Cortical.io
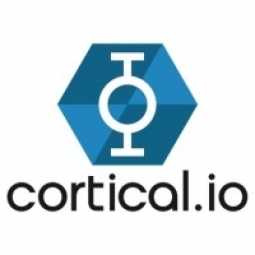
概述
总部
奥地利
|
成立年份
2011
|
公司类型
私营公司
|
收入
< $10m
|
员工人数
11 - 50
|
网站
|
推特句柄
|
公司介绍
Cortical.io delivers highly efficient AI-based solutions that help enterprises unlock the value of unstructured text by leveraging a game-changing approach to Natural Language Understanding (NLU). Cortical.io SemanticPro is an intelligent document processing solution that accurately extracts, analyzes and classifies information based on meaning and builds the basis for document workflow automation.
Supplier missing?
Start adding your own!
Register with your work email and create a new supplier profile for your business.
实例探究.
Case Study
How a large bank saved thousands of hours of manual labor by automating credit agreement analysis
The bank was looking for ways to improve efficiencies through automation, particularly in the area of credit risk assessment. The complexity in language and structure of wholesale credit agreements made automation difficult. These agreements are complex, 100+-page-documents that are very bespoke because banks use different lending systems. Especially covenants use highly specific, use-case related formulations. Some extractions are straightforward, and the values can be extracted “as is” (no inference necessary), for example: named dates, commitments, rates, parties. Others are more challenging and require fine-grained distinctions between options or covenants (eg redeem deal options, termination options). Here an automation system has to comprehend domain logic based on document structure and relations of extracted items. Pricing-related tables contain many different parameters (applicable margin, interest payment schedules, commitment tables) and it is extremely difficult to automate the extraction of pricing information. This is why the review of credit agreements was still done manually, which cost a lot of time and money to the bank, not mentioning the high error rate of such a mundane task.
Case Study
How a Big Four Accounting Firm Reduced Review Time of Lease Agreements by 80%
The company needed to help its customers disclose all leases on the balance sheet due to changes in both international and US regulations. The company’s customers, mostly large enterprises, have thousands of lease agreements, which must be scoured for relevant information. The task was difficult to automate because lease agreements are not standardized and because the same type of information can be expressed using many different terms, causing poor results from keyword-based systems.
Case Study
How a Fortune 100 Technology Manufacturer Reduced Support Engineers’ Search Efforts by 70%
The support cases handled by the company’s support engineers were difficult and time-consuming to resolve because they referred to complicated technical issues in a complex networking environment. The fact that customers often used different terminology than what is used in the company internal documentation made the task even more difficult. Efficient handling of support cases was predicated on finding a solution from past cases instead of troubleshooting the issue from scratch. Attempts to reduce the time to find meaningful results with other search-based solutions failed, as they were not able to quickly and consistently identify similar support cases and did not improve the support team’s productivity.
Case Study
How Cortical.io Semantic Search Helped a Global Company Automate its Marketing Claim Verifications
Ensuring that marketing claims about a product are not misleading or deceptive is a major challenge for this large consumer goods company which must comply with consumer protection laws and industry self-regulatory guidelines. To avoid litigation and the associated costs, marketing claims must be supported by sound factual research results, such as clinical studies and consumer reports. With almost 300,000 new clinical studies registered every year, it is impossible to manually keep track of new evidence related to a specific product. The scientific vocabulary used in such studies differs significantly from the jargon used in marketing claims and the product-relevant scientific information is often not formulated in words, but encapsulated within chemical formulation. This made it impossible to automate the verification process, which remained a long and tedious process involving humanbased tasks of searching, and manually validating evidence documentation.
Case Study
How a Fortune 500 insurance company saved 30% manual labor by automating its quoting workflow
The Fortune 500 insurance company was struggling with the manual extraction of key information from prior insurance plans issued by the competition. The process was tedious, error-prone, and expensive due to labor costs. The company had to review tens of thousands of policies per year, each with their own jargon and format. The complexity of the plans, with multiple entries per row and multiple employee classes of coverage, made the task even more challenging. Manual work often overlooked important provisions, leading to inaccurate quote calculations and profit losses for the company. Conventional tools failed to extract accurate information, forcing the company to rely on expensive manual labor.
Case Study
How a biopharmaceutical company evaluated social media to monitor off-label drug use
The biopharmaceutical company was facing a challenge in tracking off-label usage of their medications. Off-label usage refers to the prescribing of medications in a manner not specified by the FDA and accounts for 10 to 20 percent of all prescriptions written. However, its exact extent is difficult to measure because pharmaceutical companies have no direct feedback mechanism to track what medical conditions their products are prescribed for. The company had no means to monitor how their medications are used as patient records are subject to privacy restrictions and do not allow the company to reconstruct which medication was prescribed for which condition. The company decided to use social media, particularly Reddit, as a source of knowledge about medication usage.
Case Study
How a large US publisher accelerated the RFP process while gaining key market insights
The media company, owning more than 40 publications, was receiving approximately 100 requests per week, corresponding to about 5,000 messages per year and double as many attachments. These Requests for Proposals (RFPs) needed to be classified depending on criteria like vertical market or target audience before being directed to the appropriate department. The challenge was that the RFPs were highly unstructured documents, coming in various formats like email body text or attachments in Word or Powerpoint. The extraction targets were very diverse and, in most cases, with very little context to learn from, making it difficult for state-of-art machine learning systems to deliver satisfactory results.
Case Study
How an International Transportation Company Optimized High Volume Email Processing in Customer Centers
The company was receiving between 100,000 and 250,000 customer emails per day in 35 countries. A major challenge with such a high volume of incoming emails was to identify the emails that actually required a response (only 50%). So far, the customer centers spent a significant amount of time sorting out emails that do not need to be processed (e.g. out of office messages, FYI mails, etc.), a task that unnecessarily burdened the teams and increased the costs in the customer centers. The task was further complicated through the use of multiple languages in the emails.
Case Study
How a major commercial property insurer leveraged SemanticPro to globally standardize policies
The commercial property insurer with offices worldwide has about 2,000 high value, commercial customers with as many as 30 policies each. The head office creates standard policies which are forwarded to the regional offices and adapted locally. As the company does not use industry standard forms, the binding copies of locally-issued documents may differ from the original in format and content. The process of reviewing the locally-issued policies is done manually and is time consuming. The team responsible for this task spends approximately one third of their time on searching for differences between source policy and final version. So far, this review process could not be automated because of the different file types and formats, and because no tool could understand semantic variations. However, the company sought for an automation solution, as about 70% of the documents still contained errors after the manual review.
Case Study
How a Leading Car Manufacturer Automated its Requirement Analysis Process
The leading car manufacturer was dealing with a growing number of requirements (over 150,000) that were described in multiple documents and databases. Thousands of specification sheets existed, each of them containing on average 500 requirements. There was no information about whether a requirement relates to a specific project or to multiple projects, nor an easy way to compare requirements across projects. The requirement engineers had to copy-paste requirements and manually compare them, before using them in new documents. Previous attempts to automate this task had failed, because similar requirements formulated in different ways could not be identified and because requirement texts are often too short to convey a meaning to conventional search engines. The fact that many specification sheets contain complex tables from which requirements must be extracted and that specification sheets do not have a standard structure made it even more difficult for current approaches to solve the problem.