DataRobot
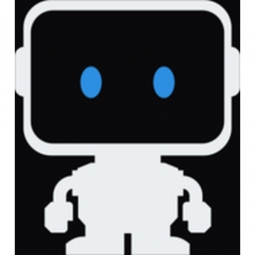
概述
总部
美国
|
成立年份
2012
|
公司类型
私营公司
|
收入
$10-100m
|
员工人数
201 - 1,000
|
网站
|
推特句柄
|
公司介绍
DataRobot 为所有技能水平的数据科学家提供了一个机器学习平台,以在过去的一小部分时间内构建和部署准确的预测模型。该技术通过改变预测分析的速度和经济性来解决数据科学家的严重短缺问题。DataRobot 平台使用大规模并行处理来训练和评估 R、Python、Spark MLlib、H2O 和其他开源库中的 1000 个模型。它搜索数百万种可能的算法、预处理步骤、特征、转换和调整参数组合,为您的数据集和预测目标提供最佳模型。 DataRobot 平台评估数百种尖端机器学习算法,以发现、部署和定制适合各种情况的最佳机器学习模型。它还大规模提供最准确的见解,为各种规模的组织提供通往数据科学成功的最快途径。 DataRobot成立于2012年6月,总部位于美国马萨诸塞州波士顿。
物联网解决方案
主要客户
埃森哲、Aegon、德勤、哈佛商学院、松下、联想、联合航空
物联网应用简介
技术栈
DataRobot的技术栈描绘了DataRobot在基础设施即服务 (iaas), 平台即服务 (paas), 和 分析与建模等物联网技术方面的实践。
-
设备层
-
边缘层
-
云层
-
应用层
-
配套技术
技术能力:
无
弱
中等
强
Supplier missing?
Start adding your own!
Register with your work email and create a new supplier profile for your business.
实例探究.
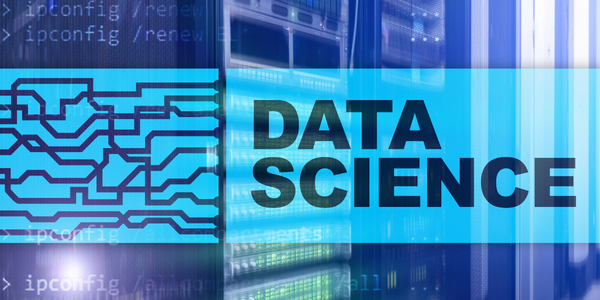
Case Study
Demystifying Data Science: A Case Study on DemystData and DataRobot
DemystData, a New York-based software company, is dedicated to demystifying data for its clients, particularly financial institutions. Despite the increasing use of data in the financial sector, it is still heavily underutilized, leading to business decisions being made based on suboptimal or incomplete data. DemystData aims to close this gap by providing clients with access to new and more data. However, as datasets grow larger and data sources become more varied, the complexity increases, leading to more time-consuming work for the limited pool of data science resources at the company. The challenge was to manage this increasing complexity and workload without compromising the quality of data analysis and insights.
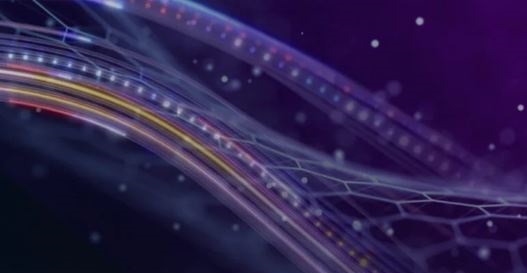
Case Study
Accelerates Data Discovery, Testing, and Deployment
As datasets get bigger and data sources more varied, complexity increases and work processes become more time-consuming. Demyst clients need help identifying which external data attributes are predictive in marketing, risk, and portfolio management use cases across the vast ocean of external data.
Case Study
84.51° Enhances Personalized Shopping Experience for Kroger Shoppers with DataRobot
84.51°, a retail data science, insights, and media company, was faced with the challenge of creating more personalized and valuable experiences for shoppers across the path to purchase. This included the entire customer journey, from initial awareness to activation, retention, and beyond. The company's goal was to leverage 1st-party retail data from over 62 million U.S. households to fuel a more customer-centric journey. However, the sheer volume of data and the complexity of creating personalized experiences for such a large customer base presented a significant challenge. The company needed a solution that would enable better production, deployment, and interpretation of data science models to meet its objectives.
Case Study
Flexiti Enhances Customer Insights with AI: A Case Study
Flexiti, a rapidly growing company in Canada, is recognized as the country's leading provider of point-of-sale financing with buy-now, pay-later solutions. Despite its success, the company faced a significant challenge. It sought to empower its talented risk and analytics team to gain greater visibility into data more quickly. The need for faster and more efficient data insights was crucial to maintain its competitive edge and continue its growth trajectory. The challenge was not only to speed up the data analysis process but also to ensure the accuracy and reliability of the insights derived from the data.
Case Study
Zidisha is Transforming Lives with DataRobot
Zidisha, a non-profit online microlending community, aims to transform the lives of people in some of the poorest countries by offering microloans to create businesses, attend school, or improve their living conditions. However, every loan carries the risk of default. Traditional lenders have found ways to identify, quantify, and price default risk, with higher risk loans attracting higher interest rates. The work of assessing risk commonly falls to a loan officer and the costs are passed on to the borrower. In developed economies where loans of thousands or hundreds of thousands of dollars are common, these costs can be comfortably absorbed without undermining the case for taking a loan, but this is not the case in developing countries. Employing a loan officer to assess default risk for a microloan results in interest rates as high as 40%, undermining promotion of economic development. Zidisha's challenge was to improve levels of repayments by identifying applicants most likely to be high-risk borrowers.
Case Study
Hitting the Bullseye on Cause Marketing with Predictive Analytics
DonorBureau, a small company that provides modeling and segmentation services to nonprofits, was facing the challenge of providing more effective and accurate predictive models to differentiate itself in a competitive market. The company was dealing with over 900 million mail transactions, 140 million donations, and over 40 million individuals, and the predictive modeling demands were mounting. Ideally, they would like to have a large team of data scientists on staff, but those are coveted positions that come at a premium. Building and deploying predictive analytics is time-consuming, budget-breaking, and for the layperson, challenging to implement and maintain.
Case Study
Avant Democratizes Data Science with DataRobot
Avant, an online lending platform, has been using data and machine learning to make smart loan decisions. However, as the company wanted to scale its business, it faced the challenge of maintaining the quality and sophistication of its analytics. The company needed a solution that would allow its analysts and business users to access data science tools that could be leveraged by the business teams. Avant was looking for a solution that was easy to use, statistically sound, supported by a reliable company, and simple to integrate with production systems.
Case Study
Speeding up the Predictive Analytics Process with Automated Machine Learning
Evariant, a rapidly growing SaaS company in the healthcare provider market, delivers a suite of innovative CRM solutions that help healthcare systems identify and execute on the most important strategic growth initiatives. However, the company faced a challenge in building and deploying predictive analytics, which can be costly and time-consuming. The complexity of their healthcare data demanded a high level of hands-on data preparation, making their existing solution adequate, but not optimal. They needed high-quality predictive analytics that could be generated both automated and semi-automated — and with an extremely high degree of reliability and validity.
Case Study
Lenovo Computes Supply Chain and Retail Success with DataRobot
Lenovo, a multinational technology company, was facing a challenge in balancing supply and demand for its products among Brazilian retailers. The company aimed to predict the sell-out volume, the number of units of a product that retailers sell to customers, but was constrained by resources. The team had started developing R code to predict sell-out volume, with a goal to have it updated weekly for their top ten retail customers. However, with only 2 people writing 1,500 lines of R code for one customer each week, reaching their target of predictions for ten customers each week was impossible. The team needed to either invest in more data scientists or find a tool that could automate all the modeling and forecasting steps.
Case Study
Teaching Predictive Analytics at the University of Colorado
Predictive analytics is reshaping business and society, raising serious questions about how colleges and universities should prepare graduates. One answer may be to teach predictive analytics to all business school students. What would it take to implement this important vision and why is it not currently being done? As a business analytics professor, Kai Larsen’s goal is to teach a mixed range of students: those who immediately understand how predictive analytics has reshaped their future jobs (Information Management and Marketing), those for whom different flavors of business analytics have long since infused into the core of their fields (Operations Management and Finance), and those for whom predictive analytics currently is reshaping “only” a small part of their discipline (Accounting). It is becoming clear that all of these students must, at a minimum, understand predictive analytics conceptually to make decisions that will affect the future of their companies as machine learning tools continue to provide business insights and drive change within and outside the enterprise.
Case Study
How the Philadelphia 76ers Win Off the Court Using Machine Learning from DataRobot
The Philadelphia 76ers, a professional basketball team in the NBA, is part of a new wave of sports franchises that are leveraging data analytics to optimize both their on-court performance and business operations. The organization has a strong focus on using data to inform decision-making processes across all levels. One of the key challenges faced by the 76ers' Analytics Team was improving the efficiency of their season ticket renewal process. The team had been using data science and simple modeling techniques, but lacked a dynamic machine learning tool that could adapt and learn as more data was collected. This meant that the team had to do a lot of work in the offseason to produce a static model. The goal was to transform the renewal process from a once-a-year event into a year-round retention process.
Case Study
DataRobot Helps D&G Find Success When the Price Is Right
Domestic & General (D&G), a specialist in providing warranties for household appliances, was facing a challenge in personalizing and delivering relevant offers to its customers. With 9 million customers in the UK and 16 million globally, the company was resource-constrained for the scale of personalized customer service and offerings they were trying to reach. The company's pricing team had to build a lot of models for each customer, which was a laborious and time-consuming process. D&G wanted to predict the likelihood of churn when customers are up for renewal and determine the price point at which customers are most likely to be happy with the warranty coverage they receive and renew their policies. However, delivering this level of personalization to individual customers required building a lot of pricing models, which was not scalable with their existing resources.
Case Study
Independent Model Validation through DataRobot’s AI Services
The fintech company, based in the US, was facing challenges in aligning their business process to regulatory compliance requirements. They were using machine learning models for decision-making, which increased the stakes due to the highly regulated nature of the industry. The company was already using DataRobot’s Enterprise AI platform to improve their model-building, but they needed to accelerate the alignment of their business process to model risk management regulation. They had several models built on DataRobot’s platform and deployed into production, including an internal credit score model, a fraud score model, and a dealer score model. However, they needed an independent model validation after partnering with a bank, which was a critical component of their partnership.
Case Study
Predicting Carpark Capacity at Ascendas-Singbridge Using Machine Learning
Ascendas-Singbridge Group (ASG), a leading sustainable urban and business space solutions provider in Asia, was facing a challenge with parking capacity at their properties. In densely populated cities like Singapore, parking capacity is a major issue. Despite having high-rise buildings with carparks or garages, parking capacity remained a challenge for both property managers and drivers. ASG wanted to forecast and predict parking lot capacity to optimize their parking services, improve the experience for visitors and drivers, and potentially increase revenue. They had previously used a different platform for model building, but it was costly and did not deliver the accurate predictions they needed.
Case Study
Snowflake + DataRobot Unlock the Value of Data at Beacon Street Services
Beacon Street Services, the services division of Stansberry Holdings, provides subscription-based publications of financial information and software to millions of investors globally. The company had a vision to have one single source of truth for all of its data, housed within Snowflake, to ensure consistency and accuracy across all applications of that data. Having migrated from AWS Redshift to Snowflake several years ago, the company had collected and stored great volumes of data within Snowflake. However, the company realized there was value to applying a data science approach to this data, especially for its marketing and sales teams. There was an opportunity to improve on previous tactics and processes of selling subscriptions, with a clearer feedback loop and signal for marketers to optimize their campaigns.
Case Study
Innovation in Investment Banking Through AutoML
Tommy Tan, CEO of TC Capital, a leading Pan-Asian boutique investment firm specializing in M&A and negotiated capital investments, was dissatisfied with the traditional methods of valuing firms used in investment banking. These methods, which include comparing past mergers and acquisitions, looking at stock market valuations of similar companies, and discounted cash flow models, were manually intensive and carried a high risk of human error. They could also lead to highly subjective valuations. Tommy and his team wanted to build their own valuation methodology, one that utilized cutting edge technology and took advantage of the amount of data available to bankers today.
Case Study
The National Association of REALTORS® Brokers Value for Members with DataRobot
The National Association of REALTORS® (NAR) is America’s largest trade association, representing over 1.4 million members around the country. Their members include brokers, salespeople, property managers, counselors, and others engaged in all aspects of the real estate industry. With so many members from unique backgrounds with varying professional interests, each looking for something different out of their membership, delivering value to them requires NAR to truly know their members well. To do that, NAR turned to the data. However, the association was trying to become more data-driven, and so was focused on higher-level objectives like understanding its members better and solving business problems that impacted its members. But because of the nature of how the two data scientists operated — without a centralized team or the appropriate resources - communication and feedback loops around data science projects were inefficient, and negatively impacted the ability of the data scientists to deliver value.
Case Study
How Florida International University Predicts the Future to Help At-Risk Students
Florida International University (FIU), one of the largest universities in Florida, was facing a challenge in identifying and assisting at-risk students. Many of their students come from low-income areas, are the first in their family to go to college, or are the first of their family to enter the country. These factors often present obstacles that make it difficult for these students to progress. The university's analysis was more reactive than proactive, identifying students who had already faced academic or financial obstacles. The university wanted to be more proactive with data to better serve their students.
Case Study
DataRobot In the Classroom
Smith School of Business at Queen’s University in Canada is known for its innovative approach to business education, including creating ground-breaking programs and courses in emerging areas including artificial intelligence, fintech, analytics, cultural diversity, team dynamics, social impact and more. Anton Ovchinnikov, Distinguished Professor of Management Analytics at the Smith School of Business, teaches courses in predictive modeling, data science and machine learning. His students are typically working professionals who are consumers of analytics, not producers. Many of them are, or will soon be, managers of analytical projects and teams. As part of Anton’s courses, he wants his students to familiarize themselves with the raw coding, at least at a basic level, in order to fully understand what’s behind the curtain of what they’re trying to predict. However, the manual coding process can be time-consuming and complex, leading to a need for a more efficient solution.
Case Study
How Consensus, a Target subsidiary, simplified data wrangling for machine learning
Consensus Corporation, a subsidiary of Target, simplifies the complex process of selling connected devices. However, a major risk for retailers selling expensive devices and services is fraudulent customer activity. To address this risk, Consensus adopted fraud prevention as one of its core services. Through its automated machine learning-powered online engine, Consensus can alert its retailer clients to high-risk consumers before they purchase expensive devices. To identify potential fraud, Consensus built an advanced data model that leverages huge volumes of disparate data and undergoes routine updates. In order to be able to constantly refine its predictive models and alert their retailer clients faster to potential fraud, Consensus sought out technologies that would allow it to prepare this data faster for use in its machine learning models. The painstaking process of re-engineering SQL scripts took Consensus up to six weeks (on average) to update its fraud detection machine learning model. In addition, the data preparation process required sophisticated knowledge of data science techniques, leaving the company’s product and business intelligence teams unable to perform data preparation tasks on their own.
Case Study
Using Explainable AI to Revolutionize the Recruitment Industry and Candidate Experience
The Adecco Group, UK & Ireland, a part of the Global 500 ranked company, The Adecco Group, was facing an efficiency problem in their recruitment process. The traditional recruitment process involved multiple manual interventions, which were prone to mistakes and human interpretation. Recruiters had to sift through high volumes of CVs, making it difficult to match the right candidates to the right job. With recruiters working full throttle, it was easy for data-driven insights to remain hidden. The company was looking for a solution to reduce time and speed to fill open positions and improve their hiring attraction pipeline for client talent pools.
Case Study
UCSF-BASIC uses DataRobot and Operating Room Data to Predict the Outcomes of Patients with Traumatic Spinal Cord Injuries
The University of California, San Francisco's Transforming Research and Clinical Knowledge in Spinal Cord Injury (TRACK-SCI) team is dedicated to improving patient care for individuals with traumatic spinal cord injuries. Each year, there are 17,000 cases of spinal cord injury (SCI) in the United States, often resulting in permanent challenges such as paralysis and sensory dysfunction. The estimated lifetime costs for each individual patient can range from just over $1 million to nearly $5 million. Acute clinical decisions made throughout SCI patient care, such as during surgery and ICU management, are critical for setting a patient up for recovery. However, clinicians lack guidance developed through data-driven research. One area of particular interest to the TRACK-SCI team is how blood pressure management during operating procedures affects a patient’s likelihood to recover.
Case Study
Kiva Uses DataRobot to Increase Microloan Funding Rate
Kiva is a financial services nonprofit that uses crowdfunding to underwrite loans for people who are underserved by traditional channels. The World Bank estimates that approximately 1.7 billion people are unbanked, meaning they do not have access to financial services offered by retail banks. This leaves many people without access to the financial instruments that much of the world takes for granted, such as credit cards and loans. Alternative banking methods tend to have high fees that can put them out of reach for the people that need them. This lack of capital hinders economic growth, opportunity, and equality in the places that need it the most. The key to Kiva’s mission is to ensure that those who apply for loans are successfully funded.
Case Study
Anacostia Riverkeeper Uses DataRobot to Predict Water Quality in the Anacostia River
Anacostia Riverkeeper is a nonprofit organization dedicated to protecting and restoring the Anacostia River, which runs through Washington, DC and parts of Maryland. The river is heavily polluted, and swimming has been illegal since the 1970s due to health concerns about pollution. The current methods for testing water quality take days to return results, creating a delay between when the water is tested and when the results are shared with the public. Moreover, water quality can rapidly change with weather conditions, such as rain, making test results outdated before they’re even returned. Anacostia Riverkeeper needed a more efficient and timely way to monitor and predict water quality in the Anacostia River.
Case Study
US Foods Analyzes Transactions from 300,000 Customers with Snowflake and DataRobot
US Foods, one of America's largest food companies, was facing significant challenges with its legacy, on-premises data warehouse. The system required constant maintenance, experienced frequent resource contention, and could not affordably store more than two years’ worth of data. Business analysts took weeks to prepare a single report due to the system’s counterintuitive user interface, inability to load large data sets, and limited BI features. Reporting delays led some business users to seek insights from siloed Microsoft Access databases and Excel spreadsheets. Data science modeling to predict customer loyalty and churn rate was simply impossible. US Foods evaluated several cloud data management solutions, but none offered the right mix of performance and affordability.
Case Study
Harris Farm Markets Taps DataRobot for Demand Forecasting
Harris Farm Markets, a grocery retailer in New South Wales, Australia, faced significant challenges in managing its perishable inventory due to unpredictable supply caused by wildfires and sudden spikes in demand due to COVID. With over two dozen stores and an expanding geographic footprint, the chain needed a way to consistently meet consumers’ demand for variety and freshness. The task of predicting demand for their 20,000 SKUs, including a subset of concurrent fresh produce running at 1200, was too vast for a manual approach. The company sought a solution that could provide accurate predictions with minimal labor on the part of the IT team.
Case Study
Optimizing Loan Predictions with DataRobot AI Apps
The fintech company provides consumer financing to merchants and consumers at point-of-sale through more adaptable alternatives to traditional lending programs. They built models to support the company’s projects in various departments including underwriting, accounting, and collections. However, they faced a challenge in the collections department. With tens of thousands of delinquent loans at any given time, there are a lot of calls for the Collections team to make. The more successful calls they have — measured by an industry metric called Right Party Contact (RPC) — the more likely they are to be able to successfully collect on these delinquent loans, and thus bring in revenue for the company. However, with such a great volume of target calls to make and generally low connection rates in terms of reaching the right person or party, any type of optimization or efficiency can make a big difference.
Case Study
Empowering Life Insurers with Epigenetics and AI
FOXO Technologies is a biotechnology company that aims to make longevity accessible to all using epigenetic science. They use machine learning to examine thousands of models to find patterns of DNA methylation that classify human health, wellness, disease, and aging. Their mission is to help people live longer, healthier lives. However, the data science team at FOXO found it challenging to scale as they looked to build thousands of predictive models based on 860,000 DNA probes. They needed a solution that could help them build, fine-tune, deploy, and manage models in production at scale.
Case Study
OYAK Cement Boosts Alternative Fuel Usage from 4% to 30% — for Savings of Around $39M
OYAK Cement, a leading Turkish cement maker, was facing a significant challenge. The company operates 18 plants in six countries with a production capacity of 33 million tons of cement each year. It was estimated that up to eight percent of CO2 emissions stem from manufacturing cement, the raw material needed for concrete. This was a major concern for OYAK Cement as it was contributing to the environmental problem and also risking costly penalties from exceeding government emissions limits. The company recognized that increasing operational efficiency by five percent would result in four to five percent cost-savings, along with reducing CO2 output by two percent — preventing the release of nearly 200,000 tons of CO2 emissions and eliminating $10M+ worth of CO2-related social impact costs per year.
Case Study
Florida International University Triples Graduation Rates by Aiding At-Risk Students
Florida International University (FIU) is a top-50 public university that serves a diverse student body of more than 58,000 and 260,000 Panther alumni. Many of these students come from low-income areas or may be the first generation to attend college. The university has a proactive approach to keep students in school, which depends on spotting signs of trouble. However, the previous modeling tools used by FIU produced inaccurate results and required exhaustive manual input. The out-of-the-box solutions weren’t tailored to the nuances of their institution, they would flag students that weren’t actually at-risk.
Case Study
Euskaltel Attracts, Keeps Customers with AI-Powered Offers
Euskaltel Group, a leading telecommunications company in Spain, was planning a nationwide expansion. The company needed a scalable way to use AI and machine learning to attract and retain customers, reduce the incidence of default, and identify cross-selling opportunities. Their business intelligence team had experimented with AI on a limited basis but still spent considerable time writing code. The challenge was to find a more efficient and effective way to use AI and machine learning in their workflow.
Case Study
Valley Bank Reduces Anti-Money Laundering False Positive Alerts by 22%
Valley Bank, a regional bank with approximately $50 billion in assets, was facing a challenge in its Anti-Money Laundering (AML) department. The bank was dealing with an overwhelming volume of false positives in its effort to uncover money laundering activities across millions of transactions. The bank's AML team was seeking to reduce the manual work involved in predictive modeling. The process of creating models manually was time-consuming, taking weeks to complete. The bank was looking for a solution that could automate its fraud detection process and manage the volume of false positives in a realistic way.
Case Study
Matmut Derives Data Insights 3 Times Faster
Matmut, a major player in the French insurance market, relies heavily on data to elevate nearly every area of the company. However, the company was facing challenges in deriving insights within the limits of stringent privacy regulations. Matmut’s data lab was building predictive models with a single Jupyter notebook, a process that was manual and required considerable coding. This approach was not efficient and did not foster collaboration between data scientists and the business. The company was in need of a single solution that could reduce the effort and enable collaboration.
Case Study
World’s Largest Car-Sharing Marketplace Maximizes Guest, Host Experience with AI
Turo, the world’s largest car-sharing marketplace, sought to optimize its operations by leveraging data insights. The company connects guests and vehicle owners for mutual benefit across the US, Canada, and the UK. With over 1.3 million active guests and over 85,000 active hosts powering more than 160,000 active vehicles across 1,300 unique makes and models, Turo needed a way to efficiently manage its vast operations. The company aimed to optimize pricing, risk, and marketing strategies using data insights. However, the sheer scale of its operations presented a significant challenge in terms of data management and analysis.
Case Study
U.S. Army Increases Financial Agility with AI by Reclaiming Funds for High Priority Projects $2.2B+ in excess funds identified at a 3x higher yield
The U.S. Army was facing a challenge of identifying funds that were potentially going to be lost due to expiring contracts. They needed an innovative AI solution that could help contracting officers accurately predict the contracts most likely to underspend their funding so they could quickly deobligate and reallocate these funds to other high priority projects. The Unliquidated Obligation (ULO) project was born out of the Army’s HQ Analytics Lab (HAL) and Deep Green OBT initiatives.
Case Study
Profitable Sustained Growth Aided by AI and Machine Learning
MinterEllison, a multinational top-tier law and professional services firm, was looking to grow profitably and sustainably as part of its 2025 strategy. The firm, which operates in five countries, needed a more sophisticated, predictive lens to understand what might happen, especially in the wake of the COVID-19 pandemic. The firm's existing data analytics platform was not sufficient for this task. The firm's Head of Data and Analytics, Shaheen Saud, emphasized the need for a good understanding of performance and opportunities, which prompted MinterEllison to take an innovative look at its IT and digital services infrastructure.
Case Study
Embrace Home Loans Doubles Its Return on Marketing Investment (ROMI) with DataRobot Zepl
Embrace Home Loans, a prominent mortgage lender licensed in all 50 states and the District of Columbia, sought to optimize its marketing spend across its digital and direct mail channels. The company wanted to maximize marketing spend and increase revenue across all marketing channels. The challenge was to do so across the scale of Embrace’s operations, which was a significant task. The company needed a solution that could manage hundreds of Jupyter notebooks and run SQL queries on millions of rows of data. The solution also needed to ensure the security of Embrace’s customer data, which included risk-based and standards-based security protocols to protect all data.
Case Study
Citi Ventures Invests in DataRobot for Pioneering Automated ML
Citi Ventures, the innovation arm of Citibank, is constantly on the lookout for emerging trends in technology and financial services that can help solve challenges faced by Citi and its clients. Since its inception in 2010, Citi Ventures has invested in over 100 different companies to enhance Citi’s products and services. However, the organization was seeking innovations that could solve challenges for Citi and its customers more efficiently. They were particularly interested in the field of AI and machine learning, which they saw as game-changing for the financial industry. They were looking for a solution that could empower both data scientists and business users, automating much of the modeling process and freeing up their time to focus on solving complex business problems.
Case Study
At Sanlam, South African Financial Institution, AI Helps Attract, Retain More Customers
Sanlam, Africa’s largest non-banking financial institution, exists with the purpose of empowering generations to be financially secure, prosperous, and confident. However, the company was facing challenges with its data science operations. The open-source AI options they were using felt cumbersome to navigate and lacked critical explainability for business stakeholders and compliance. This was hindering their ability to drive critical business value levers such as sales and client retention. The company needed a more streamlined and transparent AI solution that could help them improve their operations and deliver better results.
Case Study
Freddie Mac Advances Affordable Housing Goals and More than Doubles Analytics Productivity with AI
Freddie Mac, a company chartered by Congress in 1970 to support the U.S. housing finance system, has been facing challenges in achieving meaningful predictions and key insights to inform business decisions. The company works with hundreds of thousands of customers and mines nearly four terabytes of data. However, they found that business intelligence and manual practices didn't scale effectively across this vast customer base and data volume. As market and economic conditions change, Freddie Mac must remain flexible and continuously deliver on its commitment to affordable, adequate housing. In a sea of unstructured and semistructured data, it’s challenging to achieve meaningful predictions and key insights to inform business decisions.
Case Study
French Tech Leader Cegid Generates €15M Additional Volume Annually with AI-Driven Decisions
Cegid, a French tech company offering cloud services and management software solutions, is facing the challenge of creating more models in less time while minimizing the technical skills and resources required. The company serves 350,000 customers across 150 countries and generates €632 in revenue. The predictive analytics team at Cegid is under pressure to meet the ever-expanding demand fueled by frequent acquisitions. The team is tasked with tackling a growing list of business challenges, including predicting the likelihood of getting paid on invoices and the propensity of customers to add services.
Case Study
MAPFRE Accelerates Time to Business Value by 20% with AI
MAPFRE, a Spanish insurance company, operates in over 100 countries, generating €27.3 billion annually. The company's analytics team is responsible for providing advanced analytics to help make decisions on pricing, sales, retention, underwriting, and more. However, given the demand for data insights, the team found it challenging to keep pace with the many incoming requests and deliver value quickly. The team needed to expedite its time to market in tackling new business challenges.
Case Study
AUTOproff Automates More than 50% of Vehicle Estimates – Driving European Expansion
AUTOproff, a European leader in digital dealer-to-dealer trading, was facing a challenge in scaling its operations. The company, which had more than 100,000 cars on auction in 2021, was struggling to produce car value estimates within the 20 minutes promised to customers. This task was entirely dependent on a team of skilled vehicle professionals. As the company grew, the need for scaling became increasingly important. The challenge was to automate the process of producing car value estimates to expedite the turnaround time for customers and free up the data scientists and estimators to focus on more rewarding parts of their jobs.
Case Study
Decode Health Unlocks Better Patient Outcomes with AI
Decode Health, a healthcare AI company, has always relied on predictive analytics to unlock discoveries using data. However, in the early days, modeling was a slow, manual task. Analyzing a single dataset could take two to three weeks, with two to three data team members working around the clock. This exhaustive manual effort included considerable time preparing data, waiting on models, recalibrating, and waiting again. The company needed a solution that could streamline this process and deliver accurate results more quickly and cost-effectively.
Case Study
AI Elevates Patient Care at Phoenix Children’s
Phoenix Children’s is one of the nation’s largest pediatric health systems. It provides world-class inpatient, outpatient, trauma, emergency, and urgent care to children and families for more than 38 years. The organization is continuously at the forefront of innovation and is recognized among the nation’s top-ranked children’s hospitals. Phoenix Children’s wanted to use analytics to improve both clinical and operational decisions. However, manually building a single model took the better part of a year. The healthcare system knew that a certain percentage of children who present with other health concerns may actually have undiagnosed malnutrition. If they could identify cases of malnutrition, they could intervene and influence outcomes.
Case Study
Nigerian Bank Reduces Risk, Cost with ML Driving Decisions
Carbon Digital Bank, a financial institution serving the underserved African market, needed a way to quickly determine credit risk for individuals without prior credit. The bank also wanted to empower its data science team to take on additional business challenges. The bank had committed to a data-first strategy and looked to AI as an integral part of its decision-making. However, assessing customers' credit worthiness was a major challenge. The bank needed to expedite decisions on hundreds of thousands of loan applications every month.
Case Study
Carbon Transforms Consumer Lending with DataRobot
Ngozi Dozie and his brother Chijioke identified a significant gap in the Nigerian financial landscape, particularly in the areas of consumer lending and credit infrastructure. Out of 100 million adults in Nigeria, over 40 million of them did not have bank accounts, and there were only about 200,000 distributed credit cards in the entire country. Commercial banks were hesitant to offer consumer loans due to the high risk associated with lending to consumers without credit. Building a credit score in a market like Nigeria is a huge challenge, with little documented financial history or asset ownership. This presented an opportunity for Carbon, the fintech company started by Ngozi and his brother, to help serve the underbanked population of Nigeria.
Case Study
Trupanion Increases Productivity 10X with DataRobot
Trupanion, a leading provider of medical insurance for cats and dogs, was dealing with a lot of data from different aspects of their business; pricing, sales, claims projection, customer retention, and more. They did a good job of reporting metrics, but they did not yet have the technical capability to analyze that data on a deeper level for optimal decision-making. This required more sophisticated technology and a lot of time. Trupanion was looking for fast and accurate predictive modeling software that is robust enough to support all their different data and information from different functions of their business.
Case Study
Australian Schools Boost Student Success, Reduce Attrition by 13% — with AI
Catholic Education Diocese of Parramatta (CEDP) is an educational institution with 80 schools and 44,500 students across New South Wales. The institution holds a wealth of data on its students, from performance to attendance to demographics. However, CEDP lacked the internal resources to mine this data to improve student performance and advance operational goals. They sought a solution that could help them leverage this data to enhance student success and operations.
Case Study
Pricing Analysis with DataRobot at NTUC Income
NTUC Income, a top composite insurer in Singapore, was facing rising claims costs across the insurance industry. As the cost of doing business increased, the company needed to understand the factors driving up claims costs, who was affected, and what actions to take. Furthermore, with insurance increasingly becoming a commodity, accurate price setting became more critical than ever. However, pricing analysis in insurance can be complex, repetitive, and time-consuming. The traditional method of using Generalized Linear Models (GLMs) for pricing analysis was not ideal due to several limitations. These included assumptions of a straight-line relationship between a rating factor and claim costs, time-consuming processes, and inability to analyze text in claim descriptions. The company needed a solution that could address their pricing analysis challenges and scale with their team.
Case Study
Democratizing Data Science at DemystData
DemystData, a New York-based software company, aims to 'demystify' data by providing a platform that helps clients discover, explore, and access the vast world of data. However, as datasets get larger and data sources more varied, the complexity increases, leading to more time-consuming work for the company's limited pool of data science resources. The company's clients, particularly financial institutions, are underutilizing data, leading to business decisions being made based on suboptimal or incomplete information. DemystData aims to close this gap by increasing their clients' access to new and more data.
Case Study
Steward Health Care Leverages DataRobot’s Automated Machine Learning Platform for Predictive Analytics
Steward Health Care, the largest for-profit private hospital operator in the United States, was faced with the challenge of how to use predictive analytics, artificial intelligence (AI) and machine learning to derive value from the vast amount of data they are required to collect and maintain. The primary task was to improve operational efficiency across Steward’s network of 38 hospitals, with a focus on reducing costs. The company decided to address one of the most pressing challenges facing hospital operations — staffing volume. The typical hospital staffing model is set to average census and volume, leading to inefficiencies during peaks and valleys in patient volume. This results in high expenses for on-call staff and overtime pay. Steward Health Care’s CEO, Dr. Ralph de la Torre, challenged his team to find a more proactive approach.
Case Study
Harmoney and DataRobot Drive Innovation in Australasia’s Personal Loan Market
Harmoney, a marketplace lending platform in Australasia, was facing the challenge of keeping pace with the constant innovation required to stay ahead of big banks. The company's small team of data scientists was tasked with the development and deployment of machine learning models to improve the efficiency of the personal loans market. However, the team was finding it difficult to dedicate sufficient time to predictive analytics due to their other responsibilities. Additionally, the traditional tools they were using for modeling were time-consuming and often led to distractions from the main goal of improving the business.
同类供应商.
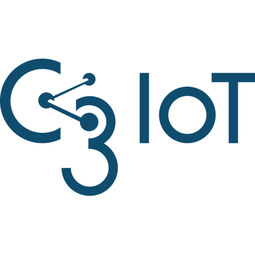
Supplier
C3 IoT
C3 IoT provides a full-stack IoT development platform (PaaS) that enables the rapid design, development, and deployment of even the largest-scale big data / IoT applications that leverage telemetry, elastic Cloud Computing, analytics, and Machine Learning to apply the power of predictive analytics to any business value chain. C3 IoT also provides a family of turn-key SaaS IoT applications including Predictive Maintenance, fraud detection, sensor network health, supply chain optimization, investment planning, and customer engagement. Customers can use pre-built C3 IoT applications, adapt those applications using the platform’s toolset, or build custom applications using C3 IoT’s Platform as a Service.Year founded: 2009
Supplier
Teksun Inc
Teksun Inc provides complete, end-to-end product development services, guiding you through the entire product development process from concept through commercialization, Teksun’s niche expertise of 100% in-house design and development includes IoT, AI/ML, Embedded Hardware, Firmware, Cloud Architecture, & Big Data products.Teksun develops highly innovative products and services which support the ongoing digitization of your enterprise mission.
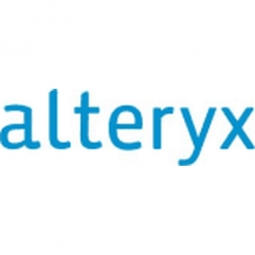
Supplier
Alteryx
Alteryx, Inc. was formed in 2011 and is a leader in self-service Data Science and analytics. Alteryx provides analysts with the unique ability to easily prep, blend and analyze all of their data using a repeatable workflow, then deploy and share analytics at scale for deeper insights in hours, not weeks.Analysts love the Alteryx Analytics platform because they can connect to and cleanse data from data warehouses, cloud applications, spreadsheets and other sources, easily join this data together, then perform analytics – predictive, statistical and spatial – using the same intuitive user interface, without writing any code.
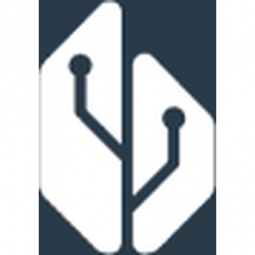
Supplier
InData Labs
InData Labs’ mission is to help clients rocket their competitiveness and get brilliant results of their work unseen before. As a Data Science firm with AI-powered solutions provider with its own R&D center, InData Labs helps businesses of all sizes all over the world to get valuable insights into data, automate repetitive tasks, enhance performance, add AI-driven features, and prevent cost overruns.