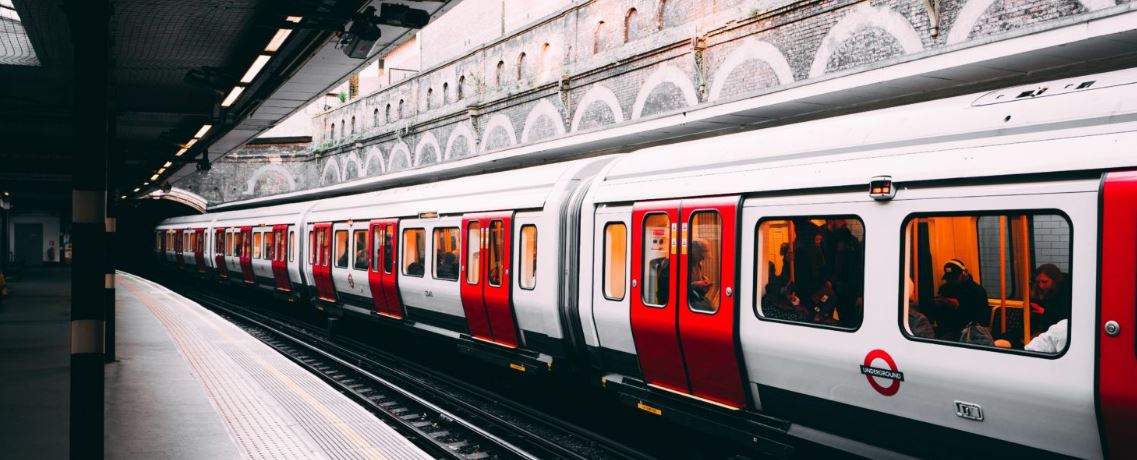
Case Study
Trainline's Global View of Marketing Acquisition through IoT
Trainline, Europe’s leading independent train travel platform, faced a significant challenge in monitoring and improving their marketing acquisition. With paid campaigns running 24/7 and users interacting with those ads around the clock, static dashboards were no longer sufficient. The company needed a dynamic, real-time data solution to provide the most accurate marketing insights. They had a technical team within the marketing department tasked with creating aggregated, centralized dashboards focused on Trainline marketing acquisition efforts. However, this ambitious endeavor required data science skills and a tool robust enough to blend and support multiple data formats and sources to track acquisition according to certain parameters. The challenge was to find a tool that would allow the technical team to improve and upgrade their skills while also satisfying the marketing department’s requests quickly and efficiently.
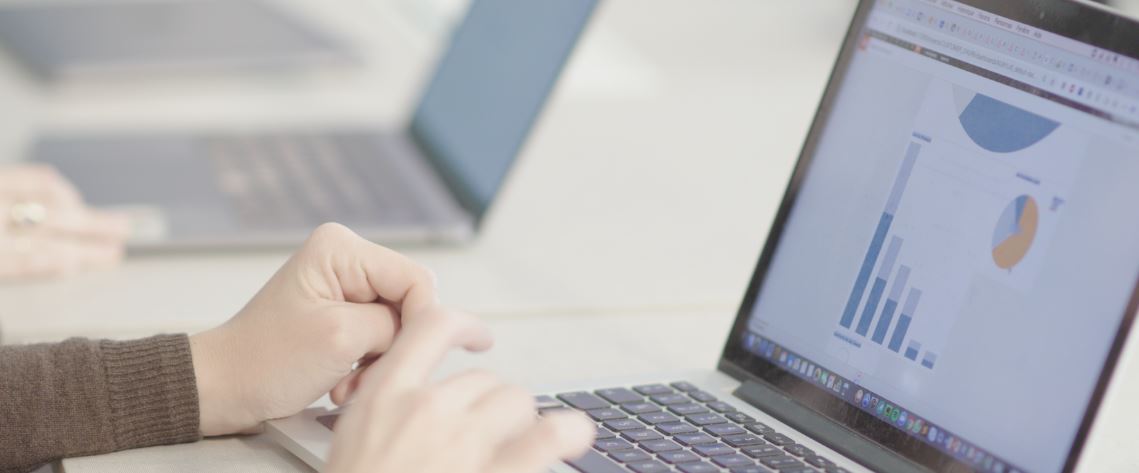
Case Study
Automated Dashboards in Customer Analysis: A Case Study of OVH
OVH, a global provider of hyperscale cloud, faced a significant challenge in analyzing user interactions on their website to inform product and operations decisions. The primary point of contact between OVH and its users was through their website, where customers could place orders and receive technical advice or support. The business analysts responsible for disseminating data and insights to inform on the commercialization and optimization of the website had built a dashboard with basic, high-level metrics like user behaviors and site traffic. However, the dashboard's utility was limited as it did not combine different data sources for a complete view, necessitating ad-hoc analysis. The analysts had little time for this, and the ETL (extract, transform, load) for the dashboard presented concerns for the data architects around data and insights quality. There was a lack of transparency around exactly what data was being transformed and how.
Case Study
Knowledge Management Optimization
L’Oreal, the world’s largest cosmetics company, wanted to optimize the effectiveness of its teams worldwide by improving knowledge transmission at all levels of the group. To achieve this, L'Oreal deployed 'Yammer,' a social web platform developed by Microsoft, for its employees in 2012. Three years later, 23,000 L’Oreal employees were using the internal social network on a voluntary basis. However, to intensify the qualitative aspect of conversations within Yammer, L’Oreal Operations wished to identify conversation leaders and incite actions for business knowledge transmission.
Case Study
Lifetime Value Optimization through Data Centralization: A BlaBlaCar Case Study
BlaBlaCar, the world's first online carpooling booking service, faced a significant challenge in accessing and utilizing their data. The company's Business Intelligence (BI) teams were heavily dependent on IT teams for reporting and analytics. The process of data retrieval was time-consuming and repetitive, often taking days to deliver the requested data. The company's data sources were heterogeneous and scattered, making it difficult for the BI teams to access the data on demand. The challenge was to find a solution that could clean, consolidate, and centralize these data sources for easy and immediate access by BI teams globally.
Case Study
Smart Pricing in Retail
A leading retailer in Europe with more than 3,500 stores and an e-commerce component was losing money due to being undercut by competitors on price. They also found that their customer base tended to wait until the end of seasons for huge markdowns and would only then buy certain seasonal products, which skewed their predictions for how to stock items in the future and perpetuated the pricing issue. In addition, they struggled to efficiently change prices and keep them consistent across stores and online - often, this resulted in inconsistent pricing, especially when individual store managers made their own decisions on sales. The retailer wanted to improve their pricing strategy by understanding what drove customer purchasing decisions for specific products and what prices would resonate best, easily understanding the price offered by all competitors in real time, and updating pricing consistently across stores and online.
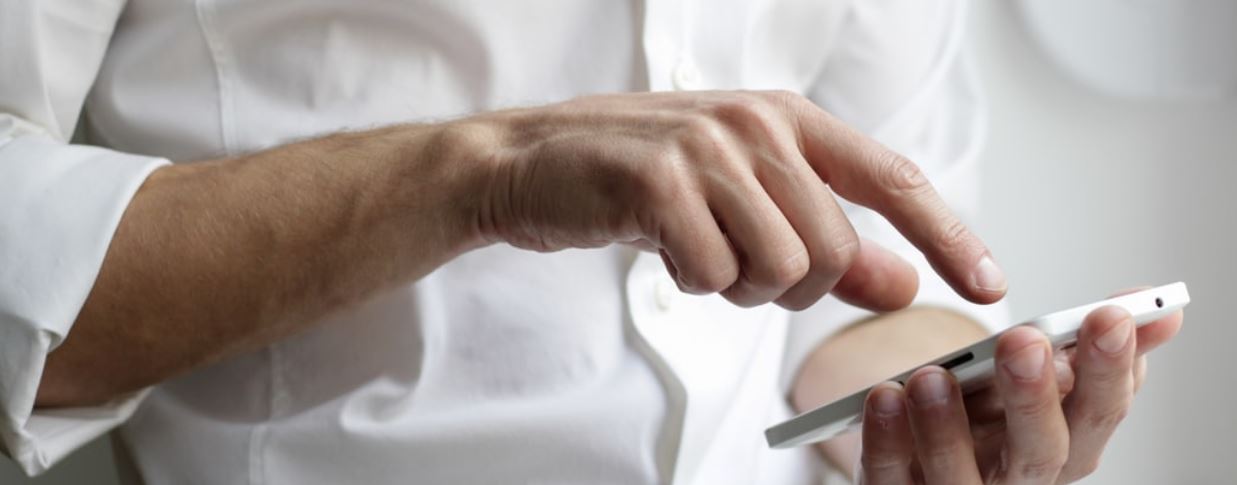
Case Study
Scaling Up Data Efforts With LINK Mobility
LINK Mobility, Europe’s leading provider of mobile communications, wanted to scale up their data efforts in 2017. Their primary offering is mobile messaging services, sending over 6 billion messages a year worldwide. These messages carry invoices, payments, and vouchers, associated with a variety of services. This generates a lot of data, and LINK Mobility saw an opportunity to expand their offerings to provide more data-driven insight to customers surrounding the delivery and performance of their messages and services. They were looking to expand to customer dashboards and send additional offers based on that data. However, with just a one-man data science team at the beginning of the project, LINK Mobility needed to find a tool that would allow them to scale up data requests coming from inside the company and provide data insights to customers without having to use two different tools or platforms.
Case Study
Convex Insurance: Enhancing Collaboration and Decision Making with Dataiku
Convex Insurance, a company that heavily relies on data for decision making, was facing challenges with its traditional data handling methods. The company's diverse team of actuaries, architects, and business analysts needed a more efficient way to collaborate and extract value from their data. The use of spreadsheets was no longer sufficient due to the enormous volume of data and the complexity of the data pipelines. The company needed a solution that could accommodate the varying levels of technical expertise within the team and facilitate effective communication and collaboration.
Case Study
Data Transformation at Rabobank: A Case Study in Execution & Innovation
Rabobank, a leading Dutch bank, was faced with the challenge of keeping up with the rapid pace of technological change in the banking sector. According to a 2020 PwC report, 81% of banking CEOs expressed concern about the speed of technological change, more than any other industry sector. Rabobank, however, chose to embrace this change and transform their organization to move with the pace of innovation. The bank had been on their data journey since 2011, and while they had the support from both the executive level and the people implementing the technology and processes, they needed to further streamline their approach to data transformation.
Case Study
Vestas: Leveraging Dataiku for Sustainable Energy Solutions and Cost Reduction
Vestas, a global leader in sustainable energy solutions, faced a complex challenge in optimizing their shipment patterns to save costs. The Service Analytics team at Vestas had to consider not only external, customer-facing products, but also internal stakeholders across the Operations, Finance, Supply Chain, and Commercial teams. All of these teams worked together to answer big questions for the company such as how and when to deliver a turbine part from point A to point B. The team recognized that a more robust data operation could help them simplify and improve logistical challenges. They understood that data science-based solutions in predictive asset maintenance, field capacity planning, inventory management, demand and supply forecasting, and price planning would provide critical support to the internal customers of Vestas. However, until that point, the data team ran a traditional business intelligence (BI) based analytics operation, querying BI-dashboards, deriving insights, and building data products in a less automated manner.
Case Study
Logistics Optimization through IoT: A Case Study of Chronopost International
Chronopost International, a member of the La Poste group, is a global provider of express shipping and delivery services. The company promises that all parcel deliveries in France will arrive by 1pm the following day after an order is placed. However, as demand continues to grow, especially during peak periods such as Christmas or Mother’s Day, Chronopost faced the challenge of ensuring they can always keep their promise and deliver parcels on time. The company needed a solution that would help them use and analyze historical data to optimize delivery operations and ensure delivery deadlines are met.
Case Study
Dynamic Pricing with Predictive Analytics
PriceMoov, a service that delivers optimal pricing suggestions and solutions to its customers, was facing a challenge with data originating from old SI systems, Oracle, or MySql. The data was dirty and required a fulltime developer to perform long ETL steps in PHP for cleaning. Once cleaned, the datasets were painfully entered into a model, as they were custom-built pipelines. And once finished, the replication and deployment process for the next customer was taking weeks. This long and arduous data preparation process was causing stale pricing recommendations.
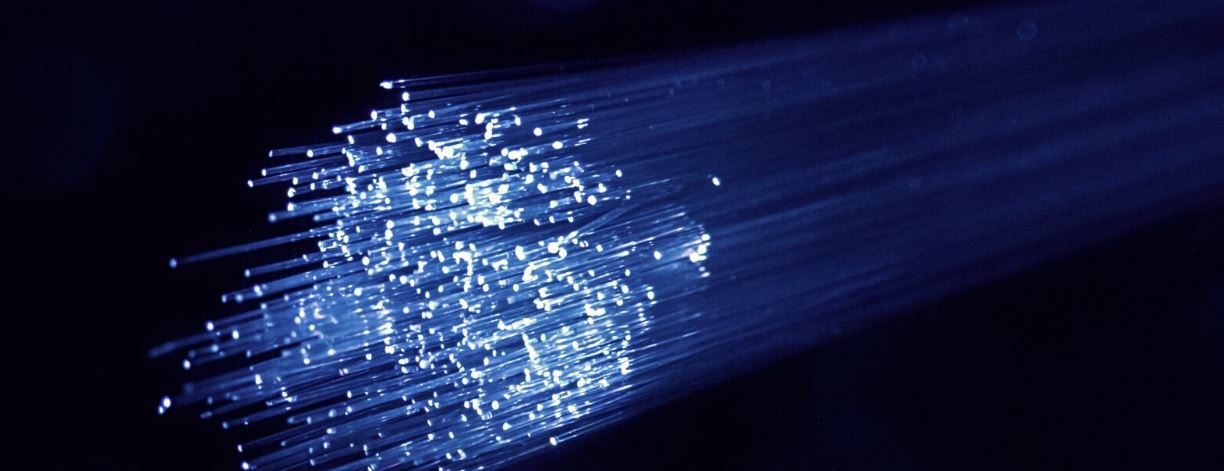
Case Study
Orange: Leveraging Dataiku for Sustainable Data Practice and Machine Learning
Orange, a leading telecommunications company, was facing challenges in its client services department's data science team. The team was primarily performing ad-hoc analysis and had limited capacity to work on complex machine learning-based projects. The challenges were twofold: tooling and hiring. The existing tool was proprietary and could only be used by statisticians or data scientists, making data access difficult and hindering project initiation. It was also not equipped to support machine learning-based data projects. On the hiring front, Orange struggled to attract fresh, ambitious data scientists due to the tooling challenge. Young data scientists preferred jobs where they could work with open-source tools like Python or R. New hires had to learn the legacy tool, which took months before they could start being productive.
Case Study
Online Fraud Detection
SendinBlue, a relationship marketing SaaS solution, faced a significant challenge in validating new customers and ensuring the quality of their databases. The company had to ensure that all contacts on the list were opted in, which required manual validation. This process was not only time-consuming and required a large workforce, but it also severely delayed account validation for customers, damaging SendinBlue’s reputation. As the customer base grew, manual validation became increasingly unfeasible. The company needed a solution that could automate the validation process and scale with the growing demand.
Case Study
Royal Bank of Canada: Streamlining Audits with Dataiku's IoT Solution
The Royal Bank of Canada (RBC) was facing challenges in its control testing process, which was manually intensive and only conducted periodically. The process involved selecting control tests, designing test procedures, sampling the resulting dataset/transactions, and checking samples for adherence to criteria. This process was repeated anywhere from annually to once every two years. The CAE Group, burdened by the administrative overhead, had less time to review and revise the outliers. The process was difficult to scale, as the platforms retreated into their silos, where they built and managed their own control testing process. This duplicated effort made consolidation into CAE Group’s holistic enterprise view a cumbersome, manual process. The challenges were both technical and organizational. Technical challenges included the need for platform analysts to onboard and update their models in production, support for the variability of different models and schemas of outliers, categorization of each control test, and managing data governance requirements. Organizational challenges included a shift in mindset for auditors, updating and onboarding existing control tests, and developing incentives for adopting the new platform.
Case Study
Thrive SPC's Transformation: Leveraging Dataiku, Snowflake, and Snow Fox Data for Improved Clinical Home Care
Thrive Skilled Pediatric Care (Thrive SPC) is a healthcare organization dedicated to providing exceptional clinical home care to medically fragile children. Their mission is powered by innovative technology and in-depth data insights. However, when Thrive SPC acquired two different types of healthcare organizations, they faced a significant challenge: managing multiple electronic medical record systems with different data reporting mechanisms. These complex and disparate systems were impossible to manage individually and manually. Thrive SPC needed a way to prepare and store data in a reliable and accessible manner. The organization was also struggling with competing and confusing spreadsheets, which hindered the efficiency and organization of their data projects.
Case Study
Insurance Fraud Detection: Leverage Data to Accurately Identify Fraudulent Claims
Insurance organizations are constantly exposed to fraud risks, including false claims, false billings, unnecessary procedures, staged incidents, and withholding of information. Santéclair, a subsidiary of several supplementary health insurance companies, was struggling with fraudulent reimbursements from both opticians and patients. They lacked a system that could effectively analyze the right data and adapt to increasingly sophisticated fraudsters. Instead, they relied on “if-then-else” business rules to identify likely fraud cases, which resulted in the manual audit team spending their time on too many low-risk cases. With the increase of reimbursement volume (more than 1.5M a year), they needed to improve their efficiency and productivity.
Case Study
Ensuring Subscriber Retention and Loyalty
Coyote, a French leader in real-time road information, was facing a challenge in retaining its customer base and enhancing its service quality. The company wanted to optimize its loyalty program to encourage customers to increase device use. To achieve this, Coyote needed a technical solution that would enable them to segment its customer base by user profile, qualify incoming data, and quantify device use through anonymous data analysis. The company understood that the more data it collected, the better its service would be. Therefore, improving retention rates was crucial to enhance the service quality and acquire more users.
Case Study
SLB People Analytics: Harnessing Dataiku for Optimized Talent Management
SLB, a global leader in the oil and gas industry, was facing challenges in its People Analytics team. Despite being a technology-centric company, the benefits of technological advancements were not reaching all business units. The People Analytics team, created in 2018, was struggling with scalability issues. Data scientists and engineers were working in isolation, preparing and transforming the same data without sharing insights, leading to a delay in project completion. The lack of a common platform for project recycling was causing a loss of time to market, discovery, and high-value projects. The team was also grappling with the challenge of applying machine learning to their vast talent pool, which required investment in learning and training, compliance monitoring, and stakeholder engagement.
Case Study
Anomaly Detection: How to Improve Core Product Accuracy and Efficiency with IoT
Coyote, the European leader of real-time road information, faced a significant challenge in maintaining the accuracy of speed limit data within their embedded maps. This data is crucial for the functioning of their IoT devices and mobile applications, which warn drivers of traffic hazards and conditions. The company needed an automated, algorithm-based solution that could correct speed limit data and leverage the high volume of incoming data from their IoT devices to generate actionable insights and predictions. This also required instilling a data-driven approach within the company, where decisions are based on real-world data rather than standard analytics reports.
Case Study
Churn Prevention
Showroomprive, a leading e-commerce player in Europe, was facing a challenge with customer churn. The company was using static rules to trigger marketing actions, which were common to all customers and did not take into account the individual value of each client. This approach was not effective in preventing churn and improving customer loyalty. Showroomprive wanted to refine its client qualification process to anticipate, prevent, and reduce churn rates. The company aimed to detect clients with a high potential of no longer buying from the website based on individual purchase rates and refine the targeting of marketing campaigns for each potential churner to improve customer loyalty.
Case Study
Faster, More Accurate Customer Segmentation
Dentsu Aegis is a media buying company that allocates advertisers’ budgets on campaigns across various media using targeted segmentation. When pitching their services to potential customers, the sales staff recommends specific segments that would be the best to target with a particular campaign to maximize return. After they make the sale, the teams need to be able to deliver on those promises and actually maximize return with effective segmentation. However, the department struggled to quickly provide segmentation recommendations to the sales team. The teams built a data lake to collect data from multiple sources, but actually using the data meant embarking on the painful process of writing new code (Python, Spark, or SQL) every time. Every time they had a project, team members had to write a query, get the results, analyze those results with another tool, and write more code to reprocess and use the data. Without an easy way to replicate past work, each project required them to start their process from scratch, no matter how similar two prospects’ or customers’ use cases were.
Case Study
Harnessing Large, Heterogenous Datasets to Improve Manufacturing Process
Essilor International, a leading ophthalmic optics company, was facing the challenge of improving the processes and performance of their surfacing machines to significantly enhance their production. The surfacing step in lens creation is complex and delicate, as it gives the lens its optical function. The company aimed to optimize this step to correspond to each person’s individual prescription and personal parameters. However, they were dealing with large, heterogeneous datasets from the surfacing machines and needed a scalable way to work with this data. The company was already using continuous monitoring technologies like IoT connected devices, but they wanted to take a step further by employing advanced algorithms and machine learning to take action from real-time insights.
Case Study
How The Law Society of BC Uses Dataiku for Risk Ranking and Anomaly Detection
The Law Society of British Columbia, a non-profit organization that regulates lawyers in British Columbia, was looking to increase the efficacy of their trust assurance audit program. The organization regulates 3,800 law firms and audits approximately 550 firms per year, which means that each firm is audited at least every four to six years. The Law Society has three decades of historical data, which enables them to categorize law firms according to their risk level: low, neutral, or high risk. The organization made the decision to focus on risk factors and, from there, work to adjust the audit schedule based on the risk category of each firm. The senior management team at The Law Society of BC firmly believes that AI and machine learning will play an important role in their responsibilities in the near future. They knew it was time to take advantage of their collected data and leverage technology to identify patterns and behaviors and increase effectiveness and efficiencies within Law Society programs.
Case Study
Finexkap: From Raw Data to Production, 7x Faster
Finexkap, a leading fintech providing digital solutions for B2B operators, marketplaces, and e-commerce in western Europe, was facing a challenge with its data science team. The team, consisting of only three data scientists, was using Python in notebooks and a bit of C# to automate processes, but they didn’t have any visual tools for building data pipelines or to conduct on-the-fly data analysis. This method was functional but extremely tedious, and in the long run, they realized it was not sustainable, especially with the company’s growth and plans for future products and expansions.
Case Study
Internal Design & Deployment of Advanced Analytics Solutions at AramisAuto
AramisAuto, a leader in France’s new and second-hand automotive sales industry, was keen on developing its own competitive advantage with data-driven projects. The company decided to internalize the design, development, and deployment of their own data-driven solutions and products. This decision was driven by the need to develop analytics projects internally using newly hired expertise such as business intelligence engineers and data scientists. Due to data sensitivity issues, outsourcing data analysis teams was not a viable option. These new team members needed to quickly get up-to-speed in terms of creating highly-scalable predictive models and applying that knowledge to a wide array of business case scenarios, including real-time deployment of data products.
Case Study
Predictive Content Management for PagesJaunes
PagesJaunes.fr, the French equivalent of the YellowPages, is a leader in local advertising and information on web, mobile, and print, generating hundreds of millions of queries each year. The quality and relevance of results is a top priority for PagesJaunes. Category managers are responsible for maintaining the quality and relevance of the directory by creating the pertinent associations between terms and categories. The challenge was to improve user experience without increasing workload. The client wanted a solution that would help them measure and improve customer satisfaction, help Category Managers automatically detect and correct problematic queries, and optimize the quality of results to improve customer satisfaction.
Case Study
Smart Cities: Enhancing Public Services with DSS
Parkeon, a global supplier of parking and transit systems, wanted to leverage the vast volumes of data they had access to regarding city drivers' habits. They aimed to design a powerful parking availability prediction B2C application that could provide reliable predictions of parking availability and enrich the parking meter data to create greater intelligence. The challenge was to turn the parking meter data and geolocalized data into accurate predictions that could be used in a user-friendly mobile application.
Case Study
Patient Scheduling Optimization (Patient No Show Predictive Analytics)
The healthcare industry is grappling with a high rate of patient no-shows, with studies indicating that 5-10% of scheduled patients miss their appointments. This has a significant impact on the financial health of healthcare organizations and their ability to care for other patients. Primary care physicians lose an average revenue of $228 for every no-show, and the lost revenue for specialists is even higher. When a patient misses an appointment, overhead costs including staffing, insurance, and utilities are not reimbursed. Cancellations with primary care physicians also impact the number of necessary specialist referrals those physicians can make. Combined, these factors contribute to significant revenue loss for physicians. To help minimize the occurrence of no-shows and thus reduce associated costs, Intermedix decided to develop and operationalize a no-show predictor that would assist office managers in scheduling appointments.
Case Study
Improving Fraud Detection by Evangelizing Data Science
BGL BNP Paribas, one of the largest banks in Luxembourg, had a machine learning model in place for advanced fraud detection. However, the model remained largely static due to limited visibility and limited data science resources. The business team was keen on updating the model but faced challenges due to lack of access to data projects and the data team. The challenge was to harness a data-driven approach across all parts of the organization. The bank needed a solution that would democratize access to and use of data throughout the company, without compromising data governance standards.
Case Study
Staffing Optimization
A major healthcare provider in the UK was struggling with staffing inefficiencies, leading to physician overwork, patient dissatisfaction, and high costs. The hospital's staffing process was largely manual and based on the number of available beds, which did not allow for efficient allocation of staffing hours. This lack of data-driven decision making was impeding the hospital's ability to deliver optimal care and retain the best doctors. The hospital sought a technical solution that would enable it to model patient inflows on a small scale and recommend staffing schedules based on patient demand forecasting.
Case Study
Hyper-Targeted Advertising in the Media Industry
Infopro Digital, a crossmedia company, wanted to offer more advanced targeting options to its advertising customers. Instead of basic category targeting, they wanted to leverage the user’s navigation path and behavior to more accurately target those who may be interested in a particular ad. This advanced targeting required experienced technical teams to handle a vast data lake. However, Infopro Digital’s marketing teams needed to be able to handle the queries and most of the day-to-day work themselves without the help of IT every time. The marketing teams had some prior knowledge of processing data using Microsoft Excel, but they were frustrated by its computing and speed limitations. Infopro Digital also wanted to develop any new processes and skills within the company to keep costs and production delays low.
Case Study
Physician Profiling
The customer, a major hospital in Western Europe, was facing challenges in accurately measuring physician and healthcare organizations' performances due to uncoordinated heterogeneous data sources, irregular and poor quality data, insufficient risk-adjustment of results, and lack of automation in physician profiling processes. They were seeking to embrace an Accountable Care Organization (ACO) model to improve clinical outcomes and compete on cost. Some clinical processes, like prescribing expensive or unnecessary drugs or recommending longer hospital stays than needed, were costly and detrimental to patient care. The customer estimated that administering the wrong care at the wrong time represented upward of $1.6M loss per year, a problem that they believed could be solved with accurate physician profiling.
Case Study
Showroomprivé: Putting ML-Powered Targeting in the Hands of Marketers
Showroomprivé, an e-commerce retailer specialized in flash sales, faced challenges in targeting their marketing emails. Until 2016, the team selected the target audience for these marketing emails manually based on what they know about the brand. However, this approach presented several challenges. Brands often have overlapping or broad audiences, which meant touching some prospective buyers multiple times, while others not at all. This also meant casting out a wide net, potentially sending emails to people who were not interested in that particular brand. The ultimate goals of the project was for the marketing team to be completely autonomous in targeting and sending these emails.
Case Study
Malakoff Humanis: Improving Customer Relations With the Power of NLP
Malakoff Humanis, the leading non-profit group health insurer in France, was facing growing challenges in keeping up with customer demands and providing quality customer service. The company offers supplementary health, welfare, and pension contracts to companies, employees, self-employed individuals, and single-payer individuals. It covers healthcare reimbursements in addition to the French social security and guides clients in their choice of care establishments. The company has a dedicated data science and analytics department led by a Chief Data Officer. The data department is comprised of four main branches, each in charge of Data Science and Analytics, Data Governance, Data Architecture and Cloud, and AI and Data Visualization. However, the company was struggling to effectively manage customer claims and improve telephone customer assistance.
Case Study
Coyote: From Churn Analysis to Predictive Safety
Coyote, a European leader in real-time road information, uses IoT-based devices and mobile applications to warn drivers of traffic hazards and conditions. The company collects extensive data on the different uses of its community, such as mileage, time spent on the road, or the number of alerts issued by the community members. Initially, Coyote started with predictive analytics for improving their customer retention. However, they wanted to leverage the value of their vast data sources and implement a data-driven strategy at the heart of their core products and services. They aimed to improve road safety using IoT devices.
Case Study
Action's Journey: Leveraging Data Analytics for Efficient Business Operations
Action, Europe’s fastest growing non-food discount retailer, faced a significant challenge in managing the vast inflow of data from its over 2,300 stores across 11 countries. The company needed to track various aspects such as consumption patterns, product placement, and supply chain disruptions, which varied according to local, national, and international trends. The existing architecture was not sufficient to handle the data efficiently and provide accurate forecasting models for demand and sales in new and existing markets. The company also faced issues with data access and quality, costly and complex processes, lack of visibility and control, and operationalization and business impact. The use of Excel for gathering, sorting, manipulating, and modeling data was proving to be a bottleneck for the speedy and efficient deployment of data analytics and models.
Case Study
JK Lakshmi Cement: Enhancing Operational Efficiency with Dataiku
JK Lakshmi Cement, a decades-old manufacturing firm in the cement industry, was facing significant challenges in improving operational efficiency and accelerating analytics reporting. The company was bottlenecked by a lack of data- and tech-savviness, with only a few people tasked with building reports for the entire organization. This limited the number of reports that could be created, hindering the company's ability to make data-driven decisions. The team was also struggling with scarce and underutilized data experts, and their data processes lacked operationalization and the ability to make a strong business impact. They were in need of a platform that could boost the efficiency of its coders and allow for cross-team collaboration with line-of-business users.
Case Study
Marketing Efforts 360° View
Trainline, Europe’s leading independent train travel platform, was facing a challenge in monitoring and improving their marketing acquisition. With paid campaigns running 24/7 and users interacting with those ads around the clock, static dashboards were no longer sufficient. The company needed a dynamic, real-time data tool for accurate marketing insights. They had invested in many different services and solutions to sustain their growth, but these were not always easy to manage. The company decided to build a centralized, global, real-time dashboard to get a global understanding of their marketing acquisition. The challenge was to start a big data project from scratch, ensuring that the technical team ended up with a tool that allowed them to improve and upgrade their own skills while also satisfying the marketing department’s requests quickly and efficiently.
Case Study
Smart User Segmentation for Targeted Recommendation
Voyage Privé, a boutique vacation retailer, faced the challenge of creating personalized offer displays for its customers. The company needed to expand the range of customer signals that could be captured and analyzed to offer travel options that were appropriate for their members. This required a software solution that could capture and make sense of large amounts of data, develop effective customer segmentation, and implement a new non-rule-based approach for analyzing incoming and historical data. The end goal was to increase customer satisfaction by providing users with personalized offer selections while simultaneously boosting the total transaction value by customer.
Case Study
Rely on Automation for Scalability
A large national media organization wanted to provide high-quality recommendations for users of their app. Their goal was to target consumers with content that they would actually be interested in based not only on what they previously consumed, but how exactly they interacted with topics in which they previously expressed interest. For example, if someone chose to listen to a report on Topic A but then fast forwarded through much of the piece (as opposed to actually listening to the piece in its entirety), the app should take that activity into account for future recommendations. However, with a very small team and limited resources, the organization wanted to accomplish this in a scalable way. Not only would the system have to be mostly or entirely automated, but the team itself would have to be able to build the recommender easily in a way that would allow for quick tweaks and adjustments in the future.
Case Study
Revenue-Generating Data Projects from the Ground Up
In 2017, LINK Mobility, Europe’s leading provider of mobile communications, decided to scale up their data efforts for handling internal requests and externally with customers. Their primary offering is mobile messaging services, sending more than 6 billion messages a year worldwide carrying invoices, payments, and vouchers, associated with various services. They produce a lot of data and saw an opportunity to expand their offerings to provide more data-driven insight to customers surrounding the delivery and performance of their messages and services. They were looking to expand to customer dashboards as well as the ability to take action based on that data. However, with just a one-man data science team at the beginning of the project, they needed to be able to get up and running quickly and easily. They also needed to find a tool that would allow them to scale up data requests coming from inside the company as well as to be flexible enough to provide data insights to customers without having to use two different tools or platforms to cover their various needs, use cases, and data types.
Case Study
Scaling a Small Data Team with the Power of Machine Learning
DAZN, a subscription sports streaming service, was looking to grow their business in existing and new markets. They wanted to enable their small data team to run predictive analytics and machine learning projects at scale. They also wanted to find a way to allow data analysts who were not necessarily technical or experienced in machine learning to contribute in meaningful ways to impactful data projects. The goal was to support an underlying data culture with advanced analytics and machine learning at the heart of the business.
Case Study
Faster, Higher Quality Dashboards for Better Customer Analysis
OVH, a global provider of hyperscale cloud services, was facing challenges with its dashboarding system. The business analysts responsible for disseminating data and insights to inform the commercialization and optimization of the website were spending more than 80% of their time on data preparation for the dashboard. The existing dashboard only provided basic, high-level metrics and did not combine different data sources for a complete view. This necessitated ad-hoc analysis, for which the analysts had little time. Additionally, the ETL process for the dashboard presented concerns for the data architects around data and insights quality, as there was a lack of transparency around exactly what data was being transformed and how.
Case Study
Real-Time Predictions for Targeted Safety Oversight
Technical Safety BC, an independent, self-funded organization, oversees the safe installation and operation of technical systems and equipment across British Columbia, Canada. Conducting physical assessments is costly, and false positive inspections can result in significant opportunity costs each year. Those same resources could be better allocated within the safety system; therefore, finding a way to more accurately predict hazards is of high strategic value to the organization, and it creates greater safety benefit to the public. Technical Safety BC was looking to find more high-hazard sites while operating at the same resourcing level by introducing more sophisticated machine autonomy in the risk assessment process. Some of the challenges faced included: uncoordinated heterogeneous data sources; data quality; speed of collaboration; and training challenges in the use of machine-recommended predictions.
Case Study
Heetch + Dataiku: Developing an Elastic AI Strategy
Heetch, a French company founded in 2013, has grown quickly to 250 employees united around one goal: making mobility more accessible by offering a smooth user experience. The company has gathered troves of data from drivers, passengers, global operations, and more since its launch, yet they struggled to scale their ability to actually leverage that data. Five years in, data warehouse costs were spiraling out of control, and performance was suffering as the amount of data grew. The company needed to find a solution that would allow anyone across the organization to work with large amounts of data while also ensuring optimized resource allocation.
Case Study
Dataiku + La Mutuelle Générale
La Mutuelle Générale, a French insurance company with over 70 years of experience in the market, serving over 1.4 million customers and 8,000 enterprise clients, and generating more than €1.1 billion in turnover annually, was facing a challenge in customer acquisition. The competition in the insurance industry is fierce, with organizations all vying to capture the same type of customer. The cost of acquiring a new customer has significantly increased in recent years. To address this, La Mutuelle Générale sought to develop a decision support tool for sales to aid their understanding and prioritization of prospects based on their likelihood to convert and their potential value compared to their cost of acquisition.
Case Study
MandM Direct: Managing Models at Scale with Dataiku + GCP
MandM Direct, one of the largest online retailers in the United Kingdom, faced a significant challenge as they grew rapidly. With over 3.5 million active customers and seven dedicated local market websites across Europe, the company delivers more than 300 brands annually to 25+ countries worldwide. Their accelerated growth meant more customers and, therefore more data, which magnified some of their challenges and pushed them to find more scalable solutions. The two main challenges were getting all the available data out of silos and into a unified, analytics-ready environment and scaling out AI deployment in a traceable, transparent, and collaborative manner. Initially, the company's first machine learning models were written in Python (.py files) and run on the data scientist’s local machine. However, as the number of models in production increased, the team quickly realized the burden involved in maintaining models.
Case Study
Provincie Noord-Holland: Scaling Data Science in the Public Sector
Provincie Noord-Holland (PNH), a province in the Netherlands, embarked on an initiative to become a more data-driven organization. However, they faced challenges in determining the necessary steps to achieve this goal, including the required technology and expertise, setting up experiments, and implementing new processes. They also faced unique challenges as a public sector organization, such as the need to consider regulations and societal impact when conducting experiments and working with data. Additionally, they had to work within a closed IT environment, limiting their access to data science tools. They also realized the need for data scientists and technology to help them succeed with their data science initiatives, and the importance of being both data and business-driven to generate positive performance and encourage buy-in among organization-wide stakeholders.
Case Study
Buildertrend: Maximizing Data Project Speed to Value
Buildertrend, a leading construction project management software company, was looking to disrupt the residential construction industry by leveraging data science to improve business operations and make residential contractors more efficient. They were seeking a data science platform that could enhance speed and agility in their data-to-insights process, enable company-wide collaboration on data projects, and empower their data scientists with the right tools and resources. The company was also keen on automating repetitive tasks, improving documentation practices, and increasing the amount of data included in their models. One of their key use cases was churn reduction, where they aimed to efficiently target at-risk accounts to drastically reduce churn.
同类供应商.
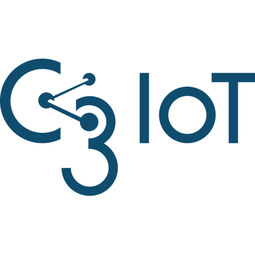
Supplier
C3 IoT
C3 IoT provides a full-stack IoT development platform (PaaS) that enables the rapid design, development, and deployment of even the largest-scale big data / IoT applications that leverage telemetry, elastic Cloud Computing, analytics, and Machine Learning to apply the power of predictive analytics to any business value chain. C3 IoT also provides a family of turn-key SaaS IoT applications including Predictive Maintenance, fraud detection, sensor network health, supply chain optimization, investment planning, and customer engagement. Customers can use pre-built C3 IoT applications, adapt those applications using the platform’s toolset, or build custom applications using C3 IoT’s Platform as a Service.Year founded: 2009
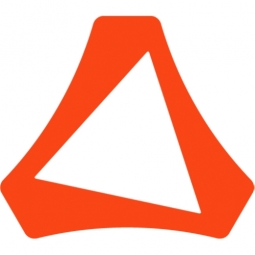
Supplier
Altair
Altair is a leading provider of enterprise-class engineering software enabling innovation, reduced development times, and lower costs through the entire product lifecycle from concept design to in-service operation. Our simulation-driven approach to innovation is powered by our integrated suite of software which optimizes design performance across multiple disciplines encompassing structures, motion, fluids, thermal management, electromagnetics, system modeling and embedded systems, while also providing data analytics and true-to-life visualization and rendering.
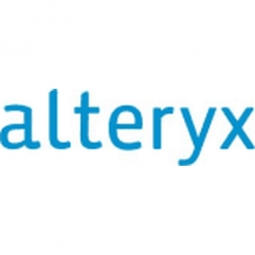
Supplier
Alteryx
Alteryx, Inc. was formed in 2011 and is a leader in self-service Data Science and analytics. Alteryx provides analysts with the unique ability to easily prep, blend and analyze all of their data using a repeatable workflow, then deploy and share analytics at scale for deeper insights in hours, not weeks.Analysts love the Alteryx Analytics platform because they can connect to and cleanse data from data warehouses, cloud applications, spreadsheets and other sources, easily join this data together, then perform analytics – predictive, statistical and spatial – using the same intuitive user interface, without writing any code.